string conditionParam = ContainRelationSetPare.TxtParam.Replace(';', ';'); List<string> arrCondition = conditionParam.Split(';').ToList<string>(); List<string> arrResultA = Source.ReturnValue.Split(';').ToList<string>(); if (ContainRelationSetPare.OptWholeContain) { if (arrCondition.Count != arrResultA.Count) { throw new Exception($"完全包含比对,返回值数量[{arrResultA.Count}]不等于条件值数量[{arrCondition.Count}]"); } } for (int i = 0; i < arrCondition.Count; i++) { if (!arrResultA.Contains(arrCondition[i].Trim())) { throw new Exception($"返回值[{Source.ReturnValue}]不包含条件值{ContainRelationSetPare.TxtParam}"); } }请告诉我这段代码在做什么
时间: 2024-02-10 13:20:41 浏览: 59
这段代码主要是在进行条件判断和异常抛出。具体来说,首先将传入的字符串参数 ContainRelationSetPare.TxtParam 中的中文分号 ';' 转换成英文分号 ';',并将其转换为字符串列表 arrCondition。然后将传入的字符串参数 Source.ReturnValue 也转换成字符串列表 arrResultA。
接下来,如果条件 ContainRelationSetPare.OptWholeContain 为真,则判断 arrCondition 和 arrResultA 的长度是否相等,如果不相等则抛出异常。
最后,对于列表 arrCondition 中的每个元素,判断其是否在列表 arrResultA 中出现,如果没有出现则抛出异常。最终输出的异常信息取决于具体的判断结果。
相关问题
spark = SparkSession.builder.getOrCreate() # 加载Mysql数据 data = spark.read.format("jdbc").options(**options).load() # 将每一行的taglist转为列表 def convert_to_list(line): tmp_list = line[0].replace("#", "").split(",") datas = [] for i in tmp_list: if len(i) > 0 and "牛" not in i: datas.append((i, 1)) return datas rdd = data.rdd.flatMap(lambda line: convert_to_list(line)).reduceByKey(lambda x, y: x + y) schemaString = "tag count" fields = [StructField(field_name, StringType(), True) for field_name in schemaString.split()] schema = StructType(fields) schema_data = spark.createDataFrame(rdd, schema).orderBy("count", ascending=False) # 将数据转换为Pandas数据帧 result_pdf = schema_data.limit(5).toPandas() # 设置matplotlib支持中文 plt.rcParams['font.family'] = ['sans-serif'] plt.rcParams['font.sans-serif'] = ['SimHei'] # colors=color, explode=explode, plt.pie(result_pdf["count"], labels=result_pdf["tag"], shadow=True, autopct='%1.1f%%') plt.legend() plt.show()每行代码讲解
这段代码主要是用来读取MySQL中的数据,并对数据中的taglist进行处理,将每一行的taglist转换为列表,然后统计列表中每个tag的出现次数,最后将结果排序并输出前五个tag及其对应的出现次数。
具体每行代码的含义如下:
1. `spark = SparkSession.builder.getOrCreate()`:创建一个SparkSession实例,如果已经存在则获取已存在的实例。
2. `data = spark.read.format("jdbc").options(**options).load()`:从MySQL中读取数据,并将数据存储为DataFrame格式。
3. `def convert_to_list(line):`:定义一个转换函数,用来将每行的taglist转换为列表。
4. `tmp_list = line[0].replace("#", "").split(",")`:将每行的taglist去掉#号,并按逗号分割,得到一个tag的列表。
5. `datas = []`:定义一个空列表,用来存储tag及其出现次数。
6. `for i in tmp_list:`:遍历每个tag。
7. `if len(i) > 0 and "牛" not in i:`:如果tag长度大于0且不包含“牛”。
8. `datas.append((i, 1))`:将tag及其出现次数1添加到列表datas中。
9. `return datas`:返回列表datas。
10. `rdd = data.rdd.flatMap(lambda line: convert_to_list(line)).reduceByKey(lambda x, y: x + y)`:将DataFrame转换为RDD,并对RDD中的tag进行统计,得到每个tag及其对应的出现次数。
11. `schemaString = "tag count"`:定义一个字符串,用来表示DataFrame的列名。
12. `fields = [StructField(field_name, StringType(), True) for field_name in schemaString.split()]`:根据列名字符串,定义一个包含两个字段(tag和count)的结构体。
13. `schema = StructType(fields)`:根据结构体定义,创建DataFrame的schema。
14. `schema_data = spark.createDataFrame(rdd, schema).orderBy("count", ascending=False)`:基于RDD和schema,创建DataFrame,并按照count字段进行降序排序。
15. `result_pdf = schema_data.limit(5).toPandas()`:将DataFrame转换为Pandas数据帧,并取前五行数据。
16. `plt.rcParams['font.family'] = ['sans-serif']`:设置matplotlib支持中文。
17. `plt.rcParams['font.sans-serif'] = ['SimHei']`:设置matplotlib使用SimHei字体。
18. `plt.pie(result_pdf["count"], labels=result_pdf["tag"], shadow=True, autopct='%1.1f%%')`:绘制饼图,以tag为标签,以count为数据,并显示百分比。
19. `plt.legend()`:添加图例。
20. `plt.show()`:显示图形。
from pyspark.sql import SparkSession import matplotlib.pyplot as plt # 指定Mysql的配置 from pyspark.sql.types import StructField, StringType, StructType options = { "url": "jdbc:mysql://localhost:3306/test?useSSL=true", "driver": "com.mysql.jdbc.Driver", "dbtable": "(SELECT taglist from travels_detail where taglist !='None') t1", "user": "root", "password": "root" } spark = SparkSession.builder.getOrCreate() # 加载Mysql数据 data = spark.read.format("jdbc").options(**options).load() # 将每一行的taglist转为列表 def convert_to_list(line): tmp_list = line[0].replace("#", "").split(",") datas = [] for i in tmp_list: if len(i) > 0 and "牛" not in i: datas.append((i, 1)) return datas rdd = data.rdd.flatMap(lambda line: convert_to_list(line)).reduceByKey(lambda x, y: x + y) schemaString = "tag count" fields = [StructField(field_name, StringType(), True) for field_name in schemaString.split()] schema = StructType(fields) schema_data = spark.createDataFrame(rdd, schema).orderBy("count", ascending=False) # 将数据转换为Pandas数据帧 result_pdf = schema_data.limit(5).toPandas() # 设置matplotlib支持中文 plt.rcParams['font.family'] = ['sans-serif'] plt.rcParams['font.sans-serif'] = ['SimHei'] # colors=color, explode=explode, plt.pie(result_pdf["count"], labels=result_pdf["tag"], shadow=True, autopct='%1.1f%%') plt.legend() plt.show()逐行解释
这段代码是使用 PySpark 从 MySQL 数据库中读取数据,将每一行的 taglist 字段转换为列表,统计列表中每个元素出现的次数,然后将结果转换为 Pandas 数据帧,最后使用 matplotlib 绘制饼图。
这段代码的详细解释如下:
1. 导入需要的模块
```
from pyspark.sql import SparkSession
import matplotlib.pyplot as plt
from pyspark.sql.types import StructField, StringType, StructType
```
这里导入了 `SparkSession` 用于创建 Spark 应用程序,`matplotlib.pyplot` 用于绘制饼图,`StructField`、`StringType`、`StructType` 用于定义数据结构。
2. 配置 MySQL 连接参数
```
options = {
"url": "jdbc:mysql://localhost:3306/test?useSSL=true",
"driver": "com.mysql.jdbc.Driver",
"dbtable": "(SELECT taglist from travels_detail where taglist !='None') t1",
"user": "root",
"password": "root"
}
```
这里定义了连接 MySQL 数据库的参数,包括 URL、驱动程序、表名、用户名和密码。
3. 创建 SparkSession
```
spark = SparkSession.builder.getOrCreate()
```
这里创建了一个 SparkSession 对象,用于连接 Spark 集群。
4. 加载 MySQL 数据
```
data = spark.read.format("jdbc").options(**options).load()
```
这里使用 `SparkSession` 对象的 `read` 方法,读取 MySQL 数据库中的数据。
5. 将每一行的 taglist 转为列表
```
def convert_to_list(line):
tmp_list = line[0].replace("#", "").split(",")
datas = []
for i in tmp_list:
if len(i) > 0 and "牛" not in i:
datas.append((i, 1))
return datas
rdd = data.rdd.flatMap(lambda line: convert_to_list(line)).reduceByKey(lambda x, y: x + y)
```
这里定义了一个函数 `convert_to_list`,用于将每一行的 taglist 字段转换为列表,并且去掉其中的 "#" 和 "牛",统计列表中每个元素出现的次数。
然后使用 `flatMap` 将每一行的 taglist 转换为一个元素为 `(tag, 1)` 的列表,再使用 `reduceByKey` 统计每个 tag 出现的次数。
6. 将数据转换为 Pandas 数据帧
```
schemaString = "tag count"
fields = [StructField(field_name, StringType(), True) for field_name in schemaString.split()]
schema = StructType(fields)
schema_data = spark.createDataFrame(rdd, schema).orderBy("count", ascending=False)
result_pdf = schema_data.limit(5).toPandas()
```
这里先定义了一个字符串 `schemaString`,表示数据结构的字段名,然后使用 `StructField` 和 `StructType` 定义了数据结构。
接着使用 `createDataFrame` 方法将 RDD 转换为 DataFrame,并按照 `count` 字段降序排序,最后使用 `limit` 和 `toPandas` 方法将结果转换为 Pandas 数据帧。
7. 绘制饼图
```
plt.rcParams['font.family'] = ['sans-serif']
plt.rcParams['font.sans-serif'] = ['SimHei']
plt.pie(result_pdf["count"], labels=result_pdf["tag"], shadow=True, autopct='%1.1f%%')
plt.legend()
plt.show()
```
这里设置 matplotlib 支持中文,然后使用 `pie` 方法绘制饼图,其中 `result_pdf["count"]` 和 `result_pdf["tag"]` 分别表示数据帧中的 `count` 和 `tag` 列。最后使用 `legend` 和 `show` 方法显示图表。
阅读全文
相关推荐
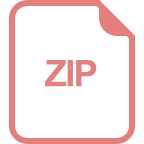
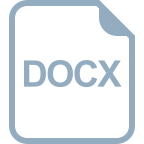
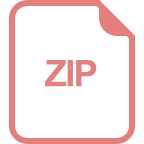
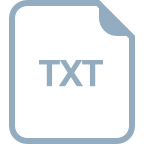
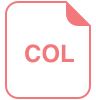
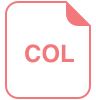
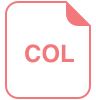
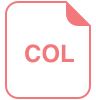
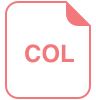
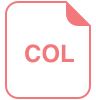
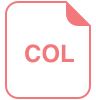
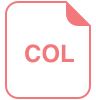
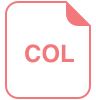
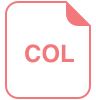


