> complete_data <- na.omit(data) > rdbwselect(y = complete_data$ALL, x = complete_data$ID, all = TRUE) Error in if (mass_l >= 0.2 | mass_r >= 0.2) { : missing value where TRUE/FALSE needed In addition: Warning messages: 1: In min(X_l) : no non-missing arguments to min; returning Inf 2: In max(X_l) : no non-missing arguments to max; returning -Inf
时间: 2023-07-14 21:12:59 浏览: 384
这个错误可能是因为你的数据中有缺失值。在执行`na.omit(data)`时,可能会删除一些行,因此在执行`rdbwselect()`时,会出现缺失值。可以尝试使用`complete.cases()`函数来删除包含缺失值的行,例如:
```
complete_data <- data[complete.cases(data),]
rdbwselect(y = complete_data$ALL, x = complete_data$ID, all = TRUE)
```
如果还是出现错误,可以检查`complete_data$ALL`和`complete_data$ID`是否包含正确的数据类型和格式。
相关问题
> complete_data <- data[complete.cases(data),] > rdbwselect(y = complete_data$ALL, x = complete_data$ID, all = TRUE) Error in if (mass_l >= 0.2 | mass_r >= 0.2) { : missing value where TRUE/FALSE needed In addition: Warning messages: 1: In min(X_l) : no non-missing arguments to min; returning Inf 2: In max(X_l) : no non-missing arguments to max; returning -Inf
这个错误可能是因为数据集中存在缺失值,导致在执行 `complete.cases` 函数时删除了包含缺失值的行,因此在后续的函数中出现了错误。建议检查数据集中是否存在缺失值,如果存在,可以使用其他方法进行处理,如填充缺失值或者删除包含缺失值的列。另外,也可以尝试使用 `na.action` 参数来处理缺失值,例如使用 `na.omit` 函数。
> missing_idx <- which(!complete.cases(data)) > missing_idx [1] 69 > data <- na.omit(data) > c1<-with(data,kruskal(Fquantity,deal1:deal2:deal3,group=TRUE, main="a")) Error in deal1:deal2 : NA/NaN argument In addition: Warning messages: 1: In deal1:deal2 : numerical expression has 84 elements: only the first used 2: In deal1:deal2 : numerical expression has 84 elements: only the first used 3: In data.frame(y, trt) : NAs introduced by coercion 4: In data.frame(y, trt) : NAs introduced by coercion
根据你提供的代码和错误信息,出现了两个问题:
1. `missing_idx [1] 69`:`which(!complete.cases(data))` 返回的是包含缺失值的行的索引号,而这里的结果是69,说明第69行包含缺失值。你需要查看这个行的数据,确定哪些变量包含了缺失值。
2. `deal1:deal2` 参数报错:这个错误和之前的问题类似,可能是因为 `deal1` 和 `deal2` 的数据类型不正确或存在缺失值导致的。你需要检查一下 `deal1` 和 `deal2` 的数据类型,并使用之前提到的方法处理缺失值。
以下是一个可能的解决方案:
```R
# 查找包含缺失值的行的索引号
missing_idx <- which(!complete.cases(data))
# 查看包含缺失值的行的数据
data[missing_idx, ]
# 删除包含缺失值的行
data <- na.omit(data)
# 将 deal1 和 deal2 转换为因子型变量
data$deal1 <- as.factor(data$deal1)
data$deal2 <- as.factor(data$deal2)
# 进行方差分析
result <- with(data, kruskal.test(Fquantity ~ deal1:deal2:deal3))
```
这样处理之后,你应该能够避免这些错误并成功进行方差分析。
阅读全文
相关推荐
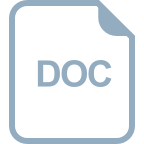
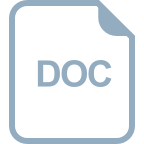
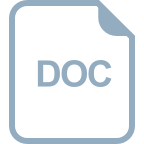



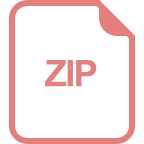
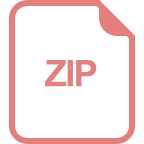
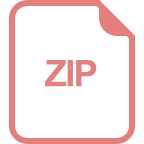
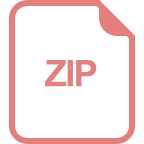
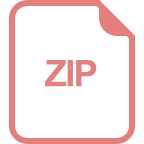
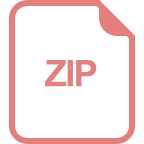
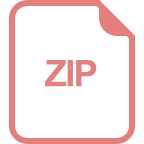
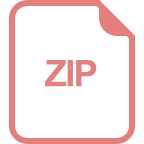


