def gradAscent(dataMatIn, classLabels): dataMatrix=np.mat(dataMatIn) labelMat=np.mat(classLabels).transpose() m,n=np.shape(dataMatrix) alpha=0.001 maxcycles=500 weights=np.ones((n,1)) for k in range(maxcycles): h=sigmoid(dataMatrix*weights) error=labelMat-h weights=weights+alpha*dataMatrix.transpose()*error return weights改为梯度下降算法怎么改?
时间: 2024-03-25 08:40:20 浏览: 52
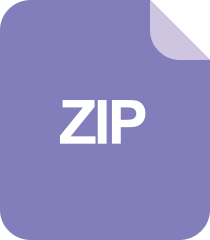
MATLAB实现梯度下降算法(gradient descent),案例丰富【数学建模、科学计算算法】.zip

要将 gradAscent 函数改为梯度下降算法,可以按照以下步骤进行修改:
1. 修改函数名为 gradDescent。
2. 将第 7 行的 weights 初始化为零向量,即 weights=np.zeros((n,1))。
3. 将第 9 行的 h=sigmoid(dataMatrix*weights) 改为 h=dataMatrix*weights,因为在梯度下降中,不需要对 h 进行 sigmoid 计算。
4. 将第 10 行的 error=labelMat-h 改为 error=h-labelMat,因为在梯度下降中,需要求的是损失函数的梯度方向。
5. 将第 11 行的 weights=weights+alpha*dataMatrix.transpose()*error 改为 weights=weights-alpha*dataMatrix.transpose()*error,因为在梯度下降中,是朝着损失函数的梯度方向进行更新权重。
修改后的代码如下:
```python
def gradDescent(dataMatIn, classLabels):
dataMatrix = np.mat(dataMatIn)
labelMat = np.mat(classLabels).transpose()
m, n = np.shape(dataMatrix)
alpha = 0.001
maxCycles = 500
weights = np.zeros((n, 1))
for k in range(maxCycles):
h = dataMatrix * weights
error = h - labelMat
weights = weights - alpha * dataMatrix.transpose() * error
return weights
```
阅读全文
相关推荐
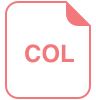
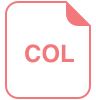
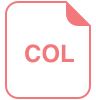
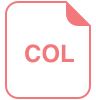



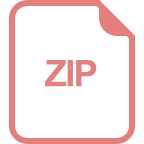
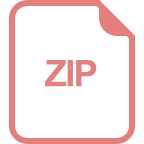
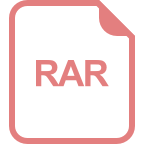
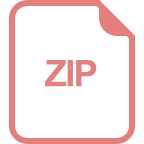
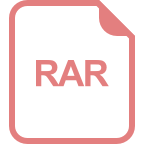
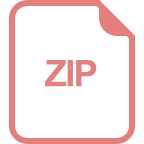