用MATLAB写Fisher线性判别的代码
时间: 2024-05-20 21:19:37 浏览: 98
下面是MATLAB中Fisher线性判别的代码实现:
% 引入数据
load fisheriris
X = meas(51:end,3:4); % 使用3、4列的数据
y = species(51:end);
% 计算Fisher线性判别
c = length(unique(y)); % 类别数目
n = length(y); % 样本数目
m = mean(X); % 样本均值
Sw = zeros(2,2); % 类内散度矩阵
Sb = zeros(2,2); % 类间散度矩阵
for i = 1:c
Xi = X(y==unique(y(i)),:); % 第i类样本
mi = mean(Xi); % 第i类样本均值
Sw = Sw + (Xi - repmat(mi,size(Xi,1),1))' * (Xi - repmat(mi,size(Xi,1),1)); % 类内散度矩阵
Sb = Sb + size(Xi,1) * (mi - m)' * (mi - m); % 类间散度矩阵
end
[V,D] = eig(Sb,Sw); % 求特征向量和特征值
[lambda,ind] = max(abs(diag(D))); % 找到最大特征值
w = V(:,ind); % 计算Fisher线性判别函数
w = w/norm(w,2); % 归一化
% 可视化结果
figure
gscatter(X(:,1),X(:,2),y,'rgb','osd');
hold on
xmin = min(X(:,1));
xmax = max(X(:,1));
ymin = (w(1)/w(2))*xmin;
ymax = (w(1)/w(2))*xmax;
plot([xmin,xmax],[ymin,ymax],'k-');
legend('setosa','versicolor','virginica','Fisher线性判别函数');
title('Fisher线性判别');
阅读全文
相关推荐
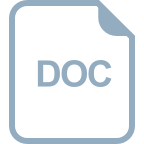
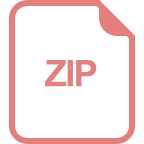
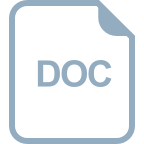
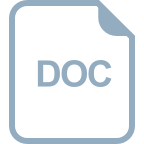
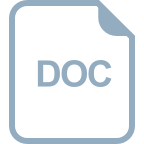
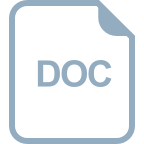
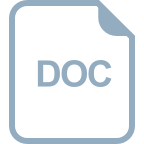
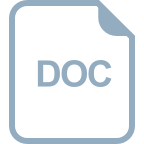



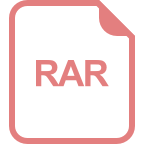


