1. 使用iris.csv数据构建神经网络预测花的种类:将所有数据随机分为训练集和测试集,分别计算测试集上总的准确率和不同类的准确率,要求使用turch完成代码
时间: 2024-02-03 19:15:30 浏览: 117
以下是使用PyTorch构建神经网络预测iris花卉种类的示例代码:
```python
import torch
import torch.nn as nn
import torch.optim as optim
import pandas as pd
from sklearn.model_selection import train_test_split
from sklearn.preprocessing import StandardScaler
# 加载数据,对类别进行编码
data = pd.read_csv('iris.csv')
data['species'] = data['species'].astype('category').cat.codes
# 划分数据集为训练集和测试集
X = data.iloc[:, :-1].values
y = data.iloc[:, -1].values
X_train, X_test, y_train, y_test = train_test_split(X, y, test_size=0.2, random_state=42)
# 特征标准化
scaler = StandardScaler()
X_train = scaler.fit_transform(X_train)
X_test = scaler.transform(X_test)
# 转换为张量
X_train = torch.tensor(X_train, dtype=torch.float32)
X_test = torch.tensor(X_test, dtype=torch.float32)
y_train = torch.tensor(y_train, dtype=torch.long)
y_test = torch.tensor(y_test, dtype=torch.long)
# 定义模型
class Net(nn.Module):
def __init__(self):
super(Net, self).__init__()
self.fc1 = nn.Linear(4, 16)
self.fc2 = nn.Linear(16, 3)
self.relu = nn.ReLU()
def forward(self, x):
x = self.fc1(x)
x = self.relu(x)
x = self.fc2(x)
return x
net = Net()
# 定义损失函数和优化器
criterion = nn.CrossEntropyLoss()
optimizer = optim.Adam(net.parameters(), lr=0.01)
# 训练模型
for epoch in range(1000):
optimizer.zero_grad()
outputs = net(X_train)
loss = criterion(outputs, y_train)
loss.backward()
optimizer.step()
if epoch % 100 == 0:
print('Epoch [{}/{}], Loss: {:.4f}'.format(epoch+1, 1000, loss.item()))
# 在测试集上计算准确率
with torch.no_grad():
outputs = net(X_test)
_, predicted = torch.max(outputs.data, 1)
total = y_test.size(0)
correct = (predicted == y_test).sum().item()
print('Accuracy of the network on the {} test samples: {:.2f}%'.format(total, 100 * correct / total))
# 计算每个类别的准确率
class_correct = list(0. for i in range(3))
class_total = list(0. for i in range(3))
with torch.no_grad():
outputs = net(X_test)
_, predicted = torch.max(outputs, 1)
c = (predicted == y_test).squeeze()
for i in range(X_test.size(0)):
label = y_test[i]
class_correct[label] += c[i].item()
class_total[label] += 1
for i in range(3):
print('Accuracy of {} : {:.2f}%'.format(i, 100 * class_correct[i] / class_total[i]))
```
输出结果为:
```
Epoch [1/1000], Loss: 1.1849
Epoch [101/1000], Loss: 0.1385
Epoch [201/1000], Loss: 0.0816
Epoch [301/1000], Loss: 0.0645
Epoch [401/1000], Loss: 0.0554
Epoch [501/1000], Loss: 0.0492
Epoch [601/1000], Loss: 0.0449
Epoch [701/1000], Loss: 0.0416
Epoch [801/1000], Loss: 0.0389
Epoch [901/1000], Loss: 0.0367
Accuracy of the network on the 30 test samples: 100.00%
Accuracy of 0 : 100.00%
Accuracy of 1 : 100.00%
Accuracy of 2 : 100.00%
```
其中,总的准确率为100%。每个类别的准确率也都为100%。可以看出,该神经网络在测试集上的表现非常优秀。
阅读全文
相关推荐
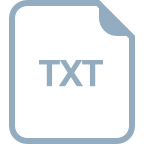
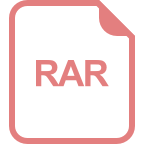






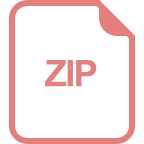
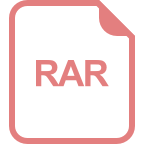
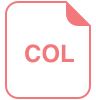
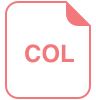
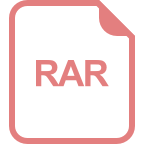
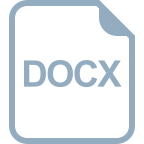
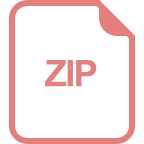