input stem
时间: 2024-01-18 11:03:08 浏览: 260
input stem是指神经网络中的输入层,它通常用于对输入数据进行预处理和特征提取。在ResNet中,input stem是指网络的前几层,用于对输入图像进行卷积和池化操作,以提取图像的低级特征。在不同版本的ResNet中,input stem的设计可能会有所不同,例如可以使用不同的卷积核大小、步长和通道数等。通过修改input stem的设计,可以改变网络的输入特征表示,从而影响网络的性能和适用场景。在ResNet中,可以通过设置input_stem_dict来选择不同版本的input stem,并通过参数version来指定所选版本。
相关问题
虚幻引擎5 配置stem vr
虚幻引擎5 (Unreal Engine 5) 支持VR技术,包括与Stem VR平台的集成。Stem是一款专为教育和娱乐设计的混合现实头戴设备,用户可以在虚拟环境中体验沉浸式的游戏和学习内容。要配置虚幻引擎5以支持Stem VR,你需要做以下步骤:
1. **安装插件**:首先确保在UE5项目中安装了Stem SDK,这通常可以从Stem官网获取,按照官方文档指示安装。
2. **启用VR支持**:在工程设置(Engine > Project Settings)中,进入"Input"类别,然后启用"VR"选项,选择你的Stem VR平台作为输入源。
3. **创建VR场景**:使用UE5的VR工具,如Scene Composer,创建VR专有的交互和布局。
4. **调试和优化**:测试游戏在Stem VR下的运行情况,确保图形性能良好,同时处理好头动追踪、空间定位等VR特定问题。
5. **适配Stem控制器**:将Stem控制器的行为映射到游戏中相应的交互元素上,让玩家能够自然地控制游戏。
6. **兼容性和验证**:确认你的游戏在实际Stem硬件上能顺利运行,并通过Stem提供的开发者工具进行兼容性和性能检查。
使用space to depth代替resnet50的stem中7x7卷积操作
ResNet50中的stem部分包含一个7x7的卷积层,用于对输入图像进行特征提取和降维。可以使用space to depth操作代替这个卷积层。
Space to depth操作将输入张量按照指定的块大小分成若干块,然后将每个块中的元素重排列成一个新的张量。这种操作可以有效地减少特征图的空间大小,从而降低计算成本和内存占用。
具体实现可以参考以下代码:
```python
import tensorflow as tf
def space_to_depth(input_tensor, block_size):
return tf.nn.space_to_depth(input_tensor, block_size)
input_shape = (224, 224, 3)
input_tensor = tf.keras.layers.Input(shape=input_shape)
# 使用space to depth代替7x7卷积
stem = space_to_depth(input_tensor, block_size=7)
# 继续构建ResNet50的后续层
# ...
model = tf.keras.models.Model(inputs=input_tensor, outputs=output_tensor)
```
需要注意的是,使用space to depth代替7x7卷积会导致特征图的通道数变为原来的(block_size x block_size)倍。因此,在后续的网络结构中可能需要做一些调整,例如调整卷积层的输出通道数或者使用更少的卷积层。
阅读全文
相关推荐

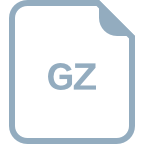














