返回第i行和第j列像素网格中的像素(零开始计数)。 如果没有行i或列j,则返回0。
时间: 2023-12-14 19:38:32 浏览: 33
好的,这里是一个示例函数来实现您所描述的功能:
```python
def get_pixel(i, j, grid):
"""
Get the pixel at row i and column j in the grid.
If there is no row i or column j, return 0.
"""
if i >= len(grid) or j >= len(grid[0]):
return 0
return grid[i][j]
```
这个函数接受三个参数:行数i、列数j和一个二维网格grid。它首先检查i和j是否超出了grid的索引范围。如果是,则返回0。否则,它返回grid中第i行第j列的像素值。请注意,这里的行和列都是从零开始计数的。
相关问题
def get_pixel_at(pixel_grid, i, j): ''' 返回第i行和第j列像素网格中的像素(零开始计数)。 如果没有行i或列j,则返回0。 '''
这是一个 Python 函数,根据给定的像素网格和行列索引返回相应的像素值。如果指定的行或列不存在,则返回 0。
下面是函数的代码实现:
```python
def get_pixel_at(pixel_grid, i, j):
'''
返回第i行和第j列像素网格中的像素(零开始计数)。
如果没有行i或列j,则返回0。
'''
if i < 0 or i >= len(pixel_grid) or j < 0 or j >= len(pixel_grid[0]):
return 0
else:
return pixel_grid[i][j]
```
函数首先检查给定的索引是否超出了像素网格的边界。如果是,则返回 0。否则,函数返回相应的像素值。
输入图片的大小和特征点的像素坐标以及划分的网格大小,返回网格均匀度
这个问题可以通过以下步骤来解决:
1. 将输入图片转换成灰度图像,并使用特征点检测算法(如SIFT,SURF等)来获取特征点的像素坐标。
2. 将图像分成NxM个网格,其中N和M是所需的网格数量。可以使用OpenCV中的cv::Mat::reshape()函数来实现。
3. 对于每个网格,计算其内部像素的标准差,以评估其均匀度。标准差越小,表示像素分布越均匀。
4. 最后,对于所有网格的标准差进行平均,以得到整个图像的网格均匀度。
下面是一个可能的Python代码示例:
```
import cv2
import numpy as np
def calculate_grid_uniformity(image_path, feature_points, grid_size):
# Load image as grayscale
img = cv2.imread(image_path, cv2.IMREAD_GRAYSCALE)
# Reshape image into NxM grid
N = img.shape[0] // grid_size[0]
M = img.shape[1] // grid_size[1]
img_grid = img.reshape(N, grid_size[0], M, grid_size[1]).swapaxes(1,2).reshape(N*M, grid_size[0]*grid_size[1])
# Calculate standard deviation for each grid
stds = []
for i in range(N*M):
stds.append(np.std(img_grid[i]))
# Calculate average standard deviation for all grids
avg_std = sum(stds) / len(stds)
return avg_std
```
其中,`image_path`是输入图像的路径,`feature_points`是特征点的像素坐标,`grid_size`是划分的网格大小(例如,`grid_size=(8, 8)`表示将图像分成$8\times8$个网格)。函数返回整个图像的网格均匀度。
相关推荐
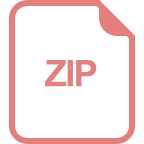
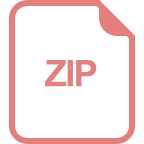
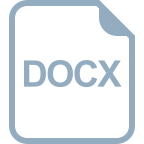












