Dilated neighborhood attention代码
时间: 2024-05-11 10:19:59 浏览: 11
以下是一个Dilated Neighborhood Attention的代码示例:
```python
import torch
import torch.nn as nn
import torch.nn.functional as F
class DilatedNeighborhoodAttention(nn.Module):
def __init__(self, in_channels, reduction_ratio=16, dilation_factors=[1, 2, 4, 8]):
super(DilatedNeighborhoodAttention, self).__init__()
self.in_channels = in_channels
self.reduction_ratio = reduction_ratio
self.dilation_factors = dilation_factors
self.num_factors = len(dilation_factors)
self.conv1 = nn.Conv2d(in_channels=in_channels, out_channels=in_channels//reduction_ratio, kernel_size=1)
self.conv2 = nn.Conv2d(in_channels=in_channels//reduction_ratio, out_channels=self.num_factors, kernel_size=1)
def forward(self, x):
b, c, h, w = x.size()
x = self.conv1(x)
x = F.relu(x)
x = self.conv2(x)
x = F.softmax(x, dim=1)
out = torch.zeros_like(x)
for i in range(self.num_factors):
factor = self.dilation_factors[i]
pad = factor // 2
padded_x = F.pad(x[:, i:i+1, :, :], (pad, pad, pad, pad), mode='constant', value=0)
out[:, i:i+1, :, :] = F.conv2d(padded_x, weight=torch.ones((1, 1, factor, factor)).to(x.device), stride=1, padding=0, dilation=factor)
out = out.view(b, self.num_factors, h*w)
out = out.transpose(1, 2)
out = torch.bmm(out, x.view(b, self.num_factors, h*w))
out = out.transpose(1, 2)
out = out.view(b, h, w, c)
out = out.permute(0, 3, 1, 2)
return out
```
该模块的输入是一个4D张量,形状为(batch_size, in_channels, height, width)。在模块的前半部分,输入通过两个1x1卷积层,首先将通道数降至1/reduction_ratio,然后将其映射到一个大小为num_factors的张量。这个张量被 softmax 规范化,以确保其值总和为1。在模块的后半部分,使用空洞卷积对输入进行多次卷积,每次使用不同的 dilation factor。然后将这些卷积输出的张量与softmax规范化的张量进行加权平均。最后输出的张量形状为(batch_size, in_channels, height, width)。
相关推荐
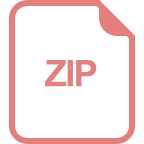
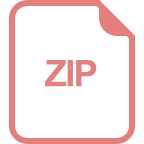
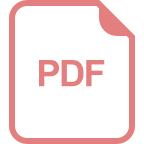














