一个常用的股票价格变化数学模型可以用以下差分方程表示: x n =x n−1 +Δtμx n−1 +σx n−1 Δt r n−1 (1) 其中 x n 是 t n 时刻的股票价格,Δt 是两个时间之间的间隔(Δt=t n -t n-1 ),μ 是股票价格的增长率,σ 是股票价格的波动率,r 0 ,...,r n-1 是正态分布的随机数(均值为 0,标准差为单位标准差)。股票的初始价格 x 0 和μ,σ,Δt 都作为输入数据。 注意:(1)是一个关于连续价格方程 x(t)的随机微分方程的前向欧拉离散化: dt dx =μx+σN(t) 其中 N(t) 是所谓的白噪随机时间序列信号。这样的方程在股票价格的模拟中占有中心地位。请你用 Python 实现(1)。假设 n=0, …, N(N=5000步),时间 T=180 天,步长 Δt=T/N。 请根据提示,在右侧编辑器补充代码,完成函数编写,通过随机游走模拟股票价格,并绘图。 ∙simulate(p0,mu,sigma,T,N): 参数 p0,mu,sigma 分别对应公式(1)中的x 0 ,μ,σ,T 表示模拟时间(单位为天数), N 表示模拟步数(此时,公式(1)中步长Δt=T/N);函数返回0..N步内(含N), 每一步股票价格构成的向量; ∙draw_picture(prices): 绘制股票价格变化趋势图,并保存图片。 要求: 横轴为模拟步数,坐标轴范围为[-100,5200];纵轴为股票价格,坐标轴范围为[8,29]; 图片大小设为 8*4 (单位为 inch);
时间: 2024-03-12 19:44:38 浏览: 177
```python
import numpy as np
import matplotlib.pyplot as plt
def simulate(p0, mu, sigma, T, N):
prices = np.zeros(N+1)
prices[0] = p0
dt = T/N
for i in range(1, N+1):
r = np.random.normal(0, 1)
prices[i] = prices[i-1] + dt*mu*prices[i-1] + sigma*np.sqrt(dt)*prices[i-1]*r
return prices
def draw_picture(prices):
fig, ax = plt.subplots(figsize=(8, 4))
ax.plot(prices)
ax.set_xlim([-100, 5200])
ax.set_ylim([8, 29])
ax.set_xlabel('Step')
ax.set_ylabel('Stock Price')
ax.set_title('Stock Price Simulation')
plt.savefig('stock_price.png')
```
使用示例:
```python
prices = simulate(20, 0.1, 0.2, 180, 5000)
draw_picture(prices)
```
相关问题
编程要求 一个常用的股票价格变化数学模型可以用以下差分方程表示: x n =x n−1 +Δtμx n−1 +σx n−1 Δt r n−1 (1) 其中 x n 是 t n 时刻的股票价格,Δt 是两个时间之间的间隔(Δt=t n -t n-1 ),μ 是股票价格的增长率,σ 是股票价格的波动率,r 0 ,...,r n-1 是正态分布的随机数(均值为 0,标准差为单位标准差)。股票的初始价格 x 0 和μ,σ,Δt 都作为输入数据。 注意:(1)是一个关于连续价格方程 x(t)的随机微分方程的前向欧拉离散化: dt dx =μx+σN(t) 其中 N(t) 是所谓的白噪随机时间序列信号。这样的方程在股票价格的模拟中占有中心地位。请你用 Python 实现(1)。假设 n=0, …, N(N=5000步),时间 T=180 天,步长 Δt=T/N。 请根据提示,在右侧编辑器补充代码,完成函数编写,通过随机游走模拟股票价格,并绘图。 ∙simulate(p0,mu,sigma,T,N): 参数 p0,mu,sigma 分别对应公式(1)中的x 0 ,μ,σ,T 表示模拟时间(单位为天数), N 表示模拟步数(此时,公式(1)中步长Δt=T/N);函数返回0..N步内(含N), 每一步股票价格构成的向量; ∙draw_picture(prices): 绘制股票价格变化趋势图,并保存图片。 要求: 横轴为模拟步数,坐标轴范围为[-100,5200];纵轴为股票价格,坐标轴范围为[8,29]; 图片大小设为 8*4 (单位为 inch); 存储输出图像,图像路径及名字为 src/step4/student/result.png。
