对于X,Y = make_moons(n_samples=400,shuffle=True,noise=0.1,random_state=136),使用AGNES聚类算法中cluster = AgglomerativeClustering(n_clusters=2, distance_threshold=None, linkage='single').fit(X)怎么调参最优,写出代码
时间: 2023-10-10 22:11:38 浏览: 76
AGNES聚类算法中有两个重要的参数需要调整:n_clusters和linkage。
其中,n_clusters表示聚类的簇数,linkage表示聚类时使用的距离度量方式。
下面是使用网格搜索(GridSearchCV)来寻找最优参数的代码:
``` python
from sklearn.datasets import make_moons
from sklearn.cluster import AgglomerativeClustering
from sklearn.model_selection import GridSearchCV
# 生成数据
X, Y = make_moons(n_samples=400, shuffle=True, noise=0.1, random_state=136)
# 定义模型
model = AgglomerativeClustering()
# 定义参数搜索范围
param_grid = {
"n_clusters": [2, 3, 4, 5, 6],
"linkage": ["ward", "complete", "average", "single"]
}
# 定义网格搜索
grid_search = GridSearchCV(
model, param_grid, cv=5, scoring="adjusted_rand_score"
)
# 进行网格搜索
grid_search.fit(X)
print("最优参数:", grid_search.best_params_)
print("最优得分:", grid_search.best_score_)
```
在上述代码中,我们使用了网格搜索来寻找最优参数。其中,param_grid指定了搜索的参数范围,cv=5指定了使用5折交叉验证来评估每个参数组合的性能,scoring="adjusted_rand_score"指定了评估指标为调整兰德指数。
最后,我们输出了最优参数和最优得分。
相关推荐
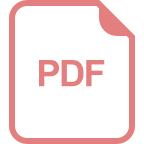
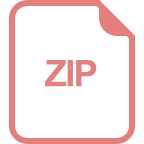








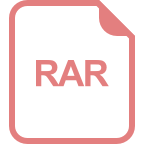
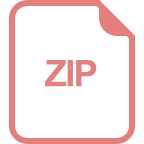
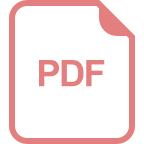
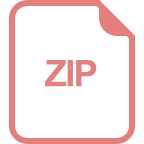