python对多维列表中所有元素进行除法
时间: 2024-02-23 07:00:06 浏览: 28
假设你想对一个二维列表中的所有元素进行除法,可以使用嵌套的 for 循环来遍历列表中的每个元素,并将其除以指定的数值。例如,下面的代码将一个二维列表中的所有元素除以 2:
```python
my_list = [[2, 4, 6], [8, 10, 12], [14, 16, 18]]
for row in my_list:
for i in range(len(row)):
row[i] /= 2
print(my_list)
```
输出结果为:
```
[[1.0, 2.0, 3.0], [4.0, 5.0, 6.0], [7.0, 8.0, 9.0]]
```
如果你想对一个三维列表中的所有元素进行除法,可以使用三层嵌套的 for 循环,以此类推。
相关问题
python中数组算数计算
在Python中,可以使用NumPy库来进行数组的算数计算。NumPy是Python中用于科学计算的一个重要库,它提供了高性能的多维数组对象以及用于处理这些数组的工具。
下面是一个示例,演示了如何在Python中进行数组的算数计算[^1]:
```python
import numpy as np
# 创建两个数组
arr1 = np.array([1, 2, 3])
arr2 = np.array([4, 5, 6])
# 加法
result_add = arr1 + arr2
print("加法结果:", result_add) # 输出:[5 7 9]
# 减法
result_subtract = arr1 - arr2
print("减法结果:", result_subtract) # 输出:[-3 -3 -3]
# 乘法
result_multiply = arr1 * arr2
print("乘法结果:", result_multiply) # 输出:[4 10 18]
# 除法
result_divide = arr1 / arr2
print("除法结果:", result_divide) # 输出:[0.25 0.4 0.5]
```
通过导入NumPy库,我们可以使用其中的数组对象和相应的运算符来进行数组的加法、减法、乘法和除法运算。以上示例中,我们创建了两个数组`arr1`和`arr2`,然后对它们进行了加法、减法、乘法和除法运算,并将结果打印输出。
python中numpy使用
NumPy是Python中用于科学计算的一个常用库。它支持多维数组和矩阵运算,以及各种数学函数的使用。
以下是一些常用的NumPy操作:
1.导入NumPy库
```python
import numpy as np
```
2.创建数组
```python
# 从列表创建一维数组
a = np.array([1, 2, 3])
print(a) # [1 2 3]
# 从列表创建二维数组
b = np.array([[1, 2, 3], [4, 5, 6]])
print(b)
# [[1 2 3]
# [4 5 6]]
# 创建零矩阵
c = np.zeros((2, 3))
print(c)
# [[0. 0. 0.]
# [0. 0. 0.]]
# 创建全矩阵
d = np.ones((2, 3))
print(d)
# [[1. 1. 1.]
# [1. 1. 1.]]
# 创建随机矩阵
e = np.random.rand(2, 3)
print(e)
# [[0.03479189 0.72403896 0.18960562]
# [0.29630598 0.33087097 0.53154379]]
```
3.索引和切片
```python
a = np.array([1, 2, 3, 4, 5])
print(a[0]) # 1
print(a[1:3]) # [2 3]
print(a[::2]) # [1 3 5]
b = np.array([[1, 2, 3], [4, 5, 6]])
print(b[0, 1]) # 2
print(b[:, 1]) # [2 5]
print(b[1, :2]) # [4 5]
```
4.数组运算
```python
a = np.array([1, 2, 3])
b = np.array([4, 5, 6])
# 加法
print(a + b) # [5 7 9]
# 减法
print(a - b) # [-3 -3 -3]
# 乘法
print(a * b) # [ 4 10 18]
# 除法
print(a / b) # [0.25 0.4 0.5 ]
# 矩阵乘法
c = np.array([[1, 2], [3, 4]])
d = np.array([[5, 6], [7, 8]])
print(np.dot(c, d))
# [[19 22]
# [43 50]]
# 数学函数
e = np.array([1, 2, 3])
print(np.exp(e)) # [ 2.71828183 7.3890561 20.08553692]
print(np.sin(e)) # [0.84147098 0.90929743 0.14112001]
```
以上是NumPy的一些基本操作,还有很多其他功能可以在官方文档中找到。
相关推荐
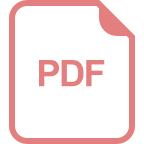












