PetalLength=iris_data["Petal.Length"].astype(float)
时间: 2024-06-04 08:12:22 浏览: 56
This line of code converts the "Petal.Length" column in the "iris_data" DataFrame to a float data type and assigns it to a new variable called "PetalLength". This can be useful for performing numerical operations on the column or for plotting the data using certain libraries that require numeric inputs.
相关问题
PetalLength=iris_data["Petal.Length"].astype(float) TypeError: list indices must be integers or slices, not str
This error occurs when trying to access a list using a string as an index. In this case, it seems like "iris_data" is a list and you are trying to access the "Petal.Length" column using a string index. To fix this, you can convert the list to a pandas dataframe and then access the column using the string as a column name:
```
import pandas as pd
iris_data = [...] # Your list of data
iris_df = pd.DataFrame(iris_data, columns=["Sepal.Length", "Sepal.Width", "Petal.Length", "Petal.Width", "Species"])
PetalLength = iris_df["Petal.Length"].astype(float)
```
from keras.models import Sequential from keras.layers import Dense, Activation # 定义模型结构 model = Sequential() model.add(Dense(units=16, input_shape=(4,))) model.add(Activation('relu')) model.add(Dense(16)) model.add(Activation('relu')) model.add(Dense(3)) model.add(Activation('softmax')) #定义损失函数和优化器,并编译 model.compile(loss='categorical_crossentropy', optimizer='adam', metrics=["accuracy"]) import pandas as pd from sklearn.model_selection import train_test_split from keras.utils import np_utils filename = 'data\iris.data' data = pd.read_csv(filename, header = None) data.columns = ['sepal length','sepal width','petal length','petal width','class'] data.iloc[0:5,:] #数据预处理 #convert classname to integer data.loc[ data['class'] == 'Iris-setosa', 'class' ] = 0 data.loc[ data['class'] == 'Iris-versicolor', 'class' ] = 1 data.loc[ data['class'] == 'Iris-virginica', 'class' ] = 2 #data X = data.iloc[:,0:4].values.astype(float) y = data.iloc[:,4].values.astype(int) train_x, test_x, train_y, test_y = train_test_split(X, y, train_size=0.8, test_size=0.2, random_state=0) #keras多分类问题需要将类型转化为独热矩阵 #与pd.get_dummies()函数作用一致 train_y_ohe = np_utils.to_categorical(train_y, 3) test_y_ohe = np_utils.to_categorical(test_y, 3) #print(test_y_ohe ) #训练模型 model.fit(train_x, train_y_ohe, epochs=50, batch_size=1, verbose=2, validation_data=(test_x,test_y_ohe)) # 评估模型 loss, accuracy = model.evaluate(test_x, test_y_ohe, verbose=2) print('loss = {},accuracy = {} '.format(loss,accuracy) ) # 查看预测结果 classes = model.predict(test_x, batch_size=1, verbose=2) print('测试样本数:',len(classes)) print("分类概率:\n",classes)
这段代码是一个使用Keras库进行机器学习的示例,主要针对鸢尾花数据集进行多分类预测。
首先,使用Pandas读取数据集,并对类别进行处理,转化为数字标签。然后将数据集划分为训练集和测试集,同时将类别转化为独热编码。
接下来,使用Keras库定义一个Sequential模型,添加三个Dense层和两个Activation层,最后使用softmax激活函数进行多分类预测。定义损失函数和优化器,并编译模型。
接着使用fit函数对模型进行训练,设置训练的轮数epochs为50,批次大小batch_size为1。同时指定验证集为测试集,verbose参数为2表示打印训练过程中的详细信息。
训练完成后,使用evaluate函数评估模型的性能,计算损失函数和准确率。最后使用predict函数对测试集进行预测,输出分类概率和测试样本数。
需要注意的是,这段代码中缺少导入numpy库的语句,需要在开头添加以下语句:
```python
import numpy as np
```
阅读全文
相关推荐
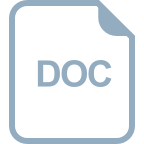
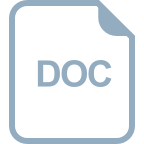
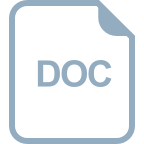





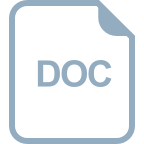
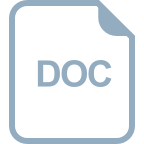
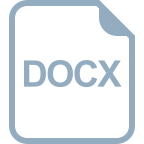
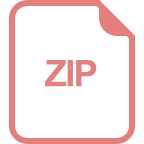