TF-IDF是特征提取方法吗
时间: 2023-12-30 10:06:36 浏览: 63
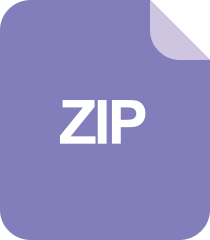
TF-IDF.py.zip_TF-IDF WEIGHT_tf-idf_tf_idf_特征提取
是的,TF-IDF是一种常用的特征提取方法。它可以用于文本分类、信息检索等任务中。TF-IDF中,TF代表词频(Term Frequency),IDF代表逆文档频率(Inverse Document Frequency)。TF用于衡量一个词在文档中出现的频率,而IDF用于衡量一个词的重要性,即在多少文档中出现过。将TF和IDF相乘可以得到一个词的TF-IDF值,这个值可以用于衡量一个词对于某个文档的重要性,从而作为文本特征。
阅读全文
相关推荐
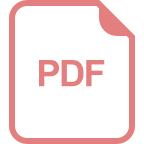
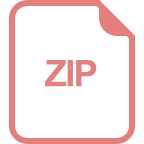
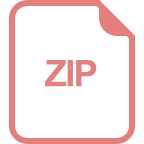
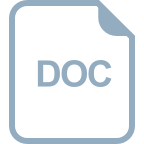
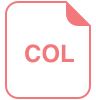
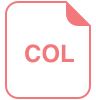









