tensorrt加速onnx
时间: 2023-08-23 17:04:39 浏览: 54
TensorRT是NVIDIA深度学习推理库,可以用于加速神经网络模型的推理,包括 ONNX 模型。下面是使用 TensorRT 加速 ONNX 模型的一些步骤:
1. 安装 TensorRT:可以从 NVIDIA 官网下载并安装 TensorRT 的相应版本。
2. 将 ONNX 模型转换为 TensorRT 引擎:使用 TensorRT 的 ONNX Parser 将 ONNX 模型转换为 TensorRT 引擎。这可以通过以下代码实现:
```python
import tensorrt as trt
import onnx
# Load the ONNX model as a graph and prepare the TensorRT inference engine
onnx_model = onnx.load('model.onnx')
onnx.checker.check_model(onnx_model)
trt_engine = trt.utils.\
onnx_to_trt_engine(onnx_model,
max_batch_size=1,
max_workspace_size=1 << 28,
precision_mode="FP16",
minimum_segment_size=2)
```
3. 创建 TensorRT 推理引擎:创建 TensorRT 推理引擎实例,并为其分配输入和输出张量的内存。这可以通过以下代码实现:
```python
# Create a TensorRT inference engine
trt_logger = trt.Logger(trt.Logger.WARNING)
trt_runtime = trt.Runtime(trt_logger)
trt_context = trt_engine.create_execution_context()
# Allocate memory for inputs and outputs
input_shape = trt_engine.get_binding_shape(0)
output_shape = trt_engine.get_binding_shape(1)
input_size = trt.volume(input_shape) * trt_engine.max_batch_size * np.dtype(np.float32).itemsize
output_size = trt.volume(output_shape) * trt_engine.max_batch_size * np.dtype(np.float32).itemsize
# Allocate device memory
d_input = cuda.mem_alloc(input_size)
d_output = cuda.mem_alloc(output_size)
```
4. 执行推理:将输入数据复制到设备内存,执行推理,然后将输出数据从设备内存复制回主机内存。这可以通过以下代码实现:
```python
# Copy input data to device memory
cuda.memcpy_htod(d_input, input_data)
# Execute the inference
trt_context.execute_v2(bindings=[int(d_input), int(d_output)])
# Copy output data from device memory
output_data = np.empty(output_shape, dtype=np.float32)
cuda.memcpy_dtoh(output_data, d_output)
```
这些步骤可以帮助你使用 TensorRT 加速 ONNX 模型的推理。
相关推荐
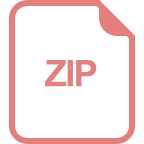
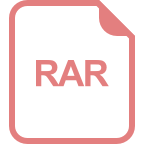
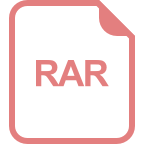
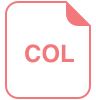
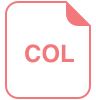
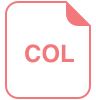
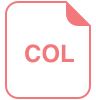
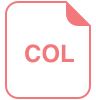









