model.predict函数返回值
时间: 2023-04-24 17:07:38 浏览: 128
model.predict函数返回的是模型对输入数据的预测结果。具体来说,它会将输入数据传入模型中,经过一系列计算后得到一个输出结果,这个结果就是模型对输入数据的预测值。在机器学习中,我们通常会将模型的预测结果与真实值进行比较,从而评估模型的性能。
相关问题
model.predict函数返回值的每个类别怎么判断
model.predict函数返回一个数组,其中每个元素表示输入样本属于对应类别的概率。通常情况下,我们会选择概率最大的类别作为模型对输入样本的预测结果。具体地,可以使用numpy.argmax函数来获取概率最大的类别的索引,然后根据索引对应的类别标签来判断预测结果。例如,假设类别标签为[0, 1, 2, 3],则可以使用以下代码来获取预测结果:
```
import numpy as np
# 假设model.predict返回的结果为一个形状为(1, 4)的数组
pred_probs = model.predict(input_data)
pred_label = np.argmax(pred_probs, axis=1)[0]
pred_class = class_labels[pred_label]
print("预测结果为:", pred_class)
```
其中,np.argmax函数的axis参数指定沿哪个轴寻找最大值,这里我们选择第一个轴(即样本维度)来寻找每个样本的最大值。最后根据预测的类别索引找到对应的类别标签即可。
model.predict函数用法
model.predict函数用于对输入数据进行预测,返回预测结果。具体用法如下:
```python
model.predict(x, batch_size=None, verbose=0, steps=None, callbacks=None, max_queue_size=10, workers=1, use_multiprocessing=False)
```
参数说明:
- `x`:需要预测的输入数据,可以是numpy数组或者是tf.data.Dataset对象。
- `batch_size`:整数或者None,预测数据的批次大小。默认为None,表示使用模型的默认批次大小。
- `verbose`:整数,控制预测过程的日志输出。默认为0,表示不输出日志信息。
- `steps`:整数或者None,控制预测数据的批次数。如果不指定,则使用默认值None,表示预测所有数据。
- `callbacks`:keras.callbacks.Callbacks对象的列表,用于在预测过程中使用回调函数。
- `max_queue_size`:整数,控制生成器队列的最大长度。如果使用多进程生成器,该参数将控制每个进程的队列长度。默认为10。
- `workers`:整数,表示使用的进程数。默认为1,表示使用单进程预测。
- `use_multiprocessing`:布尔值,表示是否使用多进程进行预测。默认为False,表示使用单进程预测。
返回值:
返回预测结果,可以是numpy数组或者是Python列表。如果模型有多个输出,返回一个列表,列表中每个元素对应一个输出。如果预测数据是多维的,则返回的预测结果也是多维的。
阅读全文
相关推荐
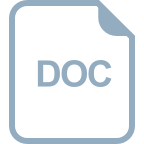
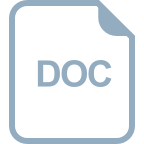
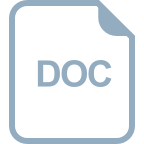
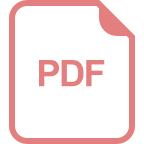




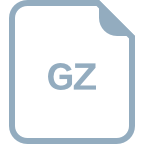
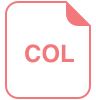
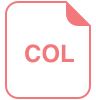





