from skimage.segmentation import slic, mark_boundaries import torchvision.transforms as transforms import numpy as np from PIL import Image import matplotlib.pyplot as plt # 加载图像 image = Image.open('3.jpg') # 转换为 PyTorch 张量 transform = transforms.ToTensor() img_tensor = transform(image).unsqueeze(0) # 将 PyTorch 张量转换为 Numpy 数组 img_np = img_tensor.numpy().transpose(0, 2, 3, 1)[0] # 使用 SLIC 算法生成超像素标记图 segments = slic(img_np, n_segments=60, compactness=10) # 可视化超像素索引映射 plt.imshow(segments, cmap='gray') plt.show() # 将超像素索引映射可视化 segment_img = mark_boundaries(img_np, segments) # 将 Numpy 数组转换为 PIL 图像 segment_img = Image.fromarray((segment_img * 255).astype(np.uint8)) # 保存超像素索引映射可视化 segment_img.save('segment_map.jpg') 将上述代码中引入超像素池化代码:import cv2 import numpy as np # 读取图像 img = cv2.imread('3.jpg') # 定义超像素分割器 num_segments = 60 # 超像素数目 slic = cv2.ximgproc.createSuperpixelSLIC(img, cv2.ximgproc.SLICO, num_segments) # 进行超像素分割 slic.iterate(10) # 获取超像素标签和数量 labels = slic.getLabels() num_label = slic.getNumberOfSuperpixels() # 对每个超像素进行池化操作,这里使用平均值池化 pooled = [] for i in range(num_label): mask = labels == i region = img[mask] pooled.append(region.mean(axis=0)) # 将池化后的特征图可视化 pooled = np.array(pooled, dtype=np.uint8) pooled_features = pooled.reshape(-1) pooled_img = cv2.resize(pooled_features, (img.shape[1], img.shape[0]), interpolation=cv2.INTER_NEAREST) print(pooled_img.shape) cv2.imshow('Pooled Image', pooled_img) cv2.waitKey(0),并显示超像素池化后的特征图
时间: 2024-03-16 20:41:13 浏览: 87
以下是引入超像素池化后的代码,可以显示超像素池化后的特征图:
```
import cv2
import numpy as np
# 读取图像
img = cv2.imread('3.jpg')
# 定义超像素分割器
num_segments = 60
slic = cv2.ximgproc.createSuperpixelSLIC(img, cv2.ximgproc.SLICO, num_segments)
# 进行超像素分割
slic.iterate(10)
# 获取超像素标签和数量
labels = slic.getLabels()
num_label = slic.getNumberOfSuperpixels()
# 对每个超像素进行池化操作,这里使用平均值池化
pooled = []
for i in range(num_label):
mask = labels == i
region = img[mask]
pooled.append(region.mean(axis=0))
# 将池化后的特征图可视化
pooled = np.array(pooled, dtype=np.uint8)
pooled_features = pooled.reshape(-1)
pooled_img = cv2.resize(pooled_features, (img.shape[1], img.shape[0]), interpolation=cv2.INTER_NEAREST)
cv2.imshow('Pooled Image', pooled_img.reshape(img.shape))
cv2.waitKey(0)
cv2.destroyAllWindows()
```
注意,这段代码需要在已经安装 OpenCV 和 NumPy 库的环境中运行。
阅读全文
相关推荐
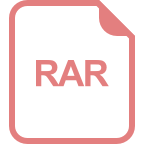
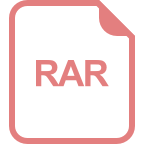



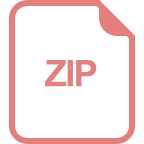
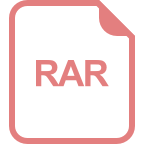
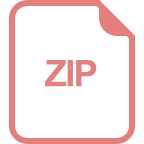
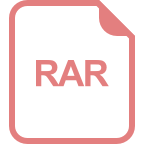
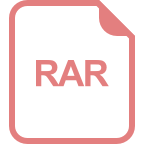
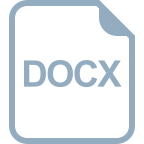
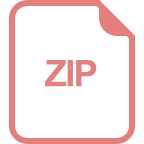
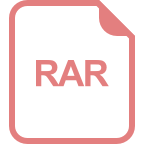
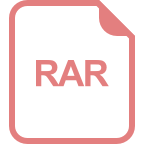
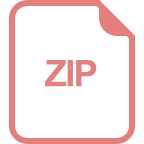