ValueError: Failed to find data adapter that can handle input: <class 'numpy.ndarray'>, (<class 'list'> containing values of types {"<class 'int'>"})
时间: 2023-07-30 12:08:04 浏览: 184
这个错误通常是因为您正在尝试使用不支持的数据类型作为模型的输入。您可以尝试将输入转换为适当的格式,或者检查模型的输入要求是否正确。
一种可能的解决方案是使用适当的数据适配器将您的输入转换为模型可以处理的格式。您可以尝试使用以下代码将numpy数组转换为Keras模型可以接受的格式:
```
from tensorflow.keras.utils import to_categorical
# 将numpy数组转换为one-hot编码
x = to_categorical(x)
# 将one-hot编码的数据转换为适当的格式
x = x.reshape(x.shape[0], x.shape[1], 1)
```
另外,您也可以检查模型的输入要求是否正确。您可以查看模型的文档或源代码,以确定正确的输入格式。如果您仍然无法解决问题,请提供更多的信息,例如您正在使用的模型和数据格式,并提供完整的错误消息。
相关问题
ValueError: Failed to find data adapter that can handle input: (<class 'list'> containing values of types {"<class 'str'>"}), <class 'numpy.ndarray'>怎么解决
这个错误通常是由于数据类型不匹配导致的。可以尝试将输入数据转换为可接受的类型。
例如,如果您正在使用Keras模型,并且输入数据是一个包含字符串的列表,您可以尝试使用以下代码将其转换为Numpy数组:
``` python
import numpy as np
data = ['hello', 'world']
data_np = np.array(data)
```
如果您仍然遇到问题,请检查您的模型和输入数据的类型是否匹配。您可以尝试将模型的输入层类型更改为与您的数据类型匹配。
怎么解决这个问题:ValueError: Failed to find data adapter that can handle input: <class 'tensorflow.python.framework.ops.EagerTensor'>, <class 'tensorflow.python.ops.resource_variable_ops.ResourceVariable'>
这个错误通常是由于输入的数据类型不匹配导致的。在 TensorFlow 2.x 中,你需要使用 `tf.data.Dataset` 来处理输入数据。你可以将输入数据转换成 `tf.data.Dataset` 格式,然后使用 `fit()` 函数来训练模型。下面是一个示例代码:
```python
import tensorflow as tf
import numpy as np
# 加载数据集
mnist = tf.keras.datasets.mnist
(x_train, y_train), (x_test, y_test) = mnist.load_data()
x_train, x_test = x_train / 255.0, x_test / 255.0
# 将数据转换成 Dataset 格式
train_dataset = tf.data.Dataset.from_tensor_slices((x_train, y_train))
train_dataset = train_dataset.shuffle(10000).batch(32)
# 定义模型
model = tf.keras.models.Sequential([
tf.keras.layers.Flatten(input_shape=(28, 28)),
tf.keras.layers.Dense(128, activation='relu'),
tf.keras.layers.Dropout(0.2),
tf.keras.layers.Dense(10, activation='softmax')
])
# 编译模型
model.compile(optimizer='adam',
loss='sparse_categorical_crossentropy',
metrics=['accuracy'])
# 训练模型
model.fit(train_dataset, epochs=5)
# 生成对抗样本
epsilon = 0.1
x_adv = tf.Variable(x_test[:1000], dtype=tf.float32)
y_true = tf.Variable(y_test[:1000], dtype=tf.int64)
with tf.GradientTape() as tape:
tape.watch(x_adv)
y_pred = model(x_adv)
loss = tf.keras.losses.sparse_categorical_crossentropy(y_true, y_pred)
grad = tape.gradient(loss, x_adv)
normed_grad = tf.sign(grad)
x_adv = x_adv + epsilon * normed_grad
# 评估模型在对抗样本上的性能
test_dataset = tf.data.Dataset.from_tensor_slices((x_adv, y_true))
test_dataset = test_dataset.batch(32)
model.evaluate(test_dataset)
```
在这个示例代码中,我们将输入数据转换成了 `tf.data.Dataset` 格式,并使用 `fit()` 函数来训练模型。在生成对抗样本时,我们同样将数据转换成了 `tf.data.Dataset` 格式,并使用 `evaluate()` 函数来评估模型在对抗样本上的性能。
阅读全文
相关推荐
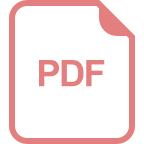
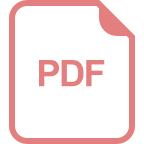
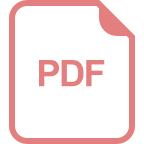








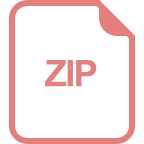