优化这段代码dataset = pd.get_dummies(data=dataset, columns=[ 'PdDistrict'], drop_first = True) print(dataset)
时间: 2023-06-09 22:08:42 浏览: 69
你对这段代码的疑问是什么?是否可以更加高效的编写方式?
我的确没有理解您的第一个问题所在,这段代码可以一次性将 'PdDistrict' 列转化为多列(每列代表一个 district),这是独热编码的一种实现方式。
如果您希望更加高效的方式,可以考虑使用 sklearn.preprocessing 的 OneHotEncoder 实现,这是一个专门用来进行独热编码的工具包,效率比 pd.get_dummies 更高。
例子:
```python
from sklearn.preprocessing import OneHotEncoder
enc = OneHotEncoder(handle_unknown='ignore')
enc.fit_transform(dataset[['PdDistrict']])
```
其中 `handle_unknown='ignore'` 表示如果出现未知的 district,就忽略,这样不会报错。
相关问题
import seaborn as sns import pandas as pd import matplotlib.pyplot as plt from sklearn.model_selection import train_test_split from sklearn.preprocessing import StandardScaler from sklearn.linear_model import LogisticRegression df = pd.read_csv('heart.csv') corrmat = df.corr() top_corr_features = corrmat.index plt.figure(figsize=(16,16)) sns.heatmap(df[top_corr_features].corr(),annot=True,cmap="RdYlGn") plt.show() sns.set_style('whitegrid') sns.countplot(x='target',data=df,palette='RdBu_r') plt.show() dataset = pd.get_dummies(df, columns=['sex', 'cp', 'fbs', 'restecg', 'exang', 'slope', 'ca', 'thal']) columns_to_scale = ['age', 'trestbps', 'chol', 'thalach', 'oldpeak'] scaler = StandardScaler() dataset[columns_to_scale] = scaler.fit_transform(dataset[columns_to_scale]) dataset.head() y = dataset['target'] X = dataset.drop(['target'], axis=1) X_train, X_test, y_train, y_test = train_test_split(X, y, test_size=0.3, random_state=0) logreg = LogisticRegression() logreg.fit(X_train, y_train) print("Training accuracy: {:.3f}".format(logreg.score(X_train, y_train))) print("Test accuracy: {:.3f}".format(logreg.score(X_test, y_test)))改写为ROC代码
以下是将 Logistic Regression 模型的评估指标改为 ROC 曲线的代码:
```python
import seaborn as sns
import pandas as pd
import matplotlib.pyplot as plt
from sklearn.model_selection import train_test_split
from sklearn.preprocessing import StandardScaler
from sklearn.linear_model import LogisticRegression
from sklearn.metrics import roc_curve, auc
df = pd.read_csv('heart.csv')
# 查看特征相关性
corrmat = df.corr()
top_corr_features = corrmat.index
plt.figure(figsize=(16,16))
sns.heatmap(df[top_corr_features].corr(),annot=True,cmap="RdYlGn")
plt.show()
# 查看数据分布
sns.set_style('whitegrid')
sns.countplot(x='target',data=df,palette='RdBu_r')
plt.show()
# 对数据进行 One-hot 编码和标准化
dataset = pd.get_dummies(df, columns=['sex', 'cp', 'fbs', 'restecg', 'exang', 'slope', 'ca', 'thal'])
columns_to_scale = ['age', 'trestbps', 'chol', 'thalach', 'oldpeak']
scaler = StandardScaler()
dataset[columns_to_scale] = scaler.fit_transform(dataset[columns_to_scale])
dataset.head()
# 划分数据集
y = dataset['target']
X = dataset.drop(['target'], axis=1)
X_train, X_test, y_train, y_test = train_test_split(X, y, test_size=0.3, random_state=0)
# 训练模型
logreg = LogisticRegression()
logreg.fit(X_train, y_train)
# 评估模型
y_train_pred = logreg.predict_proba(X_train)[:, 1]
y_test_pred = logreg.predict_proba(X_test)[:, 1]
fpr_train, tpr_train, thresholds_train = roc_curve(y_train, y_train_pred)
fpr_test, tpr_test, thresholds_test = roc_curve(y_test, y_test_pred)
roc_auc_train = auc(fpr_train, tpr_train)
roc_auc_test = auc(fpr_test, tpr_test)
# 绘制 ROC 曲线
plt.figure()
plt.plot(fpr_train, tpr_train, color='darkorange', lw=2, label='Train ROC curve (area = %0.2f)' % roc_auc_train)
plt.plot(fpr_test, tpr_test, color='navy', lw=2, label='Test ROC curve (area = %0.2f)' % roc_auc_test)
plt.plot([0, 1], [0, 1], color='black', lw=2, linestyle='--')
plt.xlim([0.0, 1.0])
plt.ylim([0.0, 1.05])
plt.xlabel('False Positive Rate')
plt.ylabel('True Positive Rate')
plt.title('Receiver operating characteristic')
plt.legend(loc="lower right")
plt.show()
```
在这个代码中,我们使用 `roc_curve` 函数计算训练集和测试集的 FPR 和 TPR,然后使用 `auc` 函数计算 ROC 曲线下的面积。最后,我们使用 `matplotlib` 库绘制 ROC 曲线。
pd.get_dummies
pd.get_dummies is a Python function from the pandas library that is used to create dummy variables from categorical data. It creates a new column for each unique category of a categorical variable, and assigns a value of 1 or 0 to each row depending on whether that row belongs to that category or not. This is useful for machine learning algorithms that require numerical input, as it converts non-numerical data into a numerical format.
For example, if we have a dataset with a categorical variable "color" that has three categories: red, green, and blue, pd.get_dummies will create three new columns in the dataset called "color_red", "color_green", and "color_blue". Each row will have a value of 1 in the column that corresponds to its color, and 0 in the other two columns.
The syntax for pd.get_dummies is:
```
pd.get_dummies(data, columns=None, prefix=None, prefix_sep='_', dummy_na=False, drop_first=False)
```
- data: the input pandas DataFrame or Series
- columns: the name or list of names of the columns to encode. If not specified, all non-numerical columns will be encoded.
- prefix: the prefix to add to the column names of the new dummy variables
- prefix_sep: the separator to use between the prefix and the original column name
- dummy_na: whether to create an additional column for missing values. If True, a column called "column_name_nan" will be created for each column with missing values.
- drop_first: whether to drop the first column of each set of dummy variables to avoid multicollinearity. If True, the first column will be dropped.
相关推荐
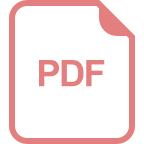
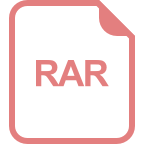












