loss_fn = torch.nn.CrossEntropyLoss() dummy_outputs = torch.rand(4, 10) dummy_labels = torch.tensor([1, 5, 3, 7])
时间: 2024-05-25 10:14:08 浏览: 19
这段代码定义了一个交叉熵损失函数 `CrossEntropyLoss`,并且定义了一个大小为 $4 \times 10$ 的虚拟输出 `dummy_outputs` 和一个大小为 $4$ 的虚拟标签 `dummy_labels`。在实际应用中,你需要将 `dummy_outputs` 替换为你的模型的输出,将 `dummy_labels` 替换为你的样本的真实标签。然后将二者输入到 `CrossEntropyLoss` 中计算损失。交叉熵损失函数常用于多分类问题中计算损失。
相关问题
loss_function=torch.nn.CrossEntropyLoss正则化代码
在PyTorch中,可以使用weight_decay参数来实现L2正则化。同时,由于CrossEntropyLoss已经包含了Softmax操作,因此不需要在模型中额外添加Softmax层。
下面是一个使用CrossEntropyLoss和L2正则化的例子:
```python
import torch
import torch.nn as nn
import torch.optim as optim
# 定义模型
class MyModel(nn.Module):
def __init__(self, input_size, hidden_size, output_size):
super(MyModel, self).__init__()
self.fc1 = nn.Linear(input_size, hidden_size)
self.fc2 = nn.Linear(hidden_size, output_size)
def forward(self, x):
x = torch.relu(self.fc1(x))
x = self.fc2(x)
return x
# 定义超参数
input_size = 10
hidden_size = 20
output_size = 5
learning_rate = 0.01
weight_decay = 0.001
epochs = 10
# 创建模型和优化器
model = MyModel(input_size, hidden_size, output_size)
optimizer = optim.SGD(model.parameters(), lr=learning_rate, weight_decay=weight_decay)
loss_fn = nn.CrossEntropyLoss()
# 准备数据
X = torch.randn(100, input_size)
y = torch.randint(0, output_size, (100,))
# 训练模型
for epoch in range(epochs):
# 前向传播和计算损失
logits = model(X)
loss = loss_fn(logits, y)
# 反向传播和更新参数
optimizer.zero_grad()
loss.backward()
optimizer.step()
# 打印训练信息
if epoch % 1 == 0:
print("Epoch [{}/{}], Loss: {:.4f}".format(epoch+1, epochs, loss.item()))
```
在这个例子中,我们将L2正则化的超参数设为0.001,即weight_decay=0.001。在优化器中使用weight_decay参数即可实现L2正则化。
loss_function = torch.nn.CrossEntropyLoss()
`torch.nn.CrossEntropyLoss()`是一个常用的损失函数。它主要用于多分类问题,计算预测值和真实值之间的交叉熵损失。在训练神经网络时,我们需要使用某种形式的损失函数来衡量模型的预测输出与真实标签之间的差异,以便通过反向传播算法来优化模型参数。
在使用`CrossEntropyLoss()`函数时,我们需要将神经网络的输出与真实标签作为输入。该函数将计算每个类别的预测值与真实标签之间的交叉熵损失,并返回总体损失。具体而言,该函数首先将网络输出的类别概率值通过softmax函数转化为类别概率分布,然后计算预测概率分布和真实标签之间的交叉熵损失。
相关推荐
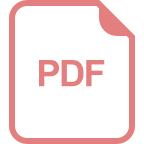
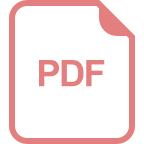
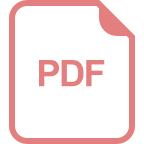













