结合scikit-learn库中自带的鸢尾花数据集进行测试 设计随机森林算法实现对鸢尾花数据的分类,并找出最好结果对应参数。 尝试可视化随机森林中的任意3个决策树。
时间: 2024-02-09 13:12:46 浏览: 153
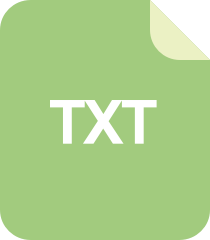
决策树莺尾花-Python scikit-learn库

好的,您的问题是如何结合scikit-learn库中自带的鸢尾花数据集进行测试,设计随机森林算法实现对鸢尾花数据的分类,并找出最好结果对应参数,同时尝试可视化随机森林中的任意3个决策树。
首先,我们需要导入必要的库和数据集:
```python
from sklearn.datasets import load_iris
from sklearn.ensemble import RandomForestClassifier
from sklearn.model_selection import GridSearchCV
from sklearn.tree import export_graphviz
import pydot
iris = load_iris()
X = iris.data
y = iris.target
```
然后,我们可以使用GridSearchCV来寻找最佳参数:
```python
param_grid = {
'n_estimators': [10, 50, 100],
'max_depth': [None, 5, 10],
'max_features': ['auto', 'sqrt', 'log2']
}
rf = RandomForestClassifier(random_state=42)
grid_search = GridSearchCV(estimator=rf, param_grid=param_grid, cv=5, n_jobs=-1)
grid_search.fit(X, y)
best_params = grid_search.best_params_
print(best_params)
```
这里我们使用了n_estimators、max_depth和max_features三个参数来调整随机森林的性能,然后使用GridSearchCV进行参数搜索。最终输出的best_params即为最佳参数组合。
接下来,我们可以使用最佳参数组合来训练随机森林模型:
```python
rf = RandomForestClassifier(random_state=42, **best_params)
rf.fit(X, y)
```
训练完成后,我们可以使用export_graphviz函数和pydot库来可视化随机森林中的任意三个决策树:
```python
estimator = rf.estimators_[0]
export_graphviz(estimator, out_file='tree.dot',
feature_names = iris.feature_names,
class_names = iris.target_names,
rounded = True, proportion = False,
precision = 2, filled = True)
(graph, ) = pydot.graph_from_dot_file('tree.dot')
graph.write_png('tree.png')
```
这里我们选择了随机森林中的第一个决策树,将其输出为.dot文件,然后使用pydot库将其转换为PNG图像文件。
重复以上步骤,我们可以得到三个决策树的可视化结果。
最终的完整代码如下:
```python
from sklearn.datasets import load_iris
from sklearn.ensemble import RandomForestClassifier
from sklearn.model_selection import GridSearchCV
from sklearn.tree import export_graphviz
import pydot
iris = load_iris()
X = iris.data
y = iris.target
param_grid = {
'n_estimators': [10, 50, 100],
'max_depth': [None, 5, 10],
'max_features': ['auto', 'sqrt', 'log2']
}
rf = RandomForestClassifier(random_state=42)
grid_search = GridSearchCV(estimator=rf, param_grid=param_grid, cv=5, n_jobs=-1)
grid_search.fit(X, y)
best_params = grid_search.best_params_
print(best_params)
rf = RandomForestClassifier(random_state=42, **best_params)
rf.fit(X, y)
for i, estimator in enumerate(rf.estimators_[:3]):
export_graphviz(estimator, out_file=f'tree_{i}.dot',
feature_names = iris.feature_names,
class_names = iris.target_names,
rounded = True, proportion = False,
precision = 2, filled = True)
(graph, ) = pydot.graph_from_dot_file(f'tree_{i}.dot')
graph.write_png(f'tree_{i}.png')
```
希望这个回答对您有所帮助!
阅读全文
相关推荐
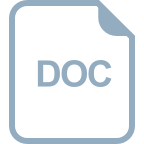
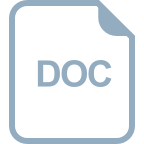















