def __getitem__(self, index): # 把numpy转换为Tensor txt=torch.from_numpy(np.array(self.Data[index])) label=torch.tensor(np.array(self.Label[index])) return txt,label出现can't convert np.ndarray of type numpy.str_. The only supported types are: float64, float32, float16, complex64, complex128, int64, int32, int16, int8, uint8, and bool.问题怎么解决
时间: 2024-02-01 21:04:36 浏览: 40
这个问题通常是由于尝试将一个类型为 `numpy.str_` 类型的数组转换为不支持该类型的数组类型,例如 `float64` 或 `int32`。要解决这个问题,可以尝试以下几种方法:
1. 将 `numpy.str_` 类型的数组转换为支持该类型的数组类型。例如,可以使用 `astype()` 方法将数组的类型转换为 `numpy.unicode_` 类型,然后再转换为其他支持的类型。例如:
```
import numpy as np
arr = np.array(['1', '2', '3'], dtype=np.str_)
arr = arr.astype(np.unicode_)
arr = arr.astype(np.int32)
```
2. 检查输入数据的格式和类型。如果输入数据的格式和类型不正确,可以考虑对其进行预处理或转换,以确保其与所使用的函数或模型兼容。
3. 如果使用的是 PyTorch,可以使用 `torch.from_numpy()` 方法将 `numpy.ndarray` 类型数据转换为 `torch.Tensor` 类型数据。例如:
```
import numpy as np
import torch
arr = np.array(['1', '2', '3'], dtype=np.str_)
tensor = torch.from_numpy(arr.astype(np.unicode_).astype(np.int32))
```
希望这些方法能够帮助您解决问题。
相关问题
def getitem(self, index): # 把numpy转换为Tensor txt=torch.from_numpy(np.array(self.Data[index])) label=torch.tensor(np.array(self.Label[index])) return txt,label出现can't convert np.ndarray of type numpy.str_. The only supported types are: float64, float32, float16, complex64, complex128, int64, int32, int16, int8, uint8, and bool.问题应该怎么修改这串代码
这个问题通常是由于尝试将一个类型为 `numpy.str_` 类型的数组转换为不支持该类型的数组类型,例如 `float64` 或 `int32`。要解决这个问题,您可以尝试以下方法:
1. 首先,检查 `self.Data[index]` 和 `self.Label[index]` 的数据类型和格式是否正确。如果是 `numpy.str_` 类型的数组,可以使用 `astype()` 方法将它们转换为其他支持的类型。例如:
```
import numpy as np
data = np.array(['1', '2', '3'], dtype=np.str_)
data = data.astype(np.float32)
label = np.array(['0', '1', '0'], dtype=np.str_)
label = label.astype(np.int32)
```
2. 然后,您可以将 `numpy.ndarray` 类型的数据转换为 `torch.Tensor` 类型的数据。在 PyTorch 中,可以使用 `torch.from_numpy()` 方法将 `numpy.ndarray` 类型数据转换为 `torch.Tensor` 类型数据。例如:
```
import torch
txt = torch.from_numpy(data)
label = torch.from_numpy(label)
```
3. 最后,将 `txt` 和 `label` 作为元组返回。例如:
```
return txt, label
```
希望这些方法能够帮助您解决问题。
请解释以下代码:class LoadDataset(Dataset): def __init__(self, data): self.x = data def __len__(self): return self.x.shape[0] def __getitem__(self, idx): return torch.from_numpy(np.array(self.x[idx])).float(), \ torch.from_numpy(np.array(idx))
该代码定义了一个名为LoadDataset的类,继承自torch中的Dataset类。其中,该类的构造函数__init__接收一个参数data作为输入,并将其赋值给类属性self.x。
类中同时定义了两个方法:__len__和__getitem__。其中,__len__方法返回self.x的第一个维度的长度,即数据集的长度。__getitem__方法通过输入idx,从self.x中取出对应的数据,将其转换为torch.Tensor类型,并返回。返回值是一个包含两个元素的元组,第一个元素是数据,第二个元素是数据的索引。其中,数据被转换为torch.Tensor类型,并使用float()转换为浮点数,索引被转换为numpy数组。
相关推荐
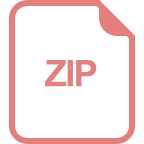
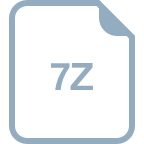
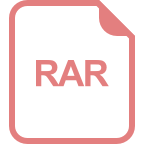












