# transforms t = [] t.append(transforms.ToTensor()) t.append(transforms.Normalize(IMAGENET_DEFAULT_MEAN, IMAGENET_DEFAULT_STD)) preprocess = transforms.Compose(t)
时间: 2024-03-30 12:40:48 浏览: 43
这段代码是使用 PyTorch 中的 transforms 模块对图像进行预处理,其中包含了两个操作,分别是将图像转换为 Tensor 格式和对图像进行归一化处理。IMAGENET_DEFAULT_MEAN 和 IMAGENET_DEFAULT_STD 分别代表了对图像进行归一化的均值和标准差,这两个值是在 ImageNet 数据集上计算得到的。最后,使用 transforms.Compose 将两个操作组合在一起,形成一个预处理的 pipeline。这个 pipeline 在对图像进行预处理的时候,会按照添加的顺序,依次对图像进行处理。
相关问题
对这段代码也使用多线程:def get_image_files(folder): image_extensions = ['.jpg', '.jpeg', '.png'] # 定义图片扩展名 image_files = [] for root, dirs, files in os.walk(folder): for file in files: if os.path.splitext(file)[1].lower() in image_extensions: img_path = os.path.join(root, file) # 将每条数据保存到txt中 path_all_files = open('./all_files.txt','a') path_all_files.write(img_path+'\n') path_all_files.close() print(img_path) image_files.append(img_path) return image_files # 加载ONNX模型 ort_session = onnxruntime.InferenceSession('./best_weights.onnx') # 预处理输入图像 transform = transforms.Compose([ transforms.Resize((224, 224)), transforms.ToTensor(), transforms.Normalize((0.5,), (0.5,)) ]) # 创建线程池 executor = concurrent.futures.ThreadPoolExecutor() # 获取路径下所有图片 image_files = get_image_files('C:/Users/xyzhuge/Desktop/detect-mamaclouds-yolov5-v2/detect-mamaclouds-yolov5-linux-main/res/')
下面是对第二段代码使用多线程的示例:
```python
import concurrent.futures
def get_image_files(folder):
image_extensions = ['.jpg', '.jpeg', '.png'] # 定义图片扩展名
image_files = []
for root, dirs, files in os.walk(folder):
for file in files:
if os.path.splitext(file)[1].lower() in image_extensions:
img_path = os.path.join(root, file)
# 将每条数据保存到txt中
path_all_files = open('./all_files.txt','a')
path_all_files.write(img_path+'\n')
path_all_files.close()
print(img_path)
image_files.append(img_path)
return image_files
# 创建线程池
executor = concurrent.futures.ThreadPoolExecutor()
# 获取路径下所有图片
image_files = get_image_files('C:/Users/xyzhuge/Desktop/detect-mamaclouds-yolov5-v2/detect-mamaclouds-yolov5-linux-main/res/')
# 定义处理图片的函数
def process_image(image_file):
# 加载图片
image = Image.open(image_file)
# 预处理
input_data = transform(image).unsqueeze(0).numpy()
# 运行推理
ort_inputs = {ort_session.get_inputs()[0].name: input_data}
ort_outputs = ort_session.run(None, ort_inputs)
# 获取预测结果
predicted_class = np.argmax(ort_outputs[0])
print(f'Image: {image_file}')
print(f'Predicted class: {predicted_class}')
# 当为0时,删除图片
if predicted_class == 0:
# 将每条数据保存到txt中
path_all_files = open('./del_files.txt','a')
path_all_files.write(image_file+'\n')
path_all_files.close()
os.remove(image_file)
print('Deleted')
# 提交任务给线程池
for image_file in image_files:
executor.submit(process_image, image_file)
# 关闭线程池,等待所有任务完成
executor.shutdown()
```
这段代码与之前的示例类似,将每个图片的处理任务提交给线程池并行执行。注意在多线程环境下使用共享资源时需要进行适当的同步操作。同时,也请确保在适当的位置关闭文件和线程池,以避免资源泄漏。
