import torch import torch.nn as nn import torch.nn.functional as F from torch.autograd import Variable class Bottleneck(nn.Module): def init(self, last_planes, in_planes, out_planes, dense_depth, stride, first_layer): super(Bottleneck, self).init() self.out_planes = out_planes self.dense_depth = dense_depth self.conv1 = nn.Conv2d(last_planes, in_planes, kernel_size=1, bias=False) self.bn1 = nn.BatchNorm2d(in_planes) self.conv2 = nn.Conv2d(in_planes, in_planes, kernel_size=3, stride=stride, padding=1, groups=32, bias=False) self.bn2 = nn.BatchNorm2d(in_planes) self.conv3 = nn.Conv2d(in_planes, out_planes+dense_depth, kernel_size=1, bias=False) self.bn3 = nn.BatchNorm2d(out_planes+dense_depth) self.shortcut = nn.Sequential() if first_layer: self.shortcut = nn.Sequential( nn.Conv2d(last_planes, out_planes+dense_depth, kernel_size=1, stride=stride, bias=False), nn.BatchNorm2d(out_planes+dense_depth) ) def forward(self, x): out = F.relu(self.bn1(self.conv1(x))) out = F.relu(self.bn2(self.conv2(out))) out = self.bn3(self.conv3(out)) x = self.shortcut(x) d = self.out_planes out = torch.cat([x[:,:d,:,:]+out[:,:d,:,:], x[:,d:,:,:], out[:,d:,:,:]], 1) out = F.relu(out) return out class DPN(nn.Module): def init(self, cfg): super(DPN, self).init() in_planes, out_planes = cfg['in_planes'], cfg['out_planes'] num_blocks, dense_depth = cfg['num_blocks'], cfg['dense_depth'] self.conv1 = nn.Conv2d(3, 64, kernel_size=3, stride=1, padding=1, bias=False) self.bn1 = nn.BatchNorm2d(64) self.last_planes = 64 self.layer1 = self._make_layer(in_planes[0], out_planes[0], num_blocks[0], dense_depth[0], stride=1) self.layer2 = self._make_layer(in_planes[1], out_planes[1], num_blocks[1], dense_depth[1], stride=2) self.layer3 = self._make_layer(in_planes[2], out_planes[2], num_blocks[2], dense_depth[2], stride=2) self.layer4 = self._make_layer(in_planes[3], out_planes[3], num_blocks[3], dense_depth[3], stride=2) self.linear = nn.Linear(out_planes[3]+(num_blocks[3]+1)dense_depth[3], 10) def _make_layer(self, in_planes, out_planes, num_blocks, dense_depth, stride): strides = [stride] + 1 layers = [] for i,stride in (strides): layers.append(Bottleneck(self.last_planes, in_planes, out_planes, dense_depth, stride, i==0)) self.last_planes = out_planes + (i+2) * dense_depth return nn.Sequential(*layers) def forward(self, x): out = F.relu(self.bn1(self.conv1(x))) out = self.layer1(out) out = self.layer2(out) out = self.layer3(out) out = self.layer4(out) out = F.avg_pool2d(out, 4) out = out.view(out.size(0), -1) out = self.linear(out) return out def DPN92(): cfg = { 'in_planes': (96,192,384,768), 'out_planes': (256,512,1024,2048), 'num_blocks': (3,4,20,3), 'dense_depth': (16,32,24,128) } return DPN(cfg)基于这个程序修改成对摄像头采集的图像检测与分类输出坐标、大小和种类
时间: 2023-06-10 15:06:30 浏览: 209
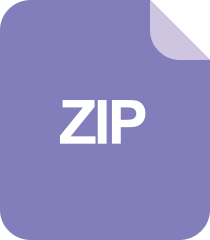
pytorch_API_docs.zip
首先,你需要将程序修改为接收摄像头采集的图像。可以使用OpenCV库来读取摄像头采集的图像。代码如下:
import cv2
cap = cv2.VideoCapture(0) # 0表示默认摄像头,如果有多个摄像头可以尝试使用1、2、3...
while True:
ret, frame = cap.read()
if not ret:
break
# 在这里加入你的图像检测与分类代码,输出坐标、大小和种类等信息
cv2.imshow('frame', frame)
if cv2.waitKey(1) & 0xFF == ord('q'):
break
cap.release()
cv2.destroyAllWindows()
在这个程序中,cap.read()用于读取摄像头的图像。如果读取成功,ret的值为True,frame为读取到的图像。接下来,你需要将读取到的图像输入到你的模型中进行检测和分类。可以使用torchvision库来完成这个任务。代码如下:
import cv2
import torch
import torchvision.transforms as transforms
cap = cv2.VideoCapture(0)
# 加载模型
model = DPN92()
model.load_state_dict(torch.load('your_model_path.pth')) # 加载预训练模型
model.eval()
# 定义图像变换
transform = transforms.Compose([
transforms.ToTensor(), # 将图像转换为Tensor
transforms.Normalize(mean=[0.485, 0.456, 0.406], std=[0.229, 0.224, 0.225]) # 归一化
])
while True:
ret, frame = cap.read()
if not ret:
break
# 对读取到的图像进行预处理
img = transform(frame)
img = img.unsqueeze(0) # 添加一维,变为[1, 3, H, W]
# 输入模型进行预测
with torch.no_grad():
output = model(img)
_, pred = torch.max(output, 1) # 获取预测结果
# 在这里加入你的图像检测与分类代码,输出坐标、大小和种类等信息
cv2.imshow('frame', frame)
if cv2.waitKey(1) & 0xFF == ord('q'):
break
cap.release()
cv2.destroyAllWindows()
在这个程序中,你需要将读取到的图像进行预处理,然后输入到你的模型中进行预测。预测结果通常是一个长度为类别数的向量,每个元素表示该图像属于该类别的概率。可以使用torch.max函数获取最大值和对应的类别,即预测结果。最后,你需要将输出的坐标、大小和种类等信息绘制到图像上,以便查看检测结果。
阅读全文
相关推荐
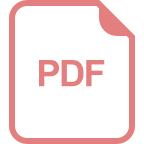
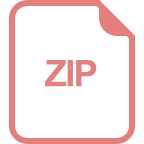










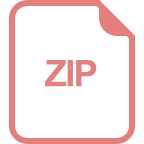
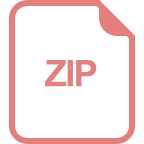
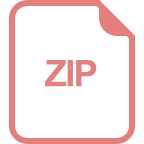
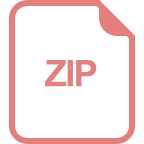
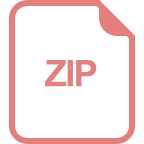
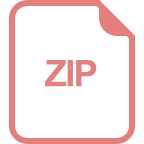