keras多输出模型
时间: 2023-09-03 21:04:34 浏览: 80
Keras是一种用于构建和训练神经网络模型的深度学习框架,它支持多输出模型。在Keras中,我们可以使用函数式API或子类化API来构建多输出模型。
使用函数式API构建多输出模型的方法如下:
1. 导入所需的库和模块。
```python
from tensorflow.keras.models import Model
from tensorflow.keras.layers import Input, Dense
```
2. 定义输入层。
```python
input = Input(shape=(n,))
```
3. 定义中间层和输出层。
```python
hidden1 = Dense(units=64, activation='relu')(input)
hidden2 = Dense(units=32, activation='relu')(hidden1)
output1 = Dense(units=10, activation='softmax')(hidden2)
output2 = Dense(units=1, activation='sigmoid')(hidden2)
```
4. 构建模型。
```python
model = Model(inputs=input, outputs=[output1, output2])
```
5. 编译模型。
```python
model.compile(optimizer='adam', loss=['categorical_crossentropy', 'binary_crossentropy'], metrics=['accuracy'])
```
在这个例子中,我们构建了一个有两个输出的模型,一个输出是10个类别的softmax分类结果,另一个输出是一个二分类结果。注意,在编译模型时,我们需要为每个输出指定相应的损失函数和评估指标。
使用子类化API构建多输出模型的方法相对更灵活,但需要更多的代码编写。我们需要定义一个继承自`tf.keras.models.Model`的子类,并在`call`方法中定义每个输出的计算逻辑。
```python
from tensorflow.keras.models import Model
from tensorflow.keras.layers import Input, Dense
class MultiOutputModel(Model):
def __init__(self):
super(MultiOutputModel, self).__init__()
self.hidden1 = Dense(units=64, activation='relu')
self.hidden2 = Dense(units=32, activation='relu')
self.output1 = Dense(units=10, activation='softmax')
self.output2 = Dense(units=1, activation='sigmoid')
def call(self, inputs):
x = self.hidden1(inputs)
x = self.hidden2(x)
output1 = self.output1(x)
output2 = self.output2(x)
return output1, output2
model = MultiOutputModel()
```
然后,我们可以像使用函数式API一样,编译和训练这个模型。
```python
model.compile(optimizer='adam', loss=['categorical_crossentropy', 'binary_crossentropy'], metrics=['accuracy'])
```
以上是使用Keras构建多输出模型的简单示例。多输出模型在一些任务中很有用,如多标签分类或多任务学习。
相关推荐
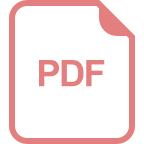














