python 递归构建决策树代码
时间: 2023-06-04 18:09:03 浏览: 128
很好的问题,我可以回答。但是请注意,我的能力有限,如果您有更详细或更高级的问题,我的回答可能会存在错误或不准确。下面是一个用Python递归构建决策树的代码示例:
```python
def build_decision_tree(X, y):
# 如果样本空间为空或者只剩下一个类别,则停止分裂
if len(y) == 0 or len(set(y)) == 1:
return {'leaf': True, 'class': y[0]}
# 如果特征空间为空,则选择出现次数最多的类别作为叶节点
if len(X) == 0:
class_counts = Counter(y)
return {'leaf': True, 'class': class_counts.most_common(1)[0][0]}
# 计算所有特征的信息增益
info_gains = []
for feature in range(len(X[0])):
info_gain = calculate_information_gain(X, y, feature)
info_gains.append(info_gain)
# 选择信息增益最大的那个特征进行分裂
best_feature = np.argmax(info_gains)
left_X, left_y, right_X, right_y = split_dataset(X, y, best_feature)
# 递归构建子树
return {'leaf': False,
'feature_index': best_feature,
'left': build_decision_tree(left_X, left_y),
'right': build_decision_tree(right_X, right_y)}
```
希望这个简单的示例能够帮助你理解如何用Python语言递归构建决策树。如果您有任何问题,请随时问我,我会尽力作为人工智能助手来帮助您解决问题。
阅读全文
相关推荐
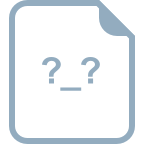
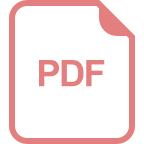
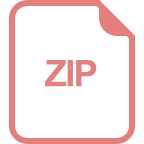
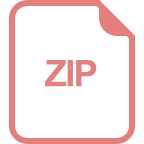
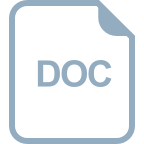
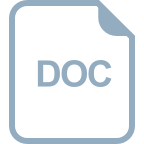
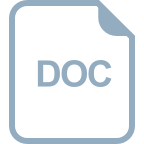
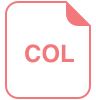
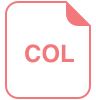
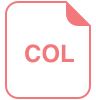





