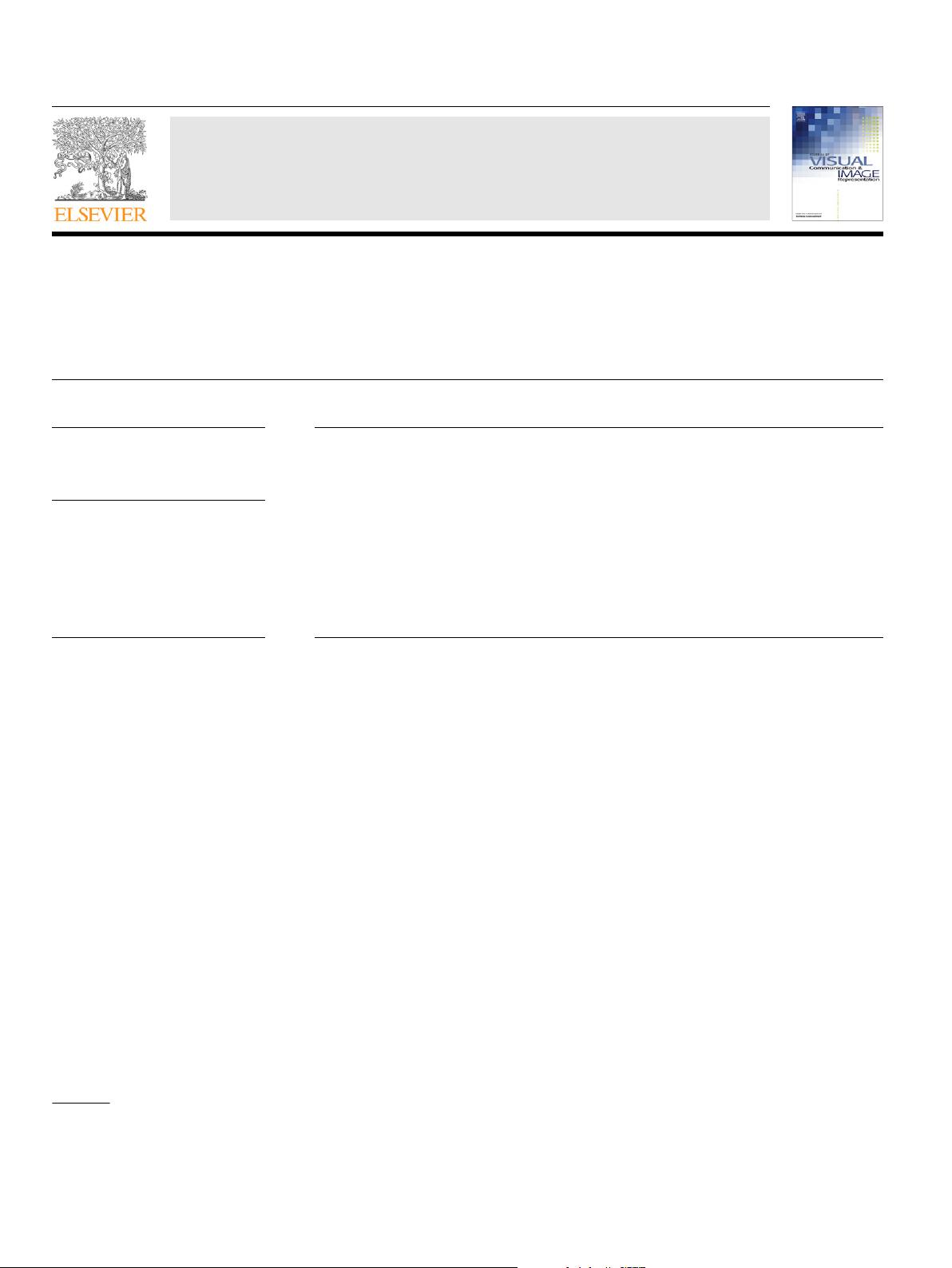
Non-local spatial redundancy reduction for bottom-up saliency estimation
q
Jinjian Wu, Fei Qi, Guangming Shi
⇑
, Yongheng Lu
School of Electronic Engineering, Xidian University, Xi’an, Shaanxi 710071, PR China
article info
Article history:
Received 28 January 2012
Accepted 23 July 2012
Available online 2 August 2012
Keywords:
Redundancy reduction
Image structure
Self-similarity
Bottom-up visual saliency
Visual attention
Non-local
Entropy
Human visual system
abstract
In this paper we present a redundancy reduction based approach for computational bottom-up visual sal-
iency estimation. In contrast to conventional methods, our approach determines the saliency by filtering
out redundant contents instead of measuring their significance. To analyze the redundancy of self-repeat-
ing spatial structures, we propose a non-local self-similarity based procedure. The result redundancy
coefficient is used to compensate the Shannon entropy, which is based on statistics of pixel intensities,
to generate the bottom-up saliency map of the visual input. Experimental results on three publicly avail-
able databases demonstrate that the proposed model is highly consistent with the subjective visual
attention.
Ó 2012 Elsevier Inc. All rights reserved.
1. Introduction
The human visual system (HVS) has a remarkable ability to ana-
lyze complex visual inputs in real-time, which locates regions of
interest very quickly [1]. Finding interesting objects is a critical
task in many image and video applications, such as region-of-inter-
est based image compression [2], object recognition [3], image
retrieval [4], image composition from sketch [5], advertisement
design [6], image and video content adaptation [7], and quality
evaluation [8,9]. Researchers attempt to build computational
models imitating the ability of the HVS to improve vision related
intelligent systems.
The rapid process during which the HVS scans the whole scene
and guides eyes to focus on the most informative areas is called vi-
sual attention [1]. There exist two distinct mechanisms governing
this procedure [10,11], which are the bottom-up stimulus driven
and the top-down goal driven mechanisms, respectively. The two
mechanisms jointly determine the distribution of attention [12].
Bottom-up saliency estimation is the first step for image under-
standing and analysis, which is involuntarily response of environ-
mental stimulus [1,13]. In this paper, rather than building a
saliency model including both the bottom-up and top-down mech-
anisms, we provide a model for pure bottom-up visual saliency
estimation from the perspective of redundancy reduction.
1.1. Related works
Current research on the computational visual attention tries to
model bottom-up and top-down mechanisms. The bottom-up
based computational model imitates the function of the preatten-
tion, which is involuntary and pure data-driven, to generate a
saliency map showing the conspicuousness of each position. The
top-down mechanism determines the final response of the HVS
[14] and directs eye fixation [15] according to voluntary affections.
The existing top-down based computational models focus mainly
on assessing contributions of each feature to fuse outputs of
bottom-up based computational models [16–19].
Researches in neuropsychology show that the bottom-up sal-
iency of a given location is determined by how distinct it is from
its surroundings [10,20,21]. Furthermore, the bottom-up attention
is driven by visual features of images, such as color [22], contrast in
luminance [23,24], sizes of objects [25], distributional statistics
[26], contrast in histogram [27], and discriminant analysis
[28,29]. Based on these results, many computational models have
been proposed to estimate the bottom-up visual saliency
[1,10,13,30–33]. In summary, bottom-up saliency is estimated
with following steps: (a) select a set of adequate features, (b) eval-
uate the distinction over each feature, and (c) fuse all channels of
distinctions into the final saliency map.
Most existing models try to select some ‘‘good’’ features, on
which objects are the most distinct against surroundings, for sal-
iency estimation. In [34], Privitera and Stark evaluated the perfor-
mances of ten contrast based models on a single feature by
comparing the generated saliency map with the eye fixation
1047-3203/$ - see front matter Ó 2012 Elsevier Inc. All rights reserved.
http://dx.doi.org/10.1016/j.jvcir.2012.07.010
q
This work is supported in part by National Natural Science Foundation of China
under Grant Nos. 60805012, 61033004, 61070138, and 61100155.
⇑
Corresponding author.
E-mail address: gmshi@xidian.edu.cn (G. Shi).
J. Vis. Commun. Image R. 23 (2012) 1158–1166
Contents lists available at SciVerse ScienceDirect
J. Vis. Commun. Image R.
journal homepage: www.elsevier.com/locate/jvci