when { aHeadsUp != bHeadsUp -> if (aHeadsUp) -1 else 1 // Provide consistent ranking with headsUpManager aHeadsUp -> headsUpManager.compare(a, b) aIsFsn != bIsFsn -> if (aIsFsn) -1 else 1 usePeopleFiltering && aPersonType != bPersonType -> peopleNotificationIdentifier.compareTo(aPersonType, bPersonType) // Upsort current media notification. aMedia != bMedia -> if (aMedia) -1 else 1 // Upsort PRIORITY_MAX system notifications aSystemMax != bSystemMax -> if (aSystemMax) -1 else 1 aIsHighPriority != bIsHighPriority -> -1 * aIsHighPriority.compareTo(bIsHighPriority) aRank != bRank -> aRank - bRank else -> nb.notification.`when`.compareTo(na.notification.`when`) } 请转为java
时间: 2024-02-10 18:13:11 浏览: 22
这段代码是 Kotlin 语言的代码,可以转化为 Java 语言的代码,如下所示:
```
Comparator<StatusBarNotification> notificationComparator = new Comparator<StatusBarNotification>() {
@Override
public int compare(StatusBarNotification a, StatusBarNotification b) {
boolean aHeadsUp = isHeadsUp(a);
boolean bHeadsUp = isHeadsUp(b);
boolean aIsFsn = isForegroundService(a);
boolean bIsFsn = isForegroundService(b);
boolean aMedia = isMediaNotification(a);
boolean bMedia = isMediaNotification(b);
boolean aSystemMax = a.getNotification().priority == Notification.PRIORITY_MAX;
boolean bSystemMax = b.getNotification().priority == Notification.PRIORITY_MAX;
boolean aIsHighPriority = a.getNotification().priority >= Notification.PRIORITY_HIGH;
boolean bIsHighPriority = b.getNotification().priority >= Notification.PRIORITY_HIGH;
int aRank = getRank(a);
int bRank = getRank(b);
int result = 0;
if (aHeadsUp != bHeadsUp) {
result = aHeadsUp ? -1 : 1;
} else if (aHeadsUp) {
result = headsUpManager.compare(a, b);
} else if (aIsFsn != bIsFsn) {
result = aIsFsn ? -1 : 1;
} else if (usePeopleFiltering && a.getNotification().extras != null &&
b.getNotification().extras != null) {
String aPersonType = a.getNotification().extras.getString(EXTRA_PEOPLE_TYPE);
String bPersonType = b.getNotification().extras.getString(EXTRA_PEOPLE_TYPE);
if (aPersonType != null && bPersonType != null &&
!aPersonType.equals(bPersonType)) {
result = peopleNotificationIdentifier.compareTo(aPersonType, bPersonType);
}
}
if (result == 0) {
if (aMedia != bMedia) {
result = aMedia ? -1 : 1;
} else if (aSystemMax != bSystemMax) {
result = aSystemMax ? -1 : 1;
} else if (aIsHighPriority != bIsHighPriority) {
result = -1 * Boolean.compare(aIsHighPriority, bIsHighPriority);
} else if (aRank != bRank) {
result = aRank - bRank;
} else {
result = Long.compare(b.getNotification().when, a.getNotification().when);
}
}
return result;
}
};
```
相关推荐
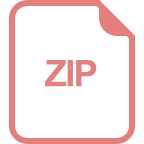
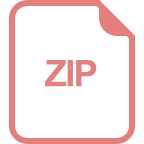
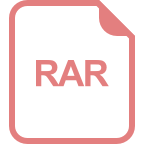