修改以下代码使其能够输出模型预测结果: def open_image(self): file_dialog = QFileDialog() file_paths, _ = file_dialog.getOpenFileNames(self, "选择图片", "", "Image Files (*.png *.jpg *.jpeg)") if file_paths: self.display_images(file_paths) def preprocess_images(self, image_paths): data_transform = transforms.Compose([ transforms.CenterCrop(150), transforms.ToTensor(), transforms.Normalize([0.485, 0.456, 0.406], [0.229, 0.224, 0.225]) ]) self.current_image_paths = [] images = [] for image_path in image_paths: image = Image.open(image_path) image = data_transform(image) image = torch.unsqueeze(image, dim=0) images.append(image) self.current_image_paths.append(image_path) return images def predict_images(self): if not self.current_image_paths: return for i, image_path in enumerate(self.current_image_paths): image = self.preprocess_image(image_path) output = self.model(image) predicted_class = self.class_dict[output.argmax().item()] self.result_labels[i].setText(f"Predicted Class: {predicted_class}") self.progress_bar.setValue((i+1)*20) def display_images(self, image_paths): for i, image_path in enumerate(image_paths): image = QImage(image_path) image = image.scaled(300, 300, Qt.KeepAspectRatio) if i == 0: self.image_label_1.setPixmap(QPixmap.fromImage(image)) elif i == 1: self.image_label_2.setPixmap(QPixmap.fromImage(image)) elif i == 2: self.image_label_3.setPixmap(QPixmap.fromImage(image)) elif i == 3: self.image_label_4.setPixmap(QPixmap.fromImage(image)) elif i == 4: self.image_label_5.setPixmap(QPixmap.fromImage(image))
请注意,代码中的 preprocess_images 函数和 predict_images 函数中的 self.preprocess_image(image_path) 需要修改为 self.preprocess_images([image_path])。修改后的代码如下:
```
def open_image(self):
file_dialog = QFileDialog()
file_paths, _ = file_dialog.getOpenFileNames(self, "选择图片", "", "Image Files (*.png *.jpg *.jpeg)")
if file_paths:
self.display_images(file_paths)
def preprocess_images(self, image_paths):
data_transform = transforms.Compose([
transforms.CenterCrop(150),
transforms.ToTensor(),
transforms.Normalize([0.485, 0.456, 0.406], [0.229, 0.224, 0.225])
])
self.current_image_paths = []
images = []
for image_path in image_paths:
image = Image.open(image_path)
image = data_transform(image)
image = torch.unsqueeze(image, dim=0)
images.append(image)
self.current_image_paths.append(image_path)
return images
def predict_images(self):
if not self.current_image_paths:
return
for i, image_path in enumerate(self.current_image_paths):
image = self.preprocess_images([image_path])
output = self.model(image[0])
predicted_class = self.class_dict[output.argmax().item()]
self.result_labels[i].setText(f"Predicted Class: {predicted_class}")
self.progress_bar.setValue((i+1)*20)
def display_images(self, image_paths):
for i, image_path in enumerate(image_paths):
image = QImage(image_path)
image = image.scaled(300, 300, Qt.KeepAspectRatio)
if i == 0:
self.image_label_1.setPixmap(QPixmap.fromImage(image))
elif i == 1:
self.image_label_2.setPixmap(QPixmap.fromImage(image))
elif i == 2:
self.image_label_3.setPixmap(QPixmap.fromImage(image))
elif i == 3:
self.image_label_4.setPixmap(QPixmap.fromImage(image))
elif i == 4:
self.image_label_5.setPixmap(QPixmap.fromImage(image))
```
阅读全文
相关推荐
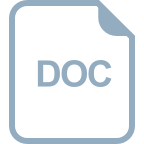
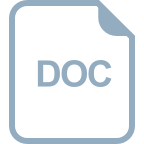
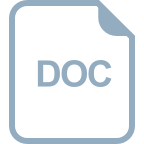










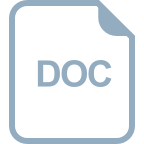
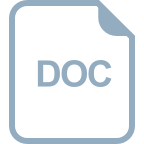
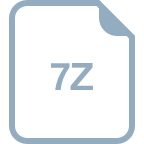
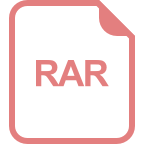