基于LSTM模型的多头注意力机制的时间序列预测代码
时间: 2023-08-01 17:13:10 浏览: 233
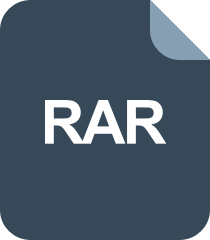
添加TPA注意力机制的LSTM时间序列预测(matlab)

以下是基于LSTM模型的多头注意力机制的时间序列预测代码。需要使用Keras和Tensorflow库。
```python
from keras.layers import Input, Dense, LSTM, concatenate
from keras.models import Model
from keras.callbacks import EarlyStopping
import numpy as np
# 构建输入层
inputs = Input(shape=(timesteps, features))
# 构建LSTM层
lstm = LSTM(units=64, return_sequences=True)(inputs)
# 构建多头注意力机制
attn_weights = []
for _ in range(num_heads):
dense = Dense(units=32, activation='relu')(lstm)
attention = Dense(units=timesteps, activation='softmax')(dense)
attn_weights.append(attention)
attn = concatenate(attn_weights, axis=2)
# 构建加权LSTM层
weighted_lstm = LSTM(units=32, return_sequences=True)(attn)
# 构建输出层
outputs = Dense(units=1)(weighted_lstm)
# 构建模型
model = Model(inputs=inputs, outputs=outputs)
# 编译模型
model.compile(optimizer='adam', loss='mse')
# 定义早期停止回调函数
early_stop = EarlyStopping(monitor='val_loss', patience=5)
# 训练模型
model.fit(x_train, y_train, epochs=100, batch_size=32, validation_split=0.2, callbacks=[early_stop])
# 预测
y_pred = model.predict(x_test)
```
其中,`timesteps`表示时间步长,`features`表示每个时间步长的特征数,`num_heads`表示多头注意力机制的头数,`x_train`和`y_train`表示训练数据,`x_test`表示测试数据。模型的输入为一个形状为`(batch_size, timesteps, features)`的张量,输出为一个形状为`(batch_size, 1)`的张量。在训练模型时,使用早期停止回调函数来避免过拟合。
阅读全文
相关推荐
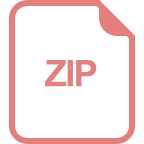
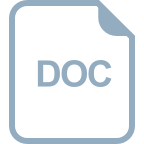
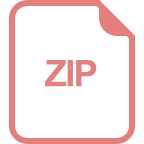
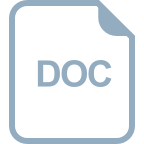
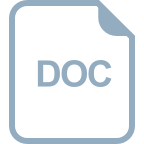
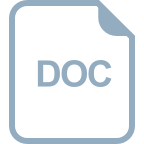
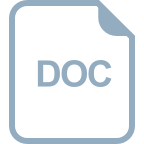
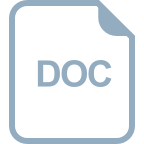
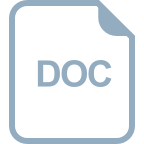
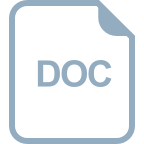
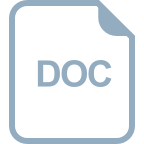





