true_labels = y[unlabeled_indices]什么意思
时间: 2023-06-10 13:07:20 浏览: 81
这行代码假设有一个名为`y`的标签数组和一个名为`unlabeled_indices`的未标记样本的索引数组。该代码行的目的是从`y`数组中提取未标记样本的标签,并将其存储在名为`true_labels`的新数组中。换句话说,它会根据未标记样本的索引,提取对应标签,并将这些标签存储在一个新的数组中,以供后续的操作使用。
相关问题
% Data preparation data = readtable('Attrition_train.csv') n = size(data, 1); train_ratio = 0.8; train_size = floor(n * train_ratio); train_indices = randsample(n, train_size); test_indices = setdiff(1:n, train_indices); train_data = data(train_indices, :); train_labels = data(train_indices, end); test_data = data(test_indices, :); test_labels = data(test_indices, end); % Model training k = 5; model = fitcknn(train_data(:, 1:end-1), train_labels, 'NumNeighbors', k); % Model test predictions = predict(model, test_data(:, 1:end-1)); accuracy = sum(predictions == test_labels) / length(test_labels); disp(['Accuracy: ', num2str(accuracy)]);代码优化
可以考虑将代码封装成函数,这样可以提高代码的可读性和可重用性。另外,可以使用交叉验证来选择最优的k值,可以使用matlab自带的cvpartition函数来进行分割数据集。代码如下:
```
function [accuracy, model] = knn_classification(data, k)
% data: 数据集,包含标签
% k: k值
% 分割数据集
cv = cvpartition(size(data, 1), 'HoldOut', 0.2);
train_data = data(cv.training,:);
train_labels = train_data(:, end);
test_data = data(cv.test,:);
test_labels = test_data(:, end);
% 模型训练
model = fitcknn(train_data(:, 1:end-1), train_labels, 'NumNeighbors', k);
% 模型测试
predictions = predict(model, test_data(:, 1:end-1));
accuracy = sum(predictions == test_labels) / length(test_labels);
disp(['Accuracy: ', num2str(accuracy)]);
end
```
使用方式:
```
data = readtable('Attrition_train.csv');
[accuracy, model] = knn_classification(data, 5);
```
def get_y_preds(y_true, cluster_assignments, n_clusters): """ Computes the predicted labels, where label assignments now correspond to the actual labels in y_true (as estimated by Munkres) cluster_assignments: array of labels, outputted by kmeans y_true: true labels n_clusters: number of clusters in the dataset returns: a tuple containing the accuracy and confusion matrix, in that order """ confusion_matrix = metrics.confusion_matrix(y_true, cluster_assignments, labels=None) # compute accuracy based on optimal 1:1 assignment of clusters to labels cost_matrix = calculate_cost_matrix(confusion_matrix, n_clusters) indices = Munkres().compute(cost_matrix) kmeans_to_true_cluster_labels = get_cluster_labels_from_indices(indices) if np.min(cluster_assignments) != 0: cluster_assignments = cluster_assignments - np.min(cluster_assignments) y_pred = kmeans_to_true_cluster_labels[cluster_assignments] return y_pred
这个函数的作用是根据聚类结果和真实标签计算预测标签,并返回准确率和混淆矩阵。让我来解释一下这个函数的算法流程:
1. 使用 `metrics.confusion_matrix` 函数计算混淆矩阵 `confusion_matrix`。混淆矩阵用于比较聚类结果和真实标签的一致性。
2. 使用 `calculate_cost_matrix` 函数计算成本矩阵 `cost_matrix`。成本矩阵表示将聚类分配给标签的成本。
3. 使用 Munkres 算法通过求解最佳分配问题,得到索引列表 `indices`。这些索引用于将聚类与真实标签进行最佳匹配。
4. 使用 `get_cluster_labels_from_indices` 函数获取从索引列表 `indices` 中提取的聚类标签。
5. 如果聚类结果中的最小值不为0,则将聚类结果减去最小值,以便将聚类结果映射到从0开始的标签。
6. 根据聚类结果映射到真实标签的聚类标签数组 `kmeans_to_true_cluster_labels` 和聚类结果数组 `cluster_assignments`,计算预测标签数组 `y_pred`。
7. 返回预测标签数组 `y_pred`。
函数还计算了准确率和混淆矩阵,但在这段代码中没有明确返回,你可以根据需要进行进一步处理。
请注意,该函数依赖于其他函数 `calculate_cost_matrix` 和 `get_cluster_labels_from_indices` 的实现。你需要确保这些函数已经定义并正确实现。
阅读全文
相关推荐
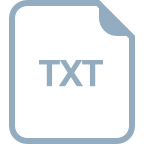
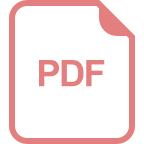
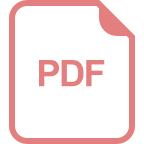













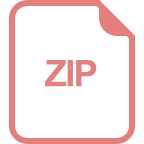
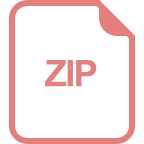