for n_1 in range(10): for n_2 in range(10): if mode == 'up': # 预测上涨 target = np.where(target > 0, 1, 0) prediction = np.where(pred_up > n_1 / 10, 1, 0) + np.where(pred_dn < n_2 / 10, 1, 0) prediction = np.where(prediction > 1, 1, 0).squeeze() elif mode == 'dn': # 预测下跌 target = np.where(target < 0, 1, 0) prediction = np.where(pred_dn > n_1 / 10, 1, 0) + np.where(pred_up < n_2 / 10, 1, 0) prediction = np.where(prediction > 1, 1, 0).squeeze() # print(list(prediction).count(1)) precision = precision_score(target, prediction, zero_division=0)这段代码有问题吗?可能的问题在哪里?
时间: 2024-02-14 22:21:40 浏览: 112
这段代码是一个分类模型的评估代码,它使用了 precision_score 函数计算模型的精度。可能的问题是:
1. 精度计算可能存在除数为零的情况,因为 zero_division 参数被设置为 0,这意味着如果真实标签中没有正类或负类,则精度将为 0。
2. 在计算分类预测时,使用了 pred_up 和 pred_dn 两个变量,但这些变量在代码中没有给出其来源或定义方式,因此可能存在变量未定义或数据类型错误的问题。
3. 在计算分类预测时,使用了 n_1 和 n_2 变量,并将它们除以 10 来将预测阈值映射到 [0,1] 范围内,但这种处理方式可能存在精度损失或不准确的问题,因为可能会出现一些小数无法精确表示的情况。
4. 在计算分类预测时,使用了 np.where 函数来将预测值转换为二进制分类结果,但这种方式可能存在灵敏度不够的问题,因为可能存在一些预测值分布在阈值附近的情况,导致分类结果不准确。
相关问题
class Dn_datasets(Dataset): def __init__(self, data_root, data_dict, transform, load_all=False, to_gray=False, s_factor=1, repeat_crop=1): self.data_root = data_root self.transform = transform self.load_all = load_all self.to_gray = to_gray self.repeat_crop = repeat_crop if self.load_all is False: self.data_dict = data_dict else: self.data_dict = [] for sample_info in data_dict: sample_data = Image.open('/'.join((self.data_root, sample_info['path']))).copy() if sample_data.mode in ['RGBA']: sample_data = sample_data.convert('RGB') width = sample_info['width'] height = sample_info['height'] sample = { 'data': sample_data, 'width': width, 'height': height } self.data_dict.append(sample) def __len__(self): return len(self.data_dict) def __getitem__(self, idx): sample_info = self.data_dict[idx] if self.load_all is False: sample_data = Image.open('/'.join((self.data_root, sample_info['path']))) if sample_data.mode in ['RGBA']: sample_data = sample_data.convert('RGB') else: sample_data = sample_info['data'] if self.to_gray: sample_data = sample_data.convert('L') # crop (w_start, h_start, w_end, h_end) image = sample_data target = sample_data sample = {'image': image, 'target': target} if self.repeat_crop != 1: image_stacks = [] target_stacks = [] for i in range(self.repeat_crop): sample_patch = self.transform(sample) image_stacks.append(sample_patch['image']) target_stacks.append(sample_patch['target']) return torch.stack(image_stacks), torch.stack(target_stacks) else: sample = self.transform(sample) return sample['image'], sample['target']
这段代码是用来创建一个自定义的 PyTorch 数据集类,名为 Dn_datasets。它的构造函数接受四个参数:data_root,data_dict,transform 和 load_all。其中,data_root 是数据集的根目录,data_dict 是一个字典,包含了数据集中每个样本的路径、宽度和高度等信息,transform 是一个用于数据增强的 torchvision.transforms 实例,load_all 是一个布尔值,指示是否将整个数据集加载到内存中。
在 __init__ 函数中,如果 load_all 是 False,那么 self.data_dict 直接赋值为传入的 data_dict;否则,它会遍历 data_dict 中的每个样本,将其加载到内存中,并将其图像数据、宽度和高度信息封装为一个字典,并将其存储到 self.data_dict 中。
__len__ 函数返回数据集的样本数量,__getitem__ 函数接受一个索引 idx,返回该索引对应的样本。如果 load_all 是 False,那么它会从磁盘上读取该样本的图像数据;否则,它会从 self.data_dict 中读取该样本的图像数据。如果 to_gray 是 True,那么它会将图像转换为灰度图。最后,如果 repeat_crop 大于 1,那么它会对该样本进行多次裁剪,并返回多个图像和目标对作为一个元组;否则,它会对该样本进行单次裁剪,并返回一个图像和目标对作为一个元组。
# Splitting training data into train & dev sets # if mode == 'train': # indices = [i for i in range(len(data)) if i % 10 != 0] # elif mode == 'dev': # indices = [i for i in range(len(data)) if i % 10 == 0] #baseline上面这段代码划分训练集和测试集按照顺序选择数据,可能造成数据分布问题,我改成随机选择 indices_tr, indices_dev = train_test_split([i for i in range(data.shape[0])], test_size = 0.1, random_state = 0) if self.mode == 'train': indices = indices_tr elif self.mode == 'dev': indices = indices_dev # Convert data into PyTorch tensors self.data = torch.FloatTensor(data[indices]) self.target = torch.FloatTensor(target[indices])
这部分代码用于将训练数据划分为训练集和开发集。原来的代码是根据数据的顺序进行划分,可能会导致数据分布不均匀的问题。现在改成了随机选择数据进行划分,使用了train_test_split函数从范围为0到数据长度的列表中随机选择一定比例的索引,其中的10%作为开发集,而剩下的90%作为训练集。
然后,将选择的数据转换为PyTorch的FloatTensor类型,并分别赋值给self.data和self.target。
阅读全文
相关推荐
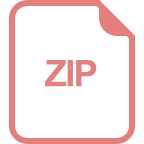
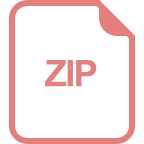













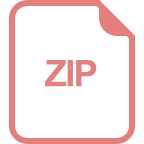