用python代码实现基于word2vec的关键词聚类模型
时间: 2023-12-22 13:03:24 浏览: 119
下面是一个用Python实现基于word2vec的关键词聚类模型的示例代码:
```python
import gensim
import nltk
import string
from nltk.corpus import stopwords
from sklearn.cluster import KMeans
import matplotlib.pyplot as plt
# 加载停用词
stop_words = stopwords.words('english')
punctuations = list(string.punctuation)
stop_words += punctuations
# 加载文本数据
with open('data.txt', 'r') as f:
text = f.read()
# 分词
tokens = nltk.word_tokenize(text.lower())
# 去除停用词和标点符号
tokens = [token for token in tokens if token not in stop_words]
# 训练词向量模型
model = gensim.models.Word2Vec([tokens], min_count=1, size=100)
# 聚类
X = model[model.wv.vocab]
kmeans = KMeans(n_clusters=5)
kmeans.fit(X)
y_kmeans = kmeans.predict(X)
# 可视化
plt.scatter(X[:, 0], X[:, 1], c=y_kmeans, s=50, cmap='viridis')
centers = kmeans.cluster_centers_
plt.scatter(centers[:, 0], centers[:, 1], c='black', s=200, alpha=0.5)
plt.show()
```
在这个示例代码中,我们首先加载了停用词和文本数据,然后使用NLTK库对文本数据进行分词和去除停用词和标点符号的操作。接着,我们使用gensim库训练了一个词向量模型,并使用K-means算法将单词聚类成5个类别。最后,我们使用matplotlib库将聚类结果可视化。
阅读全文
相关推荐
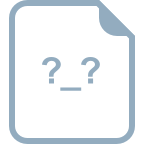
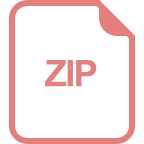

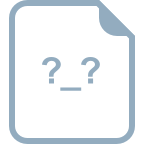



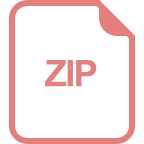
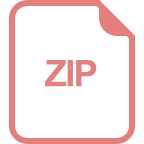
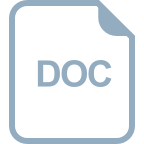
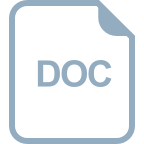
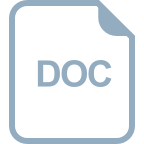
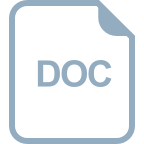
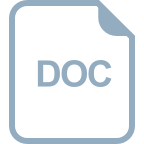


