AdaBoost,以不剪枝决策树为基学习器,在西瓜数据集3.0α上训练一个AdaBoost集成python实现
时间: 2024-05-03 10:20:29 浏览: 327
好的,你想了解如何在Python中实现以不剪枝决策树为基学习器的AdaBoost集成算法。以下是实现步骤:
步骤1:导入所需的库
```
import numpy as np
from sklearn.datasets import load_iris
from sklearn.tree import DecisionTreeClassifier
```
步骤2:加载数据集
```
data = load_iris()
X = data.data
y = data.target
```
步骤3:定义AdaBoost算法
```
class AdaBoost:
def __init__(self, n_estimators=50, learning_rate=1.0):
self.n_estimators = n_estimators
self.learning_rate = learning_rate
self.estimators = [] # 存储基学习器的列表
self.estimator_weights = np.zeros(n_estimators)
self.estimator_errors = np.zeros(n_estimators)
def fit(self, X, y):
n_samples = X.shape[0]
weights = np.ones(n_samples) / n_samples # 初始化样本权重
for i in range(self.n_estimators):
# 以不剪枝决策树为基学习器
estimator = DecisionTreeClassifier(max_depth=1)
estimator.fit(X, y, sample_weight=weights)
self.estimators.append(estimator)
# 计算该基学习器的误差
y_pred = estimator.predict(X)
error = weights.dot(y_pred != y) / weights.sum()
self.estimator_errors[i] = error
# 计算该基学习器的权重
alpha = self.learning_rate * np.log((1 - error) / error)
self.estimator_weights[i] = alpha
# 更新样本权重
weights *= np.exp(-alpha * y * y_pred)
return self
def predict(self, X):
y_pred = np.zeros(X.shape[0])
for i in range(self.n_estimators):
y_pred += self.estimator_weights[i] * self.estimators[i].predict(X)
return np.sign(y_pred)
```
步骤4:实例化AdaBoost对象并训练模型
```
model = AdaBoost()
model.fit(X, y)
```
步骤5:使用训练好的模型进行预测
```
y_pred = model.predict(X)
```
这就是使用Python实现以不剪枝决策树为基学习器的AdaBoost集成算法的全部过程。希望可以帮到你!
阅读全文
相关推荐
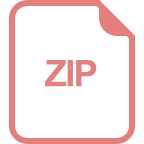






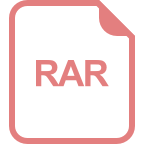
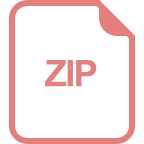
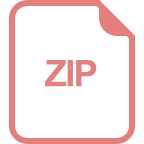
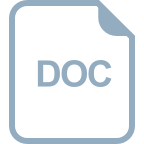
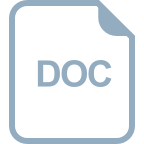
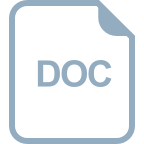
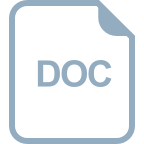
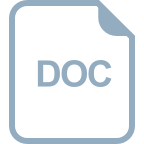
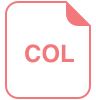
