帮我写一个Python代码,完成手写数字识别任务
时间: 2023-08-28 14:22:36 浏览: 93
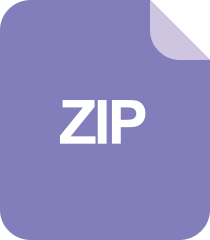
基于Python实现手写数字识别【100012586】

### 回答1:
import numpy as np
from keras.datasets import mnist
from keras.models import Sequential
from keras.layers import Dense
from keras.layers import Dropout
from keras.utils import np_utils# 加载数据
(X_train, y_train), (X_test, y_test) = mnist.load_data()# 设定输入层
num_pixels = X_train.shape[1] * X_train.shape[2]
X_train = X_train.reshape(X_train.shape[0], num_pixels).astype('float32')
X_test = X_test.reshape(X_test.shape[0], num_pixels).astype('float32')# 归一化
X_train = X_train / 255
X_test = X_test / 255# one-hot 编码
y_train = np_utils.to_categorical(y_train)
y_test = np_utils.to_categorical(y_test)
num_classes = y_test.shape[1]# 建立模型
def baseline_model():
# 建立模型
model = Sequential()
model.add(Dense(num_pixels, input_dim=num_pixels, kernel_initializer='normal', activation='relu'))
model.add(Dense(num_classes, kernel_initializer='normal', activation='softmax'))
# 编译模型
model.compile(loss='categorical_crossentropy', optimizer='adam', metrics=['accuracy'])
return model# 建立模型
model = baseline_model()
# 训练模型
model.fit(X_train, y_train, validation_data=(X_test, y_test), epochs=10, batch_size=200, verbose=2)
# 评估模型
scores = model.evaluate(X_test, y_test, verbose=0)
print("Baseline Error: %.2f%%" % (100-scores[1]*100))
### 回答2:
当然可以!以下是一个使用Python和机器学习模型完成手写数字识别任务的示例代码:
```python
import numpy as np
import matplotlib.pyplot as plt
from sklearn.datasets import load_digits
from sklearn.model_selection import train_test_split
from sklearn.neural_network import MLPClassifier
from sklearn.metrics import accuracy_score
# 加载手写数字数据集
digits = load_digits()
# 划分数据集
X_train, X_test, y_train, y_test = train_test_split(digits.data, digits.target, test_size=0.2, random_state=42)
# 创建多层感知器模型
model = MLPClassifier(hidden_layer_sizes=(100,), max_iter=500)
# 在训练集上训练模型
model.fit(X_train, y_train)
# 在测试集上进行预测
y_pred = model.predict(X_test)
# 计算准确率
accuracy = accuracy_score(y_test, y_pred)
print("准确率:", accuracy)
# 随机选择一个测试样本进行显示
random_index = np.random.randint(0, len(X_test))
plt.gray()
plt.matshow(X_test[random_index].reshape(8, 8))
plt.title("真实标签:" + str(y_test[random_index]) + ",预测标签:" + str(y_pred[random_index]))
plt.show()
```
这段代码使用了一个多层感知器模型(MLP)来完成手写数字识别任务。它使用`sklearn`库中的`load_digits()`函数加载了一个手写数字数据集,并将数据集划分为训练集和测试集。然后,它创建了一个包含一个隐藏层的多层感知器模型,并在训练集上训练模型。最后,它使用训练好的模型对测试集进行预测,并计算了预测准确率。
在代码的最后,它还随机选择一个测试样本进行显示,展示了真实标签和预测标签。你可以根据自己的需求进行修改和扩展这段代码,以满足你的个性化需求。
### 回答3:
当然可以帮您写一个Python代码来完成手写数字识别任务。以下是一个简单的示例代码:
```python
import tensorflow as tf
from tensorflow import keras
# 加载MNIST数据集
mnist = keras.datasets.mnist
(train_images, train_labels), (test_images, test_labels) = mnist.load_data()
# 数据预处理
train_images = train_images / 255.0
test_images = test_images / 255.0
# 构建模型
model = keras.Sequential([
keras.layers.Flatten(input_shape=(28, 28)),
keras.layers.Dense(128, activation='relu'),
keras.layers.Dense(10, activation='softmax')
])
# 编译模型
model.compile(optimizer='adam',
loss='sparse_categorical_crossentropy',
metrics=['accuracy'])
# 训练模型
model.fit(train_images, train_labels, epochs=5)
# 评估模型
test_loss, test_acc = model.evaluate(test_images, test_labels)
print('Test accuracy:', test_acc)
# 进行预测
predictions = model.predict(test_images)
```
上述代码使用了TensorFlow库来构建一个简单的全连接神经网络模型,用于手写数字识别任务。首先,我们会加载MNIST数据集,并对图像数据进行预处理。然后,我们构建了一个具有一个输入层、一个隐藏层和一个输出层的神经网络模型。之后,我们编译模型并使用训练数据进行模型训练。训练完成后,我们使用测试数据对模型进行评估,最后进行数字识别的预测。
请注意,这只是一个简单的示例代码,您可以根据自己的需求进行相应的修改和调整。
阅读全文
相关推荐
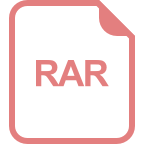
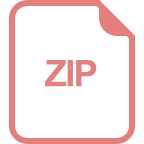
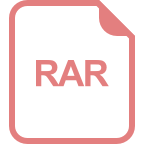
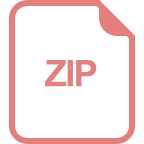
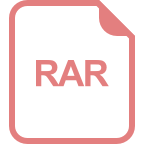
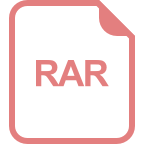
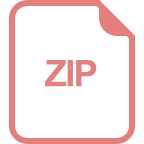
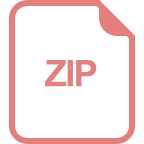
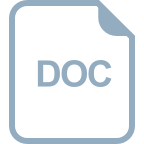







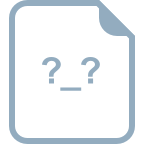