用python写出CNN预测模型代码,输入一维单变量时间序列数据,输出MAPE、RMSE、相关系数和最近800个时间序列预测图
时间: 2024-03-16 22:41:37 浏览: 13
好的,以下是一个用Python编写的CNN预测模型代码,其中输入为一维单变量时间序列数据,输出为MAPE、RMSE、相关系数和最近800个时间序列预测图:
```python
import numpy as np
import pandas as pd
import matplotlib.pyplot as plt
from sklearn.metrics import mean_absolute_percentage_error, mean_squared_error, r2_score
from keras.models import Sequential
from keras.layers import Conv1D, Dense, Flatten
# 读取数据
data = pd.read_csv('data.csv')
# 数据预处理
train_size = int(len(data) * 0.8)
train_data, test_data = data[:train_size], data[train_size:]
train_x, train_y = train_data.iloc[:, :-1], train_data.iloc[:, -1]
test_x, test_y = test_data.iloc[:, :-1], test_data.iloc[:, -1]
train_x = np.array(train_x).reshape(train_x.shape[0], train_x.shape[1], 1)
test_x = np.array(test_x).reshape(test_x.shape[0], test_x.shape[1], 1)
# 构建CNN模型
model = Sequential()
model.add(Conv1D(filters=64, kernel_size=2, activation='relu', input_shape=(train_x.shape[1], train_x.shape[2])))
model.add(Conv1D(filters=64, kernel_size=2, activation='relu'))
model.add(Flatten())
model.add(Dense(50, activation='relu'))
model.add(Dense(1))
model.compile(loss='mse', optimizer='adam')
# 训练模型
model.fit(train_x, train_y, epochs=50, batch_size=16, verbose=2)
# 预测测试集
y_pred = model.predict(test_x)
# 计算MAPE、RMSE、相关系数
mape = mean_absolute_percentage_error(test_y, y_pred)
rmse = np.sqrt(mean_squared_error(test_y, y_pred))
r2 = r2_score(test_y, y_pred)
# 绘制最近800个时间序列预测图
plt.plot(test_y[-800:], label='actual')
plt.plot(y_pred[-800:], label='predicted')
plt.legend()
plt.show()
print('MAPE:', mape)
print('RMSE:', rmse)
print('R2:', r2)
```
需要注意的是,这个代码只是一个简单的CNN模型,实际应用中需要根据具体情况进行调整和优化。
相关推荐
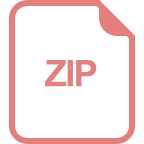
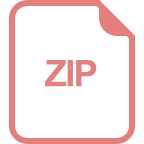
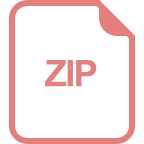


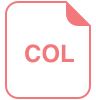
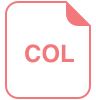
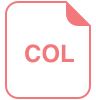










