shape based matching
时间: 2023-04-30 16:04:06 浏览: 66
形状匹配算法,是一种基于形状特征的图像匹配方法,主要通过对图像的形状特征进行提取,计算两个对象之间形状相似度,进而实现图像匹配的目的。常见的形状特征包括边缘、轮廓、角点等。形状匹配算法常被应用于物体识别、机器视觉等领域。
相关问题
fastest_image_pattern_matching shape_based_matching
最快的图像模式匹配算法可能就是基于形状的匹配(shape-based matching)了。这种算法的基本思路是先用特征提取算法将图像中的物体特征提取出来,比如轮廓、边缘、角点等等,然后求出各个特征之间的相对位置关系和大小关系,从而得到该物体的特征描述子。接着,对于任意一张原始图像,也可以用相同的特征提取算法得到它的物体特征描述子,然后就可以通过比较两个物体特征描述子的相似程度,来判断它们是否匹配。
相比于基于像素的匹配算法,基于形状的匹配算法具有更强的鲁棒性和适用性,因为它能够有效地处理物体旋转、缩放、变形、遮挡以及光照变化等问题。而且在对大规模图像数据库进行查询时,基于形状的匹配算法也能够达到较高的检索精度和速度。
不过,需要注意的是,基于形状的匹配算法也存在一些问题,比如可能会出现误匹配和漏匹配的情况,因为不同物体可能具有相似的形状特征。此外,基于形状的匹配算法的运算复杂度也相对较高,需要消耗较多的计算资源。
opencv find_shape_model
The `find_shape_model` function in OpenCV is used for finding a shape model from a set of training images. It is part of the Shape Matching module in OpenCV, which provides tools for matching shapes based on their contour and other features.
The `find_shape_model` function takes as input a set of training images, which are typically binary images with the object of interest represented as a white shape on a black background. The function then computes a shape model based on the contours of the objects in the training images.
The shape model can be used for shape matching, which involves finding the best match between a given image and the shape model. This can be useful for object recognition and tracking.
To use the `find_shape_model` function, you first need to create a ShapeContextDistanceExtractor object, which defines the distance metric used for shape matching. You then call the `find_shape_model` function, passing in the training images and the ShapeContextDistanceExtractor object.
Here's an example of how to use the `find_shape_model` function:
```
import cv2
# Load training images
img1 = cv2.imread("train1.png", cv2.IMREAD_GRAYSCALE)
img2 = cv2.imread("train2.png", cv2.IMREAD_GRAYSCALE)
img3 = cv2.imread("train3.png", cv2.IMREAD_GRAYSCALE)
# Create ShapeContextDistanceExtractor object
sc_extractor = cv2.createShapeContextDistanceExtractor()
# Find shape model from training images
shape_model = cv2.findShapeModel([img1, img2, img3], sc_extractor)
```
In this example, we load three training images and create a ShapeContextDistanceExtractor object. We then call the `findShapeModel` function, passing in the three training images and the ShapeContextDistanceExtractor object. The function returns a shape model, which can be used for shape matching.
阅读全文
相关推荐
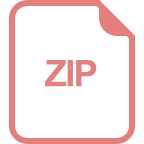
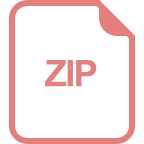
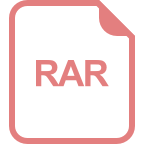













