proj = ccrs.PlateCarree()
时间: 2024-04-08 18:35:21 浏览: 127
这行代码创建了一个名为`proj`的变量,它是使用Cartopy库的`ccrs.PlateCarree()`函数创建的一个投影对象。
`ccrs.PlateCarree()`函数返回一个`PlateCarree`类的实例,表示Plate Carrée投影(也称为等经纬度投影),它是一种简单的经纬度网格投影,将经度和纬度直接映射到平面上。
你可以使用这个`proj`对象来指定地图或图形的投影,例如在绘制地图时使用这个投影来显示经纬度数据。
下面是一个使用这个投影对象创建地图的示例:
```python
import matplotlib.pyplot as plt
import cartopy.crs as ccrs
# 创建一个基于Plate Carrée投影的地图对象
fig = plt.figure()
ax = fig.add_subplot(1, 1, 1, projection=ccrs.PlateCarree())
# 在地图上绘制数据或添加其他地图元素
ax.coastlines()
# 显示地图
plt.show()
```
在上述示例中,我们创建了一个基于Plate Carrée投影的地图对象,并在地图上添加了海岸线。请注意,我们将投影对象作为`projection`参数传递给`add_subplot()`方法,以指定使用该投影对象创建子图。
你可以根据你的具体需求调整代码和使用`proj`对象的方式。如果你需要进一步了解Cartopy库和不同的投影选项,可以查阅Cartopy文档以获取更多信息。
相关问题
ccrs.PlateCarree()的参数有哪些
`ccrs.PlateCarree()`是一个在cartopy库中用于创建平心圆柱投影的对象,它是UTM (Universal Transverse Mercator) 系统的基础。虽然这不是传统的圆柱投影如Mercator,但它通常被用于全球地图,特别是在不需要特别处理极区的情况。`PlateCarree()`函数本身并没有显式传入参数,它是一种预设的标准投影,直接实例化即可使用。它的主要作用在于设置坐标系统的参考体系。
然而,当你使用这个投影作为地理坐标系(Geographic CRS)时,在创建`ccrs.Projection`实例时,你可以关联一些额外的属性,比如添加辅助网格(gridlines)、调整纬度范围等。例如:
```python
import cartopy.crs as ccrs
# 创建一个默认的平心圆柱投影
proj = ccrs.PlateCarree()
# 添加辅助网格
ax = plt.axes(projection=proj)
ax.coastlines(resolution='10m')
ax.gridlines(draw_labels=True)
# 或者调整经度和纬度范围
custom_proj = ccrs.PlateCarree(central_longitude=180) # 以东经180为中心
```
这里提到的是在使用时可能涉及到的一些间接操作,而不是`PlateCarree()`函数本身的参数。如果你想要了解更多关于`ccrs.Projection`及其子类如何自定义的详细信息,可以查阅cartopy的官方文档。
python地图ccrs.PlateCarree()如何跨180度经线连线
在Python的Cartopy库中,`ccrs.PlateCarree()`是一个常用的投影,它代表了地理坐标系统,通常用于处理经纬度数据。当你需要跨越180度经线(国际日期变更线)进行连线时,可能会遇到一些挑战,因为传统的 PlateCarree 投影在180度处会有一个特殊的对齐,称为“反照”(Antimeridian Alignment)。
为了跨过180度经线正确绘制连接线,你需要做的是:
1. **设置正确的中央经线**:如果你的地图范围包含了180度经线,你应该在定义 `PlateCarree()` 时指定中央经线,而不是让它默认为0度。例如,如果你想从西向东画线,可以创建一个 `ccrs.Mercator(central_longitude=180)`。
2. **处理负经度**:对于负经度区域,Cartopy会在180度和-180度之间自动转换,但需要注意的是,这可能导致方向上的错误。因此,在绘制路径时,明确地指定线的方向,比如先从西到东,然后再到东到西。
3. **手动调整终点坐标**:如果线条需要准确地跨越180度经线,你可以在计算终点坐标时,将它们加上360度,以避免负经度带来的影响。
```python
import cartopy.crs as ccrs
# 假设你有两点,分别在175度E和175度W
lon1 = -175
lon2 = 175
# 转换为 PlateCarree 并考虑180度对齐
proj = ccrs.PlateCarree(central_longitude=180)
start_point = (lat1, lon1 + 360) # 注意加360度
end_point = (lat2, lon2)
ax = plt.axes(projection=proj)
ax.plot([start_point[0], end_point[0]], [start_point[1], end_point[1]])
```
阅读全文
相关推荐
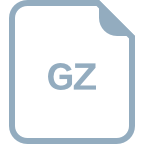

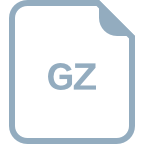













