keras建模输出为类别变量
时间: 2023-06-11 15:07:14 浏览: 59
如果你的输出是类别变量,你可以使用Keras的Sequential模型中的最后一层添加一个Dense层,并使用softmax激活函数来进行多分类的预测。例如:
```
from keras.models import Sequential
from keras.layers import Dense
model = Sequential()
model.add(Dense(64, activation='relu', input_dim=100))
model.add(Dense(10, activation='softmax'))
model.compile(loss='categorical_crossentropy',
optimizer='sgd',
metrics=['accuracy'])
```
其中,最后一层Dense层的输出维度应该等于你的类别数量,激活函数使用softmax,损失函数使用categorical_crossentropy,评估指标使用accuracy。
在训练时,你需要将标签数据进行one-hot编码,例如:
```
from keras.utils import to_categorical
y_train = to_categorical(y_train, num_classes)
y_test = to_categorical(y_test, num_classes)
```
其中,num_classes是你的类别数量。
相关问题
LSTM单变量时间序列
LSTM是一种循环神经网络(RNN)模型,常用于处理和预测时间序列数据。它可以捕捉时间序列中的长期依赖性,并且具有非常好的记忆能力。
在单变量时间序列问题中,我们只考虑一个变量(例如,股票价格、气温等)随时间的变化情况。LSTM模型可以通过输入历史数据,来预测未来的变化趋势。具体来说,LSTM模型会通过多个LSTM单元(包含输入门、遗忘门、输出门等组件)对时间序列数据进行建模,并利用这些单元之间的信息流动来学习时间序列数据中的模式和规律。
一般而言,建立LSTM单变量时间序列模型需要以下步骤:
1. 准备数据:将时间序列数据按照固定时间间隔切割成训练集和测试集。
2. 构建模型:使用Keras或其他深度学习框架构建LSTM模型,设置输入维度、LSTM层数、隐藏单元个数等参数。
3. 模型训练:使用训练集对模型进行训练,并在训练过程中调整超参数以提高模型性能。
4. 模型评估:使用测试集对模型进行评估,计算预测值与实际值之间的误差,并根据误差指标对模型进行选择和调整。
pythonlstm多变量预测
### 回答1:
Python LSTM多变量预测是指使用Python编写LSTM(长短期记忆神经网络)算法来进行多个变量的预测。LSTM算法是一类特殊的神经网络,可以对时间序列数据进行建模,并且能够很好地捕捉到数据之间的长期依赖关系。
在Python中,可以通过使用Tensorflow、Keras、PyTorch等深度学习框架来构建LSTM模型。多变量预测需要输入多个变量,如时间、气温、湿度、气压等。这些变量需要先进行数据预处理,包括缺失值填充、归一化处理、数据平滑等。
在构建多变量LSTM模型时,需要注意的是输入数据应该具有良好的平稳性,即具有稳定的均值和方差,并且需要进行训练和测试集的划分,防止模型过拟合。
预测结果可以通过绘制时间序列图来进行可视化展示,并且可以使用各种性能指标来评估预测准确度,如均方根误差(RMSE)、平均绝对误差(MAE)等。
总之,Python LSTM多变量预测是一种有效地处理时间序列数据并预测多个变量的方法,具有广泛的应用价值。
### 回答2:
Python中的LSTM模型是深度学习在时序预测中的常用方法。它可以用于多个变量的预测。多变量预测模型需要输入和输出的变量保持一致,以便进行有效的训练和预测。
为构建多变量预测模型,需要对数据进行预处理和特征工程。预处理过程包括数据清洗、缺失值处理和变量标准化等步骤。在特征工程中,需要提取有效的特征,例如滞后特征和移动平均特征。提取好的特征可以应用到多变量的LSTM预测模型中。
对于多变量的LSTM预测模型,需要将输入的数据分为多个特征组件和一个输出组件。每个组件都是一个特定的变量或特征。在模型训练中,需要将每个组件独立训练。在预测时,需要将各个组件合并起来来得到最终的预测值。
要正确设置LSTM模型的超参数,并进行自适应优化,以便获得更好的预测结果。如果调整不当,可能会导致模型过拟合或欠拟合,影响预测效果。
总之,多变量预测的LSTM模型是一种强大的工具,可以解决各种时序预测问题。它需要合理的数据预处理、特征提取和模型调整,以获得最佳的预测效果。
相关推荐
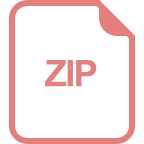
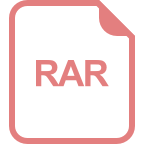
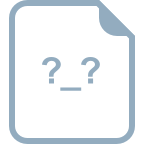









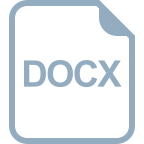
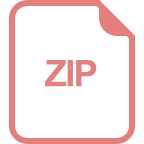
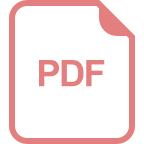
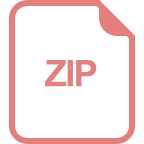