faster rcnn中RoI Pooling详细过程及代码实现
时间: 2023-10-01 13:04:49 浏览: 108
RoI Pooling是Faster R-CNN中提出的一种特征提取方法,用于从卷积层特征图中提取感兴趣区域(Region of Interest,简称RoI)的特征表示。RoI Pooling的主要目的是将不同大小的RoI映射为固定大小的特征图,从而方便后续的分类和回归任务。
RoI Pooling的过程可以分为以下几个步骤:
1. 对于每个RoI,根据其在原始图像中的坐标信息,在特征图上对应的位置计算出RoI在特征图上的坐标信息。
2. 将RoI在特征图上的坐标信息分别向下取整和向上取整,得到RoI对应的四个顶点在特征图上的坐标信息。
3. 将RoI在特征图上的区域分割为固定大小的子区域,将每个子区域内的特征值进行最大池化操作,得到每个子区域的最大特征值。
4. 将每个子区域的最大特征值作为RoI的特征表示。
下面是RoI Pooling的代码实现:
```python
import torch.nn.functional as F
class RoIPool(nn.Module):
def __init__(self, output_size):
super(RoIPool, self).__init__()
self.output_size = output_size
def forward(self, features, rois):
batch_size, channels, height, width = features.size()
num_rois = rois.size()[0]
output = torch.zeros((num_rois, channels, self.output_size, self.output_size))
for i, roi in enumerate(rois):
# 计算roi在feature map上的位置
x1, y1, x2, y2 = roi.detach().numpy()
roi_width = max(x2 - x1 + 1, 1)
roi_height = max(y2 - y1 + 1, 1)
x_step = roi_width / self.output_size
y_step = roi_height / self.output_size
# 计算每个子区域的位置
for h in range(self.output_size):
for w in range(self.output_size):
x_start = int(x1 + w * x_step)
y_start = int(y1 + h * y_step)
x_end = int(x_start + x_step)
y_end = int(y_start + y_step)
x_start = min(max(x_start, 0), width - 1)
x_end = min(max(x_end, 0), width - 1)
y_start = min(max(y_start, 0), height - 1)
y_end = min(max(y_end, 0), height - 1)
# 对每个子区域进行最大池化操作
sub_feature = features[:, :, y_start:y_end+1, x_start:x_end+1]
output[i, :, h, w] = F.max_pool2d(sub_feature, kernel_size=sub_feature.size()[2:])
return output
```
该实现中,输入的features是特征图,rois是感兴趣区域的坐标信息。输出的是RoI Pooling后的特征表示。具体实现中,我们首先计算每个RoI在特征图上的位置,然后将每个RoI划分为固定大小的子区域,对每个子区域内的特征值进行最大池化操作,得到每个子区域的最大特征值作为RoI的特征表示。
阅读全文
相关推荐











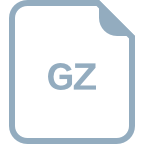






