value function和 policy function的区别
时间: 2023-10-30 14:08:18 浏览: 33
Value function和Policy function是强化学习中的两个重要概念。
Value function是指在给定某个状态下,采取某个行动所能获得的长期奖励的期望值,即对于某个状态s来说,其对应的value function V(s)表示在当前状态s下,采取最优策略所能获得的长期奖励的期望值。
Policy function是指在给定某个状态下,选择一个行动的概率分布,即对于某个状态s来说,其对应的policy function π(s)表示在当前状态s下,选择某个行动的概率分布。
因此,Value function和Policy function的区别在于,Value function关注的是在某个状态下采取某个行动所能获得的长期奖励的期望值,而Policy function关注的是在某个状态下选择某个行动的概率分布。在强化学习中,Value function和Policy function通常是相互依存的,即通过Value function来确定最优Policy function,通过Policy function来确定Value function的更新方向。
相关问题
DDPG和A3C的区别
DDPG和A3C是两种用于强化学习的算法,它们在一些方面有所不同。
首先,A3C(Asynchronous Advantage Actor-Critic)算法中有多个agent同时对网络进行异步更新,这样可以减少样本之间的相关性,因此A3C不需要使用Experience Replay机制。这使得A3C支持在线训练模式。而DDPG(Deep Deterministic Policy Gradient)算法则没有这种异步更新的机制。
其次,A3C的策略网络(Policy network)有两个输出。其中一个是softmax输出,用作策略函数(policy function),另一个是线性输出,用作值函数(value function)。而DDPG的网络结构是由一个Actor网络和一个Critic网络组成,Actor网络用于生成动作,Critic网络用于评估状态-动作对的Q值。
此外,A3C中的策略网络评估指标采用的是Advantage Function(A值),而不是DDPG中的Q值。
综上所述,DDPG和A3C在更新方式、网络结构和评估指标等方面存在一些区别。
#### 引用[.reference_title]
- *1* *3* [RL 笔记(2) 从Pollicy Gradient、DDPG到 A3C](https://blog.csdn.net/weixin_43146899/article/details/123241702)[target="_blank" data-report-click={"spm":"1018.2226.3001.9630","extra":{"utm_source":"vip_chatgpt_common_search_pc_result","utm_medium":"distribute.pc_search_result.none-task-cask-2~all~insert_cask~default-1-null.142^v91^insertT0,239^v3^insert_chatgpt"}} ] [.reference_item]
- *2* [【RL 第6章】Actor Critic、DDPG、A3C](https://blog.csdn.net/qq_51542439/article/details/128740612)[target="_blank" data-report-click={"spm":"1018.2226.3001.9630","extra":{"utm_source":"vip_chatgpt_common_search_pc_result","utm_medium":"distribute.pc_search_result.none-task-cask-2~all~insert_cask~default-1-null.142^v91^insertT0,239^v3^insert_chatgpt"}} ] [.reference_item]
[ .reference_list ]
深度强化学习中的policy-based agents
深度强化学习中的policy-based agents是指一类基于策略(policy)的强化学习算法,其主要目标是在不断与环境交互的过程中,学习一个最优的策略来获得最大的累积奖励。相比于值函数(value function)方法,policy-based agents可以直接从策略空间中搜索最优策略,因此通常具有更好的收敛性和泛化能力。
Policy-based agents的核心是策略网络(policy network),它通常是一个深度神经网络,输入是当前状态,输出是在该状态下采取各个动作的概率分布。策略网络可以使用各种深度学习模型,如全连接神经网络、卷积神经网络和循环神经网络等。在训练过程中,策略网络通过最大化累积奖励来优化其参数,通常使用梯度上升算法(如REINFORCE算法)来更新策略。
与值函数方法相比,policy-based agents的优点在于可以处理连续动作空间和高维状态空间,同时也比较容易对策略进行优化。缺点在于学习效率相对较低,需要更多的数据和计算资源。
相关推荐
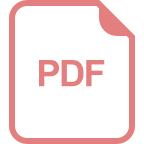
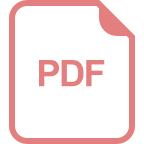
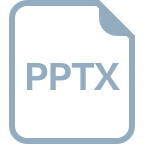













