def save_fig(self): self.fig.savefig(self.output_filename, dpi=1000)
时间: 2024-02-19 18:00:41 浏览: 19
这是一个用于保存图形的方法。其中,self.fig表示当前绘图对象,savefig()函数用于将当前绘图对象保存为图片文件。self.output_filename表示保存的文件名,dpi=1000表示设置图片的分辨率为1000。通过这个方法,我们可以将绘制好的图形保存为一个图片文件,以便于后续的使用和分享。
相关问题
import tkinter as tk import pandas as pd import matplotlib.pyplot as plt from matplotlib.backends.backend_tkagg import FigureCanvasTkAgg import os class ExcelPlotter(tk.Frame): def init(self, master=None): super().init(master) self.master = master self.master.title("图方便") self.file_label = tk.Label(master=self, text="Excel File Path:") self.file_label.grid(row=0, column=0, sticky="w") self.file_entry = tk.Entry(master=self) self.file_entry.grid(row=0, column=1, columnspan=2, sticky="we") self.file_button = tk.Button(master=self, text="Open", command=self.open_file) self.file_button.grid(row=0, column=3, sticky="e") self.plot_button = tk.Button(master=self, text="Plot", command=self.plot_data) self.plot_button.grid(row=1, column=2, sticky="we") self.name_label = tk.Label(master=self, text="Out Image Name:") self.name_label.grid(row=2, column=0, sticky="w") self.name_entry = tk.Entry(master=self) self.name_entry.grid(row=2, column=1, columnspan=2, sticky="we") self.save_button = tk.Button(master=self, text="Save", command=self.save_image) self.save_button.grid(row=2, column=3, sticky="e") self.figure = plt.figure(figsize=(5, 4), dpi=150) self.canvas = FigureCanvasTkAgg(self.figure, master=self) self.canvas.get_tk_widget().grid(row=4, column=0, columnspan=4, sticky="we") self.pack() def open_file(self): file_path = tk.filedialog.askopenfilename(filetypes=[("Excel Files", "*.xls")]) self.file_entry.delete(0, tk.END) self.file_entry.insert(tk.END, file_path) def plot_data(self): file_path = self.file_entry.get() if os.path.exists(file_path): data = pd.read_excel(file_path) plt.plot(data['波长(nm)'], data['吸光度'], 'k') plt.xlim(300, 1000) plt.xlabel('Wavelength(nm)', fontsize=16) plt.ylabel('Abs.', fontsize=16) plt.gcf().subplots_adjust(left=0.13, top=0.91, bottom=0.16) plt.savefig('Last Fig', dpi=1000) plt.show() def save_image(self): if self.figure: file_path = tk.filedialog.asksaveasfilename(defaultextension=".png") if file_path: self.figure.savefig(file_path) root = tk.Tk() app = ExcelPlotter(master=root) app.mainloop()帮我增加一个删除当前图像的功能
好的,您可以在 `ExcelPlotter` 类中添加一个名为 `delete_image` 的方法,实现删除当前图像的功能。可以在该方法中调用 `self.figure.clf()` 清空当前图像,然后在界面上更新显示。下面是修改后的代码:
```python
import tkinter as tk
import pandas as pd
import matplotlib.pyplot as plt
from matplotlib.backends.backend_tkagg import FigureCanvasTkAgg
import os
class ExcelPlotter(tk.Frame):
def __init__(self, master=None):
super().__init__(master)
self.master = master
self.master.title("图方便")
self.file_label = tk.Label(master=self, text="Excel File Path:")
self.file_label.grid(row=0, column=0, sticky="w")
self.file_entry = tk.Entry(master=self)
self.file_entry.grid(row=0, column=1, columnspan=2, sticky="we")
self.file_button = tk.Button(master=self, text="Open", command=self.open_file)
self.file_button.grid(row=0, column=3, sticky="e")
self.plot_button = tk.Button(master=self, text="Plot", command=self.plot_data)
self.plot_button.grid(row=1, column=2, sticky="we")
self.delete_button = tk.Button(master=self, text="Delete", command=self.delete_image)
self.delete_button.grid(row=1, column=3, sticky="e")
self.name_label = tk.Label(master=self, text="Out Image Name:")
self.name_label.grid(row=2, column=0, sticky="w")
self.name_entry = tk.Entry(master=self)
self.name_entry.grid(row=2, column=1, columnspan=2, sticky="we")
self.save_button = tk.Button(master=self, text="Save", command=self.save_image)
self.save_button.grid(row=2, column=3, sticky="e")
self.figure = plt.figure(figsize=(5, 4), dpi=150)
self.canvas = FigureCanvasTkAgg(self.figure, master=self)
self.canvas.get_tk_widget().grid(row=4, column=0, columnspan=4, sticky="we")
self.pack()
def open_file(self):
file_path = tk.filedialog.askopenfilename(filetypes=[("Excel Files", "*.xls")])
self.file_entry.delete(0, tk.END)
self.file_entry.insert(tk.END, file_path)
def plot_data(self):
file_path = self.file_entry.get()
if os.path.exists(file_path):
data = pd.read_excel(file_path)
plt.plot(data['波长(nm)'], data['吸光度'], 'k')
plt.xlim(300, 1000)
plt.xlabel('Wavelength(nm)', fontsize=16)
plt.ylabel('Abs.', fontsize=16)
plt.gcf().subplots_adjust(left=0.13, top=0.91, bottom=0.16)
plt.show()
def delete_image(self):
if self.figure:
self.figure.clf()
self.canvas.draw()
def save_image(self):
if self.figure:
file_path = tk.filedialog.asksaveasfilename(defaultextension=".png")
if file_path:
self.figure.savefig(file_path)
root = tk.Tk()
app = ExcelPlotter(master=root)
app.mainloop()
```
在界面上添加了一个名为 `Delete` 的按钮,点击该按钮会调用 `delete_image` 方法。该方法会清空当前图像并在界面上更新显示。
import pandas as pd import warnings import sklearn.datasets import sklearn.linear_model import matplotlib import matplotlib.font_manager as fm import matplotlib.pyplot as plt import numpy as np import seaborn as sns data = pd.read_excel(r'C:\Users\Lenovo\Desktop\data.xlsx') print(data.info()) fig = plt.figure(figsize=(10, 8)) sns.heatmap(data.corr(), cmap="YlGnBu", annot=True) plt.title('相关性分析热力图') plt.rcParams['axes.unicode_minus'] = False plt.rcParams['font.sans-serif'] = 'SimHei' plt.show() y = data['y'] x = data.drop(['y'], axis=1) print('************************输出新的特征集数据***************************') print(x.head()) from sklearn.model_selection import train_test_split x_train, x_test, y_train, y_test = train_test_split(x, y, test_size=0.2, random_state=42) def relu(x): output=np.maximum(0, x) return output def relu_back_propagation(derror_wrt_output,x): derror_wrt_dinputs = np.array(derror_wrt_output, copy=True) derror_wrt_dinputs[x <= 0] = 0 return derror_wrt_dinputs def activated(activation_choose,x): if activation_choose == 'relu': return relu(x) def activated_back_propagation(activation_choose, derror_wrt_output, output): if activation_choose == 'relu': return relu_back_propagation(derror_wrt_output, output) class NeuralNetwork: def __init__(self, layers_strcuture, print_cost = False): self.layers_strcuture = layers_strcuture self.layers_num = len(layers_strcuture) self.param_layers_num = self.layers_num - 1 self.learning_rate = 0.0618 self.num_iterations = 2000 self.x = None self.y = None self.w = dict() self.b = dict() self.costs = [] self.print_cost = print_cost self.init_w_and_b() def set_learning_rate(self,learning_rate): self.learning_rate=learning_rate def set_num_iterations(self, num_iterations): self.num_iterations = num_iterations def set_xy(self, input, expected_output): self.x = input self.y = expected_output
这段代码存在两个问题:
1. 在import语句中没有使用逗号分隔符,应该在每个import语句之间添加逗号分隔符。
2. 在函数set_xy()的定义中,函数体没有缩进,应该在函数体内部添加缩进。
以下是修改后的代码:
```python
import pandas as pd, warnings
import sklearn.datasets, sklearn.linear_model
import matplotlib, matplotlib.font_manager as fm
import matplotlib.pyplot as plt
import numpy as np
import seaborn as sns
data = pd.read_excel(r'C:\Users\Lenovo\Desktop\data.xlsx')
print(data.info())
fig = plt.figure(figsize=(10, 8))
sns.heatmap(data.corr(), cmap="YlGnBu", annot=True)
plt.title('相关性分析热力图')
plt.rcParams['axes.unicode_minus'] = False
plt.rcParams['font.sans-serif'] = 'SimHei'
plt.show()
y = data['y']
x = data.drop(['y'], axis=1)
print('************************输出新的特征集数据***************************')
print(x.head())
from sklearn.model_selection import train_test_split
x_train, x_test, y_train, y_test = train_test_split(x, y, test_size=0.2, random_state=42)
def relu(x):
output=np.maximum(0, x)
return output
def relu_back_propagation(derror_wrt_output,x):
derror_wrt_dinputs = np.array(derror_wrt_output, copy=True)
derror_wrt_dinputs[x <= 0] = 0
return derror_wrt_dinputs
def activated(activation_choose,x):
if activation_choose == 'relu':
return relu(x)
def activated_back_propagation(activation_choose, derror_wrt_output, output):
if activation_choose == 'relu':
return relu_back_propagation(derror_wrt_output, output)
class NeuralNetwork:
def __init__(self, layers_strcuture, print_cost = False):
self.layers_strcuture = layers_strcuture
self.layers_num = len(layers_strcuture)
self.param_layers_num = self.layers_num - 1
self.learning_rate = 0.0618
self.num_iterations = 2000
self.x = None
self.y = None
self.w = dict()
self.b = dict()
self.costs = []
self.print_cost = print_cost
self.init_w_and_b()
def set_learning_rate(self,learning_rate):
self.learning_rate=learning_rate
def set_num_iterations(self, num_iterations):
self.num_iterations = num_iterations
def set_xy(self, input, expected_output):
self.x = input
self.y = expected_output
```
相关推荐
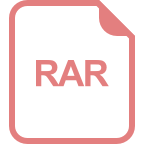
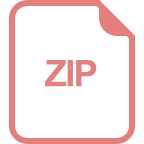







