import numpy as np import matplotlib.pyplot as plt # 创建三个一维矩阵 x = np.array([1, 2, 3, 4]) y = np.array([1, 2, 3]) z = np.array([1, 2, 3, 4, 5, 6, 7, 8, 9]) # 将三个一维矩阵插值到一个二维网格中 xi, yi = np.meshgrid(x, y) zi = np.reshape(z, (len(y), len(x))) # 绘制插值后的二维矩阵结果 plt.imshow(zi) plt.show()
时间: 2023-10-05 19:13:56 浏览: 117
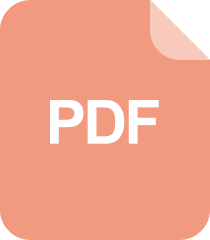
详解numpy.meshgrid()方法使用
这段代码实现了三个一维矩阵的插值,将它们插值到一个二维网格中,并绘制出插值后的二维矩阵结果。具体来说,代码首先导入了 numpy 和 matplotlib.pyplot 两个库,然后创建了三个一维矩阵 x、y、z。
接着,代码使用 meshgrid 函数将 x 和 y 两个一维矩阵插值到一个二维网格中,得到了 xi 和 yi 两个二维矩阵。然后,代码使用 reshape 函数将 z 一维矩阵重新排列成一个 len(y) 行 len(x) 列的二维矩阵 zi,与 xi 和 yi 对应。
最后,代码使用 imshow 函数将插值后的二维矩阵结果 zi 绘制出来,调用 show 函数显示图像。这段代码的作用是可视化插值后的数据,有助于我们更直观地理解数据之间的关系。
阅读全文
相关推荐
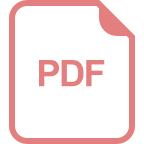




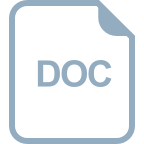
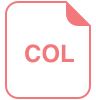
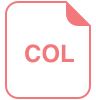
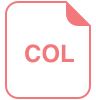
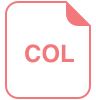
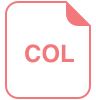
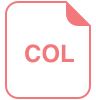
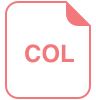
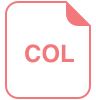



