enumerate(data_sets)
时间: 2024-04-30 14:21:24 浏览: 53
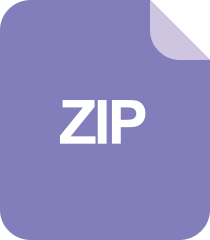
investigate-a-dataset
这是一个Python的内置函数,用于将一个可迭代对象转换成一个枚举对象。在这个函数中,参数data_sets应该是一个可迭代对象,比如一个列表或元组。函数会返回一个迭代器对象,每个元素都是一个元组,包含两个值:序号和对应的数据集元素。示例如下:
```
data_sets = ['train', 'validation', 'test']
for i, data_set in enumerate(data_sets):
print(i, data_set)
```
输出结果为:
```
0 train
1 validation
2 test
```
这里,enumerate()函数将列表data_sets转换成了一个枚举对象,然后在for循环中依次枚举每个元素,并打印其序号和对应的数据集元素。
阅读全文
相关推荐
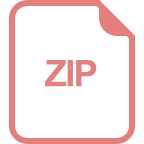

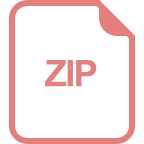
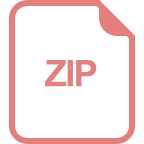
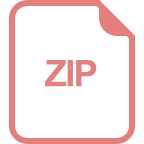
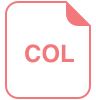
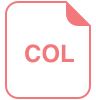
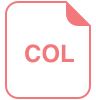



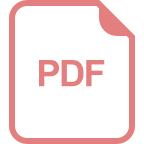
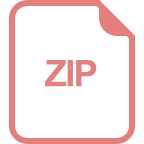
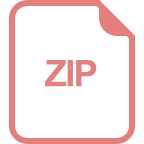