tarfile.open('CUB_200_2011.tar', 'r:gz')
时间: 2023-08-21 11:07:50 浏览: 156
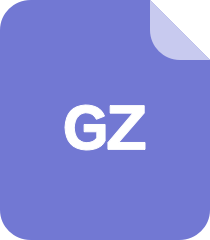
auto_download_IRIS_data.tar.gz
如果你使用 `tarfile.open('CUB_200_2011.tar', 'r:gz')` 打开一个名为 `CUB_200_2011.tar` 的文件,那么在解压缩时会出现错误,因为该文件不是一个正确的 `gzip` 格式的压缩文件。
`tarfile` 模块中的 `open()` 方法支持多种压缩格式,包括 `gzip`、`bzip2`、`xz` 和 `tar` 等格式。但是,要正确地打开和解压缩一个文件,你需要指定正确的压缩格式。
在这个例子中,如果你要打开一个 `gzip` 格式的压缩文件 `CUB_200_2011.tar.gz`,你应该使用 `r:gz` 模式打开它:
```python
import tarfile
# 打开 tar.gz 文件
with tarfile.open('CUB_200_2011.tar.gz', 'r:gz') as tar:
# 解压所有文件到当前目录
tar.extractall()
```
如果你要打开一个 `tar` 格式的文件,你应该使用 `r:` 模式打开它:
```python
import tarfile
# 打开 tar 文件
with tarfile.open('CUB_200_2011.tar', 'r:') as tar:
# 解压所有文件到当前目录
tar.extractall()
```
注意,在解压缩时,你需要使用正确的模式打开文件,以便 `tarfile` 模块可以正确地识别压缩格式并解压缩文件。
阅读全文
相关推荐
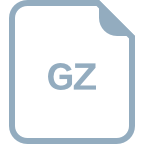
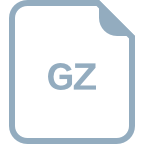








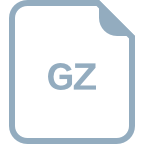
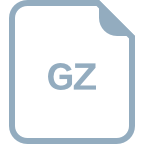
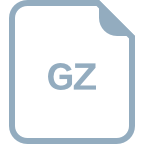
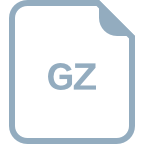
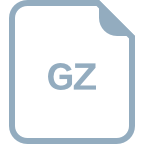
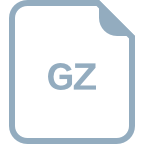
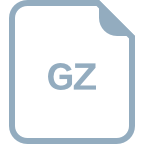
