quant, diff, (_,_,ind) = self.encode(input)详细解析
时间: 2023-09-24 21:02:49 浏览: 152
这行代码是将self.encode(input)函数的返回值解包(unpack)到quant、diff和(_,_,ind)三个变量中。
在该模型中,self.encode(input)是编码器的一次前向传递,输入input经过编码器处理后,得到量化表示quant、量化误差diff和编码器输出的中间状态(_,_,ind)。其中,_表示占位符,表示该变量不会被使用,只是为了占位。ind是预测的索引。
在该行代码中,我们使用了解包操作,将self.encode(input)的返回值按顺序赋值给quant、diff和(_,_,ind)三个变量。这意味着,我们可以直接使用quant、diff和ind变量来引用编码器的输出,而不需要通过self.encode(input)来访问。
需要注意的是,这行代码前提是self.encode(input)的返回值是一个元组(tuple)或者列表(list),并且元素数量必须和左边的变量数量相等,否则会报错。
相关问题
super().__init__() self.embed_dim = embed_dim self.n_embed = n_embed self.image_key = image_key self.encoder = Encoder(**ddconfig) self.decoder = Decoder(**ddconfig) self.loss = instantiate_from_config(lossconfig) self.quantize = VectorQuantizer(n_embed, embed_dim, beta=0.25, remap=remap, sane_index_shape=sane_index_shape) self.quant_conv = torch.nn.Conv2d(ddconfig["z_channels"], embed_dim, 1) self.post_quant_conv = torch.nn.Conv2d(embed_dim, ddconfig["z_channels"], 1)解析
这是一个Python类的初始化方法,其中包含了如下代码:
- `super().__init__()`:调用父类的初始化方法。
- `self.embed_dim = embed_dim`:将传入的`embed_dim`参数赋值给类的实例变量`embed_dim`。
- `self.n_embed = n_embed`:将传入的`n_embed`参数赋值给类的实例变量`n_embed`。
- `self.image_key = image_key`:将传入的`image_key`参数赋值给类的实例变量`image_key`。
- `self.encoder = Encoder(**ddconfig)`:实例化一个`Encoder`类的对象,并将`ddconfig`参数解包后传入。
- `self.decoder = Decoder(**ddconfig)`:实例化一个`Decoder`类的对象,并将`ddconfig`参数解包后传入。
- `self.loss = instantiate_from_config(lossconfig)`:通过`instantiate_from_config()`函数实例化一个损失函数对象,并将`lossconfig`参数传入。
- `self.quantize = VectorQuantizer(n_embed, embed_dim, beta=0.25, remap=remap, sane_index_shape=sane_index_shape)`:实例化一个`VectorQuantizer`类的对象,其中`n_embed`和`embed_dim`分别为向量量化器的嵌入向量数量和维度,`beta`为损失函数中的权重因子,`remap`为需要重映射的键名和新的键名,`sane_index_shape`表示向量量化器是否需要返回索引的形状。
- `self.quant_conv = torch.nn.Conv2d(ddconfig["z_channels"], embed_dim, 1)`:实例化一个`Conv2d`类的对象,用于将潜空间编码为嵌入向量。
- `self.post_quant_conv = torch.nn.Conv2d(embed_dim, ddconfig["z_channels"], 1)`:实例化一个`Conv2d`类的对象,用于将嵌入向量解码为潜空间。
# -*- coding: utf-8 -*- """ Created on Fri Mar 5 19:13:21 2021 @author: LXM """ import torch import torch.nn as nn from torch.autograd import Function class UpdateRange(nn.Module): def __init__(self, device): super(UpdateRange, self).__init__() self.device = device self.flag = 0 self.fmin = torch.zeros((1), dtype = torch.float32, device = self.device) self.fmax = torch.zeros((1), dtype = torch.float32, device = self.device) def Update(self, fmin, fmax): if self.flag == 0: self.flag = 1 new_fmin = fmin new_fmax = fmax else: new_fmin = torch.min(fmin, self.fmin) new_fmax = torch.max(fmax, self.fmax) self.fmin.copy_(new_fmin) self.fmax.copy_(new_fmax) @torch.no_grad() def forward(self, input): fmin = torch.min(input) fmax = torch.max(input) self.Update(fmin, fmax) class Round(Function): @staticmethod def forward(self, input): # output = torch.round(input) # output = torch.floor(input) output = input.int().float() return output @staticmethod def backward(self, output): input = output.clone() return input class Quantizer(nn.Module): def __init__(self, bits, device): super(Quantizer, self).__init__() self.bits = bits self.scale = 1 self.UpdateRange = UpdateRange(device) self.qmin = torch.tensor((-((1 << (bits - 1)) - 1)), device = device) self.qmax = torch.tensor((+((1 << (bits - 1)) - 1)), device = device) def round(self, input): output = Round.apply(input) return output def Quantization(self): quant_range = float(1 << (self.bits - 1)) float_range = torch.max(torch.abs(self.UpdateRange.fmin), torch.abs(self.UpdateRange.fmax)) scale = 1 for i in range(32): if torch.round(float_range * (1 << i)) < quant_range: scale = 1 << i else: break self.scale = scale def forward(self, input): if self.training: self.UpdateRange(input) self.Quantization() output = (torch.clamp(self.round(input * self.scale), self.qmin, self.qmax)) / self.scale return output
这段代码是一个用于量化神经网络参数的模块。它定义了三个类:UpdateRange、Round和Quantizer。
UpdateRange类用于更新输入数据的范围,它记录了输入数据的最小值和最大值,并在每个前向传播过程中更新这些值。
Round类是一个自定义的torch.autograd.Function,用于将输入数据四舍五入为整数。
Quantizer类是一个量化器模块,它接收一个bits参数,用于指定量化的位数。在前向传播过程中,它首先使用UpdateRange类更新输入数据的范围,然后根据量化范围和位数计算量化的比例因子scale。最后,它将输入数据乘以scale进行量化,并使用clamp函数将量化结果限制在指定的最小值和最大值范围内。
这段代码看起来是一个基于PyTorch框架实现的量化模块,用于将浮点数参数量化为固定位数的整数,以减少存储空间和计算资源的使用。
阅读全文
相关推荐
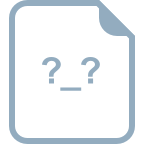
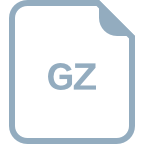
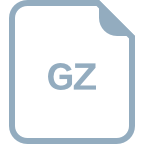


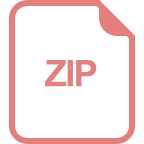


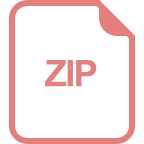
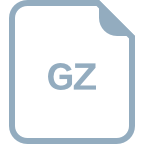
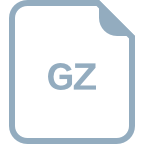
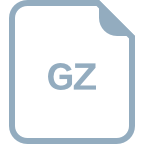
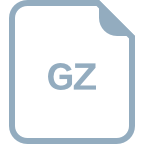
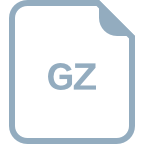
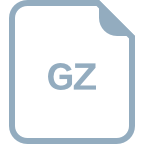


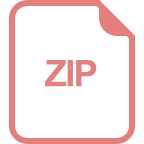