利用Distiller进行知识蒸馏,给我一个可运行的python代码
时间: 2023-04-05 14:01:04 浏览: 55
以下是一个简单的利用Distiller进行知识蒸馏的Python代码示例:
```python
import torch
import distiller
# 定义原始模型
class OriginalModel(torch.nn.Module):
def __init__(self):
super(OriginalModel, self).__init__()
self.conv1 = torch.nn.Conv2d(3, 64, kernel_size=3, stride=1, padding=1)
self.relu1 = torch.nn.ReLU(inplace=True)
self.conv2 = torch.nn.Conv2d(64, 64, kernel_size=3, stride=1, padding=1)
self.relu2 = torch.nn.ReLU(inplace=True)
self.fc = torch.nn.Linear(64*32*32, 10)
def forward(self, x):
x = self.conv1(x)
x = self.relu1(x)
x = self.conv2(x)
x = self.relu2(x)
x = x.view(-1, 64*32*32)
x = self.fc(x)
return x
# 定义蒸馏模型
class DistilledModel(torch.nn.Module):
def __init__(self, teacher_model):
super(DistilledModel, self).__init__()
self.teacher_model = teacher_model
self.conv1 = torch.nn.Conv2d(3, 32, kernel_size=3, stride=1, padding=1)
self.relu1 = torch.nn.ReLU(inplace=True)
self.conv2 = torch.nn.Conv2d(32, 32, kernel_size=3, stride=1, padding=1)
self.relu2 = torch.nn.ReLU(inplace=True)
self.fc = torch.nn.Linear(32*32*32, 10)
def forward(self, x):
with torch.no_grad():
teacher_output = self.teacher_model(x)
x = self.conv1(x)
x = self.relu1(x)
x = self.conv2(x)
x = self.relu2(x)
x = x.view(-1, 32*32*32)
x = self.fc(x)
return x, teacher_output
# 加载原始模型和数据
original_model = OriginalModel()
original_model.eval()
train_loader, val_loader = distiller.data.load_data(
"cifar10", "/path/to/data", 128, 4)
# 定义蒸馏器
distiller = distiller.Distiller(
train_loader, val_loader, original_model, DistilledModel,
"cifar10", "/path/to/checkpoints", 0.1, 0.1, 0.5, 0.5, 0.5)
# 开始蒸馏
distiller.run()
```
以上代码示例中,我们定义了一个原始模型和一个蒸馏模型,并使用Distiller进行知识蒸馏。在蒸馏模型中,我们使用了原始模型的输出作为教师模型的输出,并在训练过程中使用了蒸馏损失和原始模型的损失来进行训练。
相关推荐
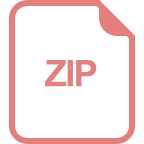
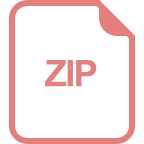
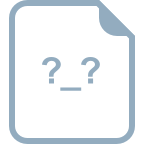














