使用R语言搭建卷积神经网络,实现MNIST手写数字数据集识别
时间: 2023-09-17 12:13:57 浏览: 102
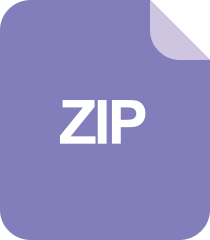
卷积神经网络实现mnist手写识别
好的,以下是使用R语言搭建卷积神经网络,实现MNIST手写数字数据集识别的步骤:
1. 导入所需的包和数据集
```
library(keras)
library(dplyr)
mnist <- dataset_mnist()
x_train <- mnist$train$x
y_train <- mnist$train$y
x_test <- mnist$test$x
y_test <- mnist$test$y
# 将图像像素值缩放到0-1之间
x_train <- x_train / 255
x_test <- x_test / 255
```
2. 将输入数据reshape成适合卷积神经网络的形式
```
x_train <- array_reshape(x_train, c(nrow(x_train), 28, 28, 1))
x_test <- array_reshape(x_test, c(nrow(x_test), 28, 28, 1))
```
3. 构建卷积神经网络模型
```
model <- keras_model_sequential() %>%
layer_conv_2d(filters = 32, kernel_size = c(3, 3), activation = 'relu', input_shape = c(28, 28, 1)) %>%
layer_max_pooling_2d(pool_size = c(2, 2)) %>%
layer_conv_2d(filters = 64, kernel_size = c(3, 3), activation = 'relu') %>%
layer_max_pooling_2d(pool_size = c(2, 2)) %>%
layer_flatten() %>%
layer_dense(units = 128, activation = 'relu') %>%
layer_dropout(rate = 0.5) %>%
layer_dense(units = 10, activation = 'softmax')
```
4. 编译模型
```
model %>% compile(
loss = 'sparse_categorical_crossentropy',
optimizer = optimizer_rmsprop(),
metrics = c('accuracy')
)
```
5. 训练模型
```
model %>% fit(
x_train, y_train,
epochs = 5,
batch_size = 128,
validation_split = 0.2
)
```
6. 在测试集上进行评估
```
model %>% evaluate(x_test, y_test)
```
通过以上步骤,我们可以使用R语言搭建卷积神经网络,实现MNIST手写数字数据集识别。
阅读全文
相关推荐
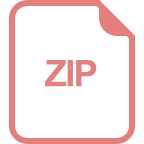
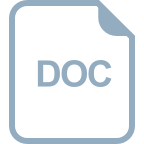



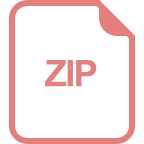
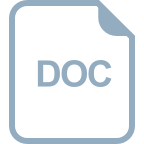


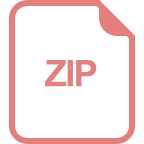
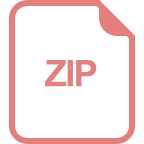
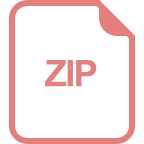
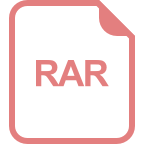
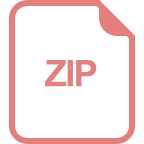
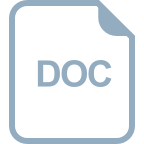
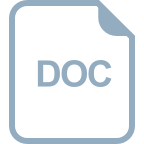