Abstract— Image nonlocal self-similarity (NSS) property has been widely exploited via various sparsity models such as joint sparsity (JS) and group sparse coding (GSC). However, the existing NSS-based sparsity models are either too restrictive, e.g., JS enforces the sparse codes to share the same support, or too general, e.g., GSC imposes only plain sparsity on the group coefficients, which limit their effectiveness for modeling real images. In this paper, we propose a novel NSS-based sparsity model, namely, low-rank regularized group sparse coding (LR-GSC), to bridge the gap between the popular GSC and JS. The proposed LR-GSC model simultaneously exploits the sparsity and low-rankness of the dictionary-domain coefficients for each group of similar patches. An alternating minimization with an adaptive adjusted parameter strategy is developed to solve the proposed optimization problem for different image restoration tasks, including image denoising, image deblocking, image inpainting, and image compressive sensing. Extensive experimental results demonstrate that the proposed LR-GSC algorithm outperforms many popular or state-of-the-art methods in terms of objective and perceptual metrics.翻译
时间: 2024-04-28 22:25:41 浏览: 113
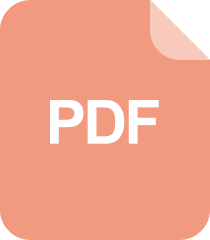
Blind Deconvolution With Nonlocal Similarity and l(0) Sparsity for Noisy Image
摘要—图像的非局部自相似性(NSS)属性已经被广泛应用于各种稀疏模型中,例如联合稀疏(JS)和群组稀疏编码(GSC)。然而,现有的基于NSS的稀疏模型要么太过严格,例如JS强制使稀疏编码共享相同的支持,要么太过通用,例如GSC仅对群组系数施加简单的稀疏性,这限制了它们对实际图像建模的有效性。本文提出了一种新的NSS-based稀疏模型,即低秩正则化群组稀疏编码(LR-GSC),以弥合流行的GSC和JS之间的差距。所提出的LR-GSC模型同时利用了每组相似补丁的字典域系数的稀疏性和低秩性。开发了一种带有自适应调整参数策略的交替最小化方法,用于解决不同的图像恢复任务,包括图像去噪、图像去块、图像修复和图像压缩感知。广泛的实验结果表明,所提出的LR-GSC算法在客观和感知度量方面优于许多流行或最先进的方法。
阅读全文
相关推荐
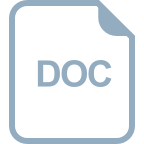
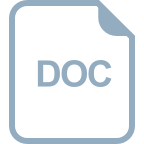
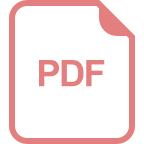
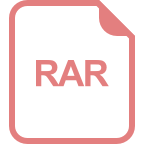
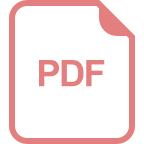
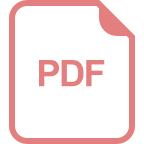
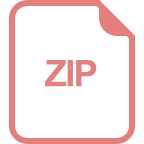
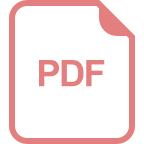
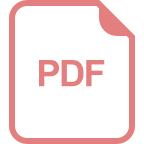
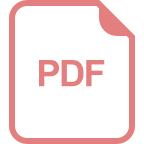
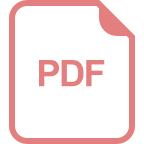
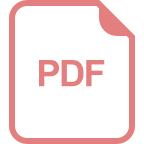
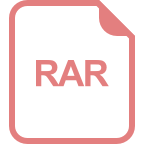
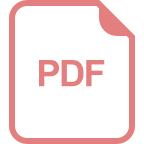
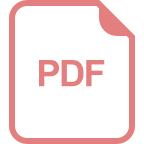
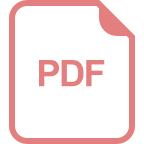
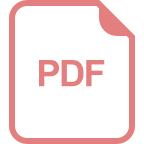
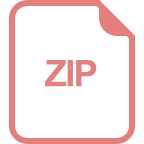