python opencv Criminisi 算法的具体实现
时间: 2024-02-03 08:14:55 浏览: 49
Criminisi算法是一种图像修复算法,可以用于填补图像中的缺失区域。下面是Python OpenCV实现Criminisi算法的具体步骤:
1.导入必要的库和图像
```python
import cv2
import numpy as np
img = cv2.imread('input.jpg')
mask = cv2.imread('mask.jpg', 0)
```
2.初始化图像和掩码
```python
rows, cols, _ = img.shape
dst = np.zeros_like(img)
# Create a binary mask of the missing area
mask[mask > 0] = 255
```
3.实现Criminisi算法
```python
# Set the size of the search window
w_size = 11
# Initialize the priority queue
p_queue = []
# Loop through each pixel in the mask
for i in range(rows):
for j in range(cols):
if mask[i, j] == 0:
continue
# Get the patch to be filled
patch = img[max(0, i-w_size):min(rows, i+w_size+1),
max(0, j-w_size):min(cols, j+w_size+1)]
# Get the mask for the patch
patch_mask = mask[max(0, i-w_size):min(rows, i+w_size+1),
max(0, j-w_size):min(cols, j+w_size+1)]
# Get the confidence value for each pixel in the patch
confidence = np.zeros(patch.shape[:2])
confidence[patch_mask == 0] = 1
# Get the priority value for each pixel in the patch
priority = np.zeros(patch.shape[:2])
priority[patch_mask == 0] = np.inf
# Add the patch to the priority queue
p_queue.append((i, j, patch, confidence, priority))
```
4.填充缺失区域
```python
# Loop through each pixel in the priority queue
while p_queue:
# Get the pixel with the highest priority value
i, j, patch, confidence, priority = max(p_queue, key=lambda x: np.min(x[4]))
# Get the mask for the patch
patch_mask = mask[max(0, i-w_size):min(rows, i+w_size+1),
max(0, j-w_size):min(cols, j+w_size+1)]
# Compute the fill value for each pixel in the patch
fill = cv2.inpaint(patch, patch_mask, 3, cv2.INPAINT_TELEA)
# Update the destination image and mask
dst[i, j] = fill[patch_mask == 255]
mask[i, j] = 0
# Update the confidence and priority values for each pixel in the patch
confidence[patch_mask == 255] = 1
priority[patch_mask == 255] = np.inf
# Update the priority queue
p_queue.remove((i, j, patch, confidence, priority))
for ii in range(max(0, i-w_size), min(rows, i+w_size+1)):
for jj in range(max(0, j-w_size), min(cols, j+w_size+1)):
if mask[ii, jj] == 0:
continue
# Get the patch to be filled
new_patch = img[max(0, ii-w_size):min(rows, ii+w_size+1),
max(0, jj-w_size):min(cols, jj+w_size+1)]
# Get the mask for the patch
new_patch_mask = mask[max(0, ii-w_size):min(rows, ii+w_size+1),
max(0, jj-w_size):min(cols, jj+w_size+1)]
# Compute the confidence value for each pixel in the patch
new_confidence = np.zeros(new_patch.shape[:2])
new_confidence[new_patch_mask == 0] = 1
# Compute the priority value for each pixel in the patch
new_priority = np.zeros(new_patch.shape[:2])
new_priority[new_patch_mask == 0] = np.inf
# Add the patch to the priority queue
p_queue.append((ii, jj, new_patch, new_confidence, new_priority))
```
5.保存修复后的图像
```python
cv2.imwrite('output.jpg', dst)
```
以上就是Python OpenCV实现Criminisi算法的具体步骤。
相关推荐
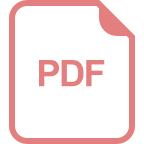