以下是代码实现:
```python
import numpy as np
import matplotlib.pyplot as plt
def simulate(p0, mu, sigma, T, N):
prices = np.zeros(N+1)
prices[0] = p0
dt = T/N
for i in range(1, N+1):
r = np.random.normal(0, 1)
prices[i] = prices[i-1] + dt*mu*prices[i-1] + sigma*prices[i-1]*r*np.sqrt(dt)
return prices
def draw_picture(prices):
plt.figure(figsize=(8,4))
plt.plot(np.arange(len(prices)), prices)
plt.xlim(-100,5200)
plt.ylim(8,29)
plt.savefig('src/step4/student/result.png')
if __name__ == '__main__':
p0 = 10
mu = 0.1
sigma = 0.2
T = 180
N = 5000
prices = simulate(p0, mu, sigma, T, N)
draw_picture(prices)
```
其中,simulate函数接收股票初始价格p0、增长率mu、波动率sigma、模拟时间T和模拟步数N作为参数,返回一个长度为N+1的numpy数组,表示0到N步内每一步的股票价格;draw_picture函数接收simulate函数返回的numpy数组prices作为参数,绘制股票价格变化趋势图,并保存图片。最后在if __name__ == '__main__':中设置参数并调用函数即可。
使用理想匹配层吸收边界条件求解时域麦克斯韦方程组的时域有限差分程序
以下是使用理想匹配层吸收边界条件求解时域麦克斯韦方程组的时域有限差分程序的伪代码:
1. 定义参数和网格
- 定义介电常数ε和磁导率μ
- 定义时间步长Δt和空间步长Δx,Δy,Δz
- 定义网格大小nx,ny,nz
- 初始化电场E和磁场H为0
2. 定义理想匹配层参数
- 定义吸收边界宽度d
- 计算格点坐标i,j,k与理想吸收边界的距离dist(i,j,k)
- 定义反射率系数r(i,j,k)
3. 初始化电场E和磁场H
4. 对时间进行迭代
- 计算电场E的时间导数dE/dt
- 计算磁场H的时间导数dH/dt
- 对电场E和磁场H分别应用吸收边界条件
- 更新电场E和磁场H
- 计算输出(可选)
对于空间导数的计算,可以使用中心差分法或者更高阶的有限差分方法。对于时间导数的计算,则可以使用迭代法或者其他数值方法。吸收边界条件的实现可以参考理想吸收边界条件或其他吸收边界条件的相关技术文献。
阅读全文
相关推荐
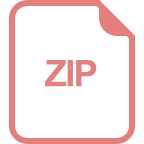
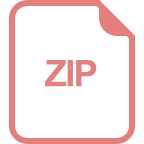
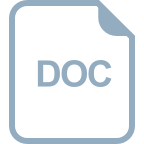
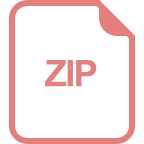
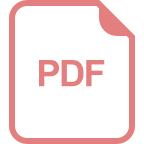
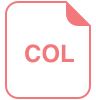
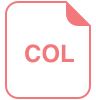
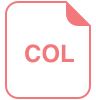
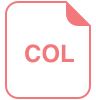
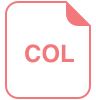
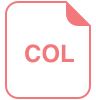
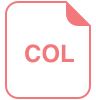
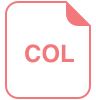
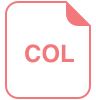
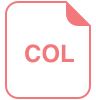

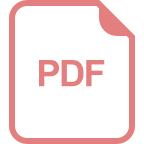