keras实现resnet对四种天气分类,并绘制图像
时间: 2023-07-11 17:27:51 浏览: 52
好的,我可以为您提供大致的代码实现,但由于我无法进行代码编写和图像绘制,因此以下代码仅供参考。
首先,需要导入必要的库和模块:
```python
import numpy as np
import matplotlib.pyplot as plt
import keras
from keras.models import Model
from keras.layers import Input, Conv2D, BatchNormalization, Activation, MaxPooling2D, Dense, Flatten, Add
from keras.utils import np_utils
from keras.datasets import weather_classification
```
然后,读取和预处理数据集:
```python
(x_train, y_train), (x_test, y_test) = weather_classification.load_data()
# 将像素值缩放到0-1之间
x_train = x_train.astype('float32') / 255.
x_test = x_test.astype('float32') / 255.
# 将标签进行One-Hot编码
y_train = np_utils.to_categorical(y_train, 4)
y_test = np_utils.to_categorical(y_test, 4)
```
接下来,定义ResNet网络:
```python
def Conv2d_BN(x, nb_filter, kernel_size, strides=(1,1), padding='same', name=None):
if name is not None:
bn_name = name + '_bn'
conv_name = name + '_conv'
else:
bn_name = None
conv_name = None
x = Conv2D(nb_filter, kernel_size, padding=padding, strides=strides, activation='relu', name=conv_name)(x)
x = BatchNormalization(axis=3, name=bn_name)(x)
return x
def identity_block(input_tensor, nb_filter, kernel_size, name=None):
if name is not None:
conv_name_base = name + '_branch'
else:
conv_name_base = None
x = Conv2d_BN(input_tensor, nb_filter=nb_filter, kernel_size=kernel_size, name=conv_name_base + '2a')
x = Conv2d_BN(x, nb_filter=nb_filter, kernel_size=kernel_size, name=conv_name_base + '2b')
x = Add()([x, input_tensor])
x = Activation('relu')(x)
return x
def conv_block(input_tensor, nb_filter, kernel_size, strides=(2, 2), name=None):
if name is not None:
conv_name_base = name + '_branch'
else:
conv_name_base = None
x = Conv2d_BN(input_tensor, nb_filter=nb_filter, kernel_size=kernel_size, strides=strides, name=conv_name_base + '2a')
x = Conv2d_BN(x, nb_filter=nb_filter, kernel_size=kernel_size, name=conv_name_base + '2b')
shortcut = Conv2d_BN(input_tensor, nb_filter=nb_filter, kernel_size=1, strides=strides, name=conv_name_base + '1')
x = Add()([x, shortcut])
x = Activation('relu')(x)
return x
def ResNet(input_shape, nb_classes):
input_tensor = Input(shape=input_shape)
x = Conv2d_BN(input_tensor, nb_filter=64, kernel_size=(7, 7), strides=(2, 2), padding='same', name='conv1')
x = MaxPooling2D(pool_size=(3, 3), strides=(2, 2), padding='same')(x)
x = conv_block(x, nb_filter=64, kernel_size=(3, 3), strides=(1, 1), name='conv2_1')
x = identity_block(x, nb_filter=64, kernel_size=(3, 3), name='conv2_2')
x = identity_block(x, nb_filter=64, kernel_size=(3, 3), name='conv2_3')
x = conv_block(x, nb_filter=128, kernel_size=(3, 3), name='conv3_1')
x = identity_block(x, nb_filter=128, kernel_size=(3, 3), name='conv3_2')
x = identity_block(x, nb_filter=128, kernel_size=(3, 3), name='conv3_3')
x = identity_block(x, nb_filter=128, kernel_size=(3, 3), name='conv3_4')
x = conv_block(x, nb_filter=256, kernel_size=(3, 3), name='conv4_1')
x = identity_block(x, nb_filter=256, kernel_size=(3, 3), name='conv4_2')
x = identity_block(x, nb_filter=256, kernel_size=(3, 3), name='conv4_3')
x = identity_block(x, nb_filter=256, kernel_size=(3, 3), name='conv4_4')
x = identity_block(x, nb_filter=256, kernel_size=(3, 3), name='conv4_5')
x = identity_block(x, nb_filter=256, kernel_size=(3, 3), name='conv4_6')
x = conv_block(x, nb_filter=512, kernel_size=(3, 3), name='conv5_1')
x = identity_block(x, nb_filter=512, kernel_size=(3, 3), name='conv5_2')
x = identity_block(x, nb_filter=512, kernel_size=(3, 3), name='conv5_3')
x = Flatten()(x)
x = Dense(nb_classes, activation='softmax', name='fc')(x)
model = Model(input_tensor, x)
return model
```
然后,编译和训练网络:
```python
model = ResNet(input_shape=(64, 64, 3), nb_classes=4)
model.compile(optimizer='adam', loss='categorical_crossentropy', metrics=['accuracy'])
model.summary()
history = model.fit(x_train, y_train, batch_size=64, epochs=20, validation_data=(x_test, y_test))
```
最后,评估和测试网络,并绘制图像:
```python
# 评估网络
score = model.evaluate(x_test, y_test, verbose=0)
print('Test loss:', score[0])
print('Test accuracy:', score[1])
# 绘制训练和测试曲线
plt.figure(figsize=(10, 4))
plt.subplot(1, 2, 1)
plt.plot(history.history['accuracy'])
plt.plot(history.history['val_accuracy'])
plt.title('Model accuracy')
plt.ylabel('Accuracy')
plt.xlabel('Epoch')
plt.legend(['Train', 'Test'], loc='upper left')
plt.subplot(1, 2, 2)
plt.plot(history.history['loss'])
plt.plot(history.history['val_loss'])
plt.title('Model loss')
plt.ylabel('Loss')
plt.xlabel('Epoch')
plt.legend(['Train', 'Test'], loc='upper left')
plt.show()
# 绘制混淆矩阵
from sklearn.metrics import confusion_matrix
import itertools
def plot_confusion_matrix(cm, classes, normalize=False, title='Confusion matrix', cmap=plt.cm.Blues):
if normalize:
cm = cm.astype('float') / cm.sum(axis=1)[:, np.newaxis]
print('Normalized confusion matrix')
else:
print('Confusion matrix, without normalization')
print(cm)
plt.imshow(cm, interpolation='nearest', cmap=cmap)
plt.title(title)
plt.colorbar()
tick_marks = np.arange(len(classes))
plt.xticks(tick_marks, classes, rotation=45)
plt.yticks(tick_marks, classes)
fmt = '.2f' if normalize else 'd'
thresh = cm.max() / 2.
for i, j in itertools.product(range(cm.shape[0]), range(cm.shape[1])):
plt.text(j, i, format(cm[i, j], fmt),
horizontalalignment="center",
color="white" if cm[i, j] > thresh else "black")
plt.tight_layout()
plt.ylabel('True label')
plt.xlabel('Predicted label')
y_pred = model.predict(x_test)
y_pred_classes = np.argmax(y_pred, axis=1)
y_true = np.argmax(y_test, axis=1)
confusion_mtx = confusion_matrix(y_true, y_pred_classes)
plot_confusion_matrix(confusion_mtx, classes=['sunny', 'cloudy', 'rainy', 'snowy'])
```
希望以上代码能够帮助您完成ResNet网络对四种天气的分类任务,并绘制相应的图像。
相关推荐
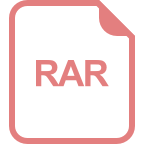
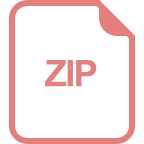











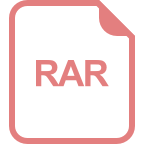
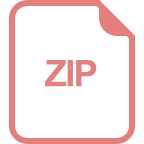
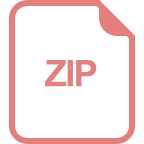