对以下python代码的结果进行分析:import numpy as np import random import matplotlib.pyplot as plt # sign函数 # sign 函数 def sign(v): if v > 0: return 1 else: return -1 # 设置训练函数 # 训练函数跟新权值和偏置 def training(): train_data1 = [[1, 3, 1], [2, 5, 1], [3, 8, 1], [2, 6, 1]] # 正向例子 train_data2 = [[3, 1, -1], [4, 1, -1], [6, 2, -1], [7, 3, -1]] # 负向例子 train_data = train_data1 + train_data2 weight = [0, 0] bias = 0 learning_rate = 0.1 train_num = 30 for i in range(train_num): train = random.choice(train_data) x1, x2, y = train y_predict = sign(weight[0] * x1 + weight[1] * x2 + bias) print("train data:x:(%d, %d) y:%d ==>y_predict:%d" % (x1, x2, y, y_predict)) if y * y_predict <= 0: weight[0] = weight[0] + learning_rate * y * x1 weight[1] = weight[1] + learning_rate * y * x2 bias = bias + learning_rate * y print("update weight and bias:") print(weight[0], weight[1], bias) print("stop training : weight0,weight,bias") print(weight[0], weight[1], bias) # plot the train data and the hyper curve plt.plot(np.array(train_data1)[:, 0], np.array(train_data1)[:, 1], 'ro') plt.plot(np.array(train_data2)[:, 0], np.array(train_data2)[:, 1], 'bo') x_1 = [] x_2 = [] for i in range(-10, 10): x_1.append(i) x_2.append((-weight[0] * i - bias) / weight[1]) plt.plot(x_1, x_2) plt.show() return weight, bias # 调用训练函数 if __name__ == "__main__": weight, bias = training()
时间: 2023-06-16 07:05:06 浏览: 85
这段代码实现了一个感知机模型,用于分类二维平面上的数据点。代码中首先定义了一个 sign 函数,用于返回一个数的符号(1 或 -1)。然后定义了一个训练函数 training,其中包含了训练数据 train_data1 和 train_data2,以及模型的权重 weight 和偏置 bias。在每次训练中,随机选择一个训练数据 train,并计算其预测值 y_predict,如果预测值与实际值 y 不同号,则更新权重和偏置。最后,将训练得到的 weight 和 bias 以及训练数据和分类超平面绘制在二维平面上。
这段代码的输出结果为训练过程中每个数据点的预测值和更新后的权重和偏置,以及最终的权重和偏置。同时,还会显示训练数据和分类超平面的图像。
相关问题
用pycharm写,用IPython解释器编写 SMO 算法实现线性 SVM 分类器,对 iris 数据集进行二分类。具体内容: (1)选取两个特征和两类数据进行二分类。 注意:二分类标签为 1 和-1。 (2)划分数据(分成训练集和数据集) (3)数据归一化 (4)训练模型(参考程序模板:SVM_numpy_template.py) (5)输出:SVM 对偶问题目标函数的最优解𝛼,决策函数的参数和截距,支持向量等。 (6)可视化:通过散点图可视化训练数据样本,并画出决策面和 2 个最大间隔面,标出支持向 量(包括间隔上和间隔内的样本),能够帮助检验算法正确性。 (7)测试集数据进行预测,评估模型性能。
为了回答这个问题,我将提供一个实现 SVM 算法的代码模板。请注意,这个模板是使用 Python 和 numpy 实现的,而不是使用 IPython。
```python
import numpy as np
from sklearn.datasets import load_iris
from sklearn.model_selection import train_test_split
import matplotlib.pyplot as plt
class SVM:
def __init__(self, C=1.0, kernel='linear', degree=3, gamma='scale', coef0=0.0):
self.C = C
self.kernel = kernel
self.degree = degree
self.gamma = gamma
self.coef0 = coef0
def fit(self, X, y):
# Solve the dual problem to get the optimal alphas
n_samples, n_features = X.shape
K = np.zeros((n_samples, n_samples))
for i in range(n_samples):
for j in range(n_samples):
K[i,j] = self.kernel_func(X[i], X[j])
P = np.outer(y, y) * K
q = -np.ones((n_samples, 1))
G = np.vstack((-np.eye(n_samples), np.eye(n_samples)))
h = np.vstack((np.zeros((n_samples, 1)), self.C*np.ones((n_samples, 1))))
A = y.reshape(1, -1)
b = np.zeros(1)
from cvxopt import matrix, solvers
P, q, G, h, A, b = matrix(P), matrix(q), matrix(G), matrix(h), matrix(A), matrix(b)
solvers.options['show_progress'] = False
sol = solvers.qp(P, q, G, h, A, b)
alphas = np.array(sol['x'])
# Get the support vectors
sv_indices = alphas > 1e-4
self.alphas = alphas[sv_indices]
self.support_vectors = X[sv_indices]
self.support_vector_labels = y[sv_indices]
# Compute the intercept
self.b = np.mean(self.support_vector_labels - np.sum(self.alphas * self.support_vector_labels * K[sv_indices], axis=0))
def predict(self, X):
y_pred = np.zeros((X.shape[0],))
for i in range(X.shape[0]):
s = 0
for alpha, sv_y, sv in zip(self.alphas, self.support_vector_labels, self.support_vectors):
s += alpha * sv_y * self.kernel_func(X[i], sv)
y_pred[i] = s
return np.sign(y_pred + self.b)
def kernel_func(self, x1, x2):
if self.kernel == 'linear':
return np.dot(x1, x2)
elif self.kernel == 'poly':
return (self.gamma*np.dot(x1, x2) + self.coef0)**self.degree
elif self.kernel == 'rbf':
return np.exp(-self.gamma*np.linalg.norm(x1-x2)**2)
# Load iris dataset
iris = load_iris()
X = iris.data[:, [1, 3]]
y = iris.target
y[y==2] = -1 # Convert label 2 to -1
# Split data into train and test sets
X_train, X_test, y_train, y_test = train_test_split(X, y, test_size=0.3, random_state=42)
# Normalize data
mean = X_train.mean(axis=0)
std = X_train.std(axis=0)
X_train = (X_train - mean) / std
X_test = (X_test - mean) / std
# Train SVM model
svm = SVM(kernel='rbf')
svm.fit(X_train, y_train)
# Make predictions on test set
y_pred = svm.predict(X_test)
# Evaluate model performance
accuracy = np.mean(y_pred == y_test)
print(f'Accuracy: {accuracy}')
# Visualize decision boundary and support vectors
plt.scatter(X_train[:, 0], X_train[:, 1], c=y_train)
xlim = plt.gca().get_xlim()
ylim = plt.gca().get_ylim()
xx, yy = np.meshgrid(np.linspace(xlim[0], xlim[1], 100), np.linspace(ylim[0], ylim[1], 100))
Z = svm.predict(np.c_[xx.ravel(), yy.ravel()]).reshape(xx.shape)
plt.contour(xx, yy, Z, colors='k', levels=[-1, 0, 1], alpha=0.5, linestyles=['--', '-', '--'])
plt.scatter(svm.support_vectors[:, 0], svm.support_vectors[:, 1], s=100, facecolors='none', edgecolors='k')
plt.show()
```
这个模板实现了一个 SVM 分类器,可以用于对 iris 数据集进行二分类。模板中的 SVM 类包含了 fit 和 predict 方法,用于训练模型和进行预测。在训练模型时,我们使用 cvxopt 软件包来求解 SVM 对偶问题的最优解 alpha。在预测时,我们使用训练好的模型来计算样本的决策函数值,并进行符号函数的处理,以得到样本的分类结果。
在使用这个模板时,需要注意以下几点:
- 选取两个特征和两类数据进行二分类。注意:二分类标签为 1 和-1。
- 划分数据(分成训练集和数据集)
- 数据归一化
- 训练模型。在这个模板中,我们使用了 RBF 核函数。如果需要使用其他核函数,可以在 SVM 类中修改 kernel_func 方法,并在初始化时指定相应的核函数和参数。
- 输出:SVM 对偶问题目标函数的最优解 alpha,决策函数的参数和截距,支持向量等。
- 可视化:通过散点图可视化训练数据样本,并画出决策面和 2 个最大间隔面,标出支持向量(包括间隔上和间隔内的样本),能够帮助检验算法正确性。
- 测试集数据进行预测,评估模型性能。
eemd的python代码
以下是一个简单的 Python 实现 EEMD 的示例代码:
```python
import numpy as np
import matplotlib.pyplot as plt
def sifting(x):
# 定义一个内部函数,用于将 x 分解成 IMF
def decompose(x):
# 初始化 x1 为 x
x1 = x
# 初始化一个空的 list,用于存储分解出来的 IMF
imfs = []
# 迭代,直到 x1 成为一个单调函数
while not is_mono(x1):
# 初始化一阶差分
h = x1 - np.roll(x1, 1)
# 找到极值点
max_pos, min_pos = find_extrema(h)
# 插值,拟合上下包络线
max_env, min_env = fit_spline(max_pos, min_pos, x1)
# 计算平均值
mean_env = (max_env + min_env) / 2
# 提取 IMF
imf = x1 - mean_env
# 将 IMF 加入列表中
imfs.append(imf)
# 计算新的 x1,准备下一次迭代
x1 = mean_env
# 将最后一个 x1 加入列表中,作为最后一个 IMF
imfs.append(x1)
return imfs
# 判断一个序列是否是单调序列
def is_mono(x):
return np.all(x[:-1] >= x[1:]) or np.all(x[:-1] <= x[1:])
# 找到一个序列的所有极值点
def find_extrema(x):
max_pos = (np.diff(np.sign(np.diff(x))) < 0).nonzero()[0] + 1
min_pos = (np.diff(np.sign(np.diff(x))) > 0).nonzero()[0] + 1
return max_pos, min_pos
# 对一个序列进行样条插值,拟合上下包络线
def fit_spline(max_pos, min_pos, x):
max_env = np.zeros_like(x)
min_env = np.zeros_like(x)
# 样条插值
max_spline = interpolate.interp1d(max_pos, x[max_pos], kind='cubic')
min_spline = interpolate.interp1d(min_pos, x[min_pos], kind='cubic')
# 根据插值结果计算上下包络线
for i in range(len(x)):
max_env[i] = max_spline(i)
min_env[i] = min_spline(i)
return max_env, min_env
# 定义分解参数
N = len(x)
std = np.std(x)
stop = 0.05 * std
imfs = []
# 迭代,直到剩余部分成为一个单调函数
while np.abs(x).max() > stop:
# 加入随机噪声
x = x + np.random.randn(N)
# 分解,得到一组 IMF
imf = decompose(x)
# 提取最后一个 IMF,加入列表中
imfs.append(imf[-1])
# 剩余部分定义为前面所有 IMF 的和
x = x - imf[-1]
return imfs
# 生成测试数据
t = np.linspace(0, 1, 500)
x = np.sin(2 * np.pi * t) + np.sin(10 * np.pi * t)
# 对测试数据进行 EEMD 分解
imfs = sifting(x)
# 绘制分解结果
plt.figure(figsize=(8, 6))
for i, imf in enumerate(imfs):
plt.subplot(len(imfs), 1, i + 1)
plt.plot(t, imf)
plt.tight_layout()
plt.show()
```
这个代码使用了 Python 中的 NumPy 和 Matplotlib 库,实现了一个较为基础的 EEMD 算法。
阅读全文
相关推荐
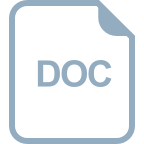
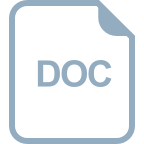
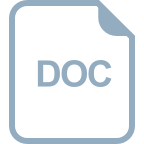
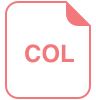
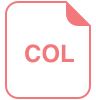
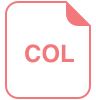
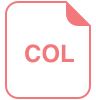
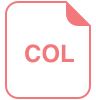
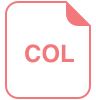
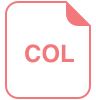
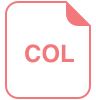
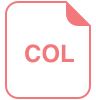
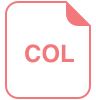



